Introduction
The latest mannequin assortment from Microsoft’s innovative household brand, Phi-3. They excel over their peers of similar and larger scales across various metrics in language understanding, logical reasoning, programming, and mathematical proficiency. These products are designed to be exceptionally high-performing and cost-efficient. With the availability of Phi-3 fashions in Azure, customers gain access to a broader spectrum of innovative styles, providing them with more effective options for developing and refining generative AI applications. As Azure prepares for its April 2024 launch, customer feedback and input from the community have identified key areas where Phi-3 SLMs can be refined.
The Phi family is delighted to welcome three new members: 1, 2, and 3, a unique Combination-of-Experts (CoE) model, further expanding their esteemed community. The Phi-3.5-mini offers an expansive 128K context size, significantly enhancing its multilingual support capabilities for users. Philosophical insights into three-dimensional visual perception enhance comprehension and facilitate logical reasoning when processing complex image sequences, thereby optimizing performance on traditional single-image evaluation metrics. The Phi-3.5-MoE solution stands out from larger trends while maintaining the effectiveness of previous Phi offerings through a team of 16 consultants, featuring 6.6 billion energetic parameters, ultra-low latency, multilingual support, and robust security capabilities.
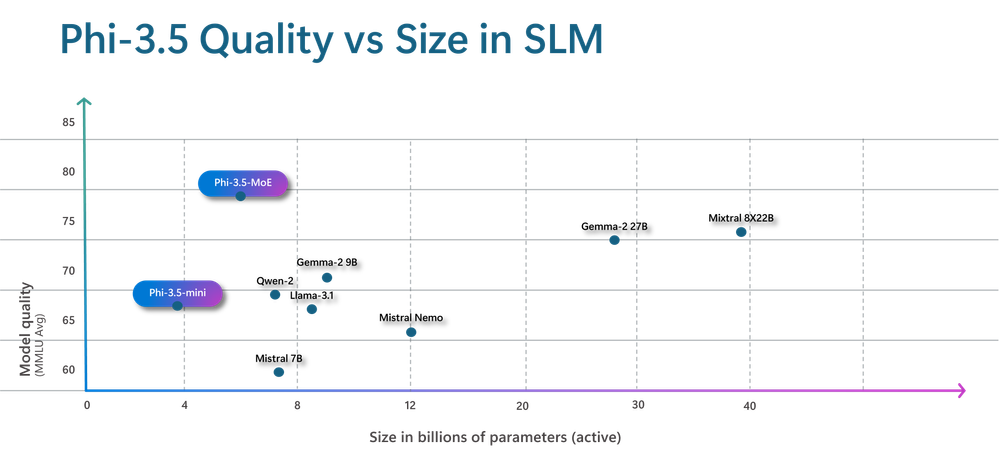
Phi-3.5-MoE: Combination-of-Consultants
The latest addition to the Phi 3.5 Series, Phi-3.5-MoE, stands out as a pioneering innovation in Soft Lithium Metal (SLM) technology. The system houses a substantial team of 16 consultants, each entrusted with an enormous volume of approximately 3.8 billion parameters. The revised text is: With a comprehensive mannequin size of 42 billion parameters, it generates 6.6 billion parameters using two consultants. The modular educational (MoE) mannequin outperforms its dense counterpart of similar size in terms of both quality and efficiency. More than 20 languages are supported across our platform. The model leverages a blend of both proprietary and open-source artificial intelligence and decision-making datasets, utilizing its robust post-training security protocol to ensure unparalleled efficacy. By leveraging both artificial and human-annotated datasets, our post-processing approach harmoniously integrates Direct Desire Optimization (DPO) with Supervised Fine-Tuning (SFT). These collections consist of various security classes and datasets that prioritize innocuousness and benevolence. Moreover, Phi-3.5-MoE enables processing of massive contexts up to 128 kilobytes in size, effectively tackling complex workload demands.
Additionally learn:
The coaching information for Phi 3.5 MoE is as follows:
Comprising a vast array of sources, the coaching knowledge contained within Phi 3.5’s Massive Open Ephemeer (MoE) totals an impressive 4.9 trillion tokens, inclusive of 10% multilingual content, and serves as a rich tapestry of information, blending together diverse forms of knowledge and perspectives to provide a comprehensive foundation for exploration and discovery.
- Publicly accessible documentation, thoroughly vetted for exceptional quality, featuring premium instructional content and code.
- Here is the rewritten text:
Artificial intelligence has given rise to a new form of knowledge that can be likened to a textbook, providing clear explanations of mathematical concepts, coding languages, logical reasoning, and everyday facts about science, human behavior, and the world at large.
- Supervised conversational models safeguard high-quality dialogue by replicating human-like traits such as instructional guidance, authenticity, integrity, and assistance, thereby fostering trust and reliability.
Emphasizes the optimization of knowledge standards that would likely elevate the mannequin’s cognitive abilities, subsequently refining the filtration process to incorporate the optimal level of relevant information from publicly available documents. The nuances of a Premier League match may seem tangential to frontier fashion’s design constraints, but extracting this data could liberate model capabilities for smaller-scale applications. The extra particulars about knowledge that underlie the Phi-3 Technical Report await discovery by those who delve into its technical details.
Coaching sessions utilizing Phi 3.5 MoE logic require a duration of approximately 23 days to execute, concurrently necessitating the utilization of 4.9 trillion tokens of coaching expertise. The platform supports a diverse range of languages, including Arabic, Chinese (Simplified and Traditional), Czech, Danish, Dutch, English, Finnish, French, German, Hebrew, Hungarian, Italian, Japanese, Korean, Norwegian, Polish, Portuguese, Russian, Spanish, Swedish, Thai, Turkish, and Ukrainian.
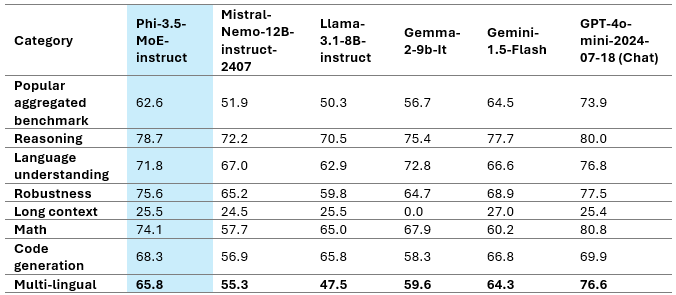
This desk exemplifies a high-fidelity Phi-3.5-MoE Mannequin with diverse capabilities. Notably, Phi 3.5 MoE surpasses several larger fashion models across diverse categories. This compact Phi-3.5-MoE model, boasting just 6.6 billion energetic parameters, surprisingly matches the linguistic comprehension and mathematical prowess of much larger architectures. Despite its inanimate nature, the mannequin surprisingly surpasses many human creations in terms of logical processing capabilities. The mannequin provides excellent flexibility for fine-tuning diverse tasks.
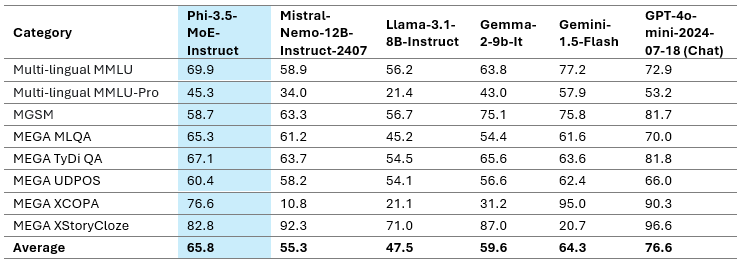
The MMLU, MEGA, and multilingual MMLU-pro datasets, which cater to diverse linguistic needs, serve as a comprehensive showcase of the Phi-3.5-MoE’s proficiency in handling multiple languages seamlessly. Our analysis reveals that the mannequin surpasses rival fashion models by a considerable margin in terms of energy performance on multilingual tasks, despite having only 6.6 billion energetic parameters.
Phi-3.5-mini
The Phi-3.5-mini mannequin was subsequently pre-trained using a rich repository of multilingual artificial intelligence and high-quality, carefully curated knowledge. Following the training procedure, various subsequent steps were implemented, including Direct Desire Optimization (DPO) and Proximal Coverage Optimization (PCO). Procedures utilized a trifecta of data sources: artificial, translated, and human-labeled datasets.
Coaching information for Phi 3.5 Mini, a premier sports coaching program, offers expert guidance to enhance players’ skills and team performance.
Phi 3.5 Mini’s comprehensive coaching package includes:
* Customized training sessions tailored to individual player needs
* Expert analysis of game footage to identify areas for improvement
* Strategies for improving teamwork, communication, and leadership
* Guidance on developing mental toughness and resilience
By leveraging Phi 3.5 Mini’s experienced coaches and cutting-edge techniques, athletes can elevate their games, build confidence, and achieve their full potential.
SKIP
Comprising an astonishing 3.4 trillion tokens, the Coaching knowledge of Phi 3.5 Mini is an unparalleled repository of information, drawing from a diverse array of sources to provide a comprehensive framework for understanding this complex subject matter.
- High-quality educational content and codes are carefully selected from publicly available documents after rigorous filtering to ensure exceptional standards.
- Here is the rewritten text in a different style:
This novel form of learning offers an innovative approach to acquiring textbook-caliber knowledge that encompasses mathematical concepts, programming skills, logical reasoning, and everyday understanding of scientific principles, societal norms, and cognitive processes.
- Supervising conversational interactions with high-quality content safeguards various topics by mimicking human preferences, such as following instructions truthfully, honestly, and providing helpful responses.
Mannequin High quality
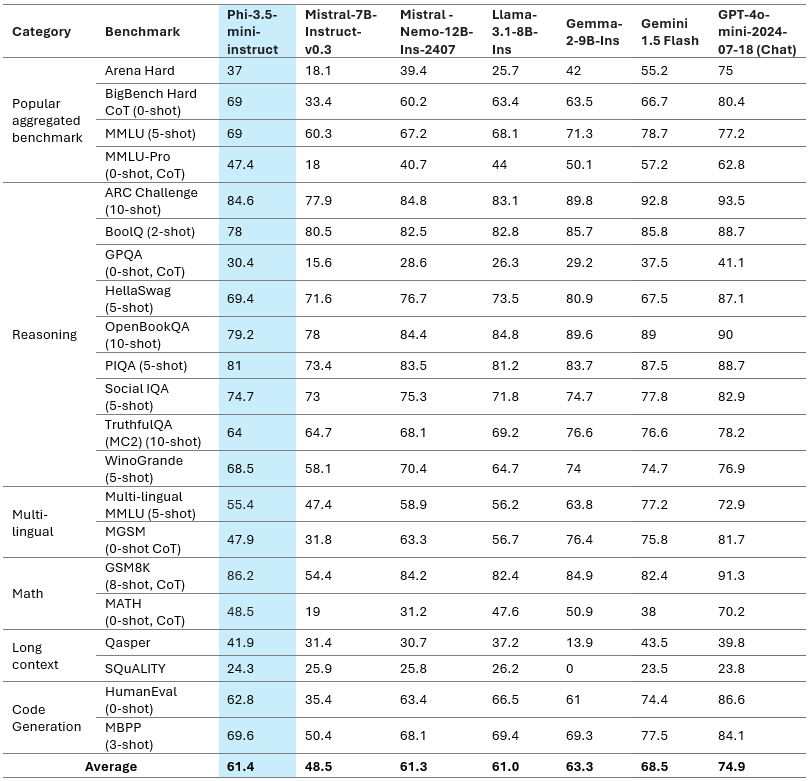
The table above offers a swift glance at the mannequin’s performance on crucial indicators. While this innovative model excels or even surpasses other styles with bigger sizes despite its remarkably compact architecture comprising just 3.8 billion parameters,
Additionally learn:
Multi-lingual Functionality
We have recently introduced our upgraded 3.8B model, now replaced by the Phi-3.5-mini. The model notably enhanced its proficiency in multilingual communication, conversational coherence, and logical reasoning through the strategic integration of additional steady pre-training and post-training knowledge sources.
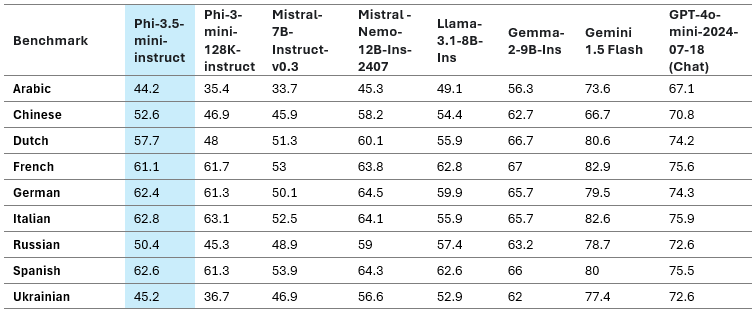
The incorporation of multilingual support represents a significant leap forward from the previous Phi-3-mini to Phi-3.5-mini models. The newly released Phi 3.5 mini reportedly achieved significant efficiency gains of 25-50%, with notable improvements for users relying on Arabic, Dutch, Finnish, Polish, Thai, and Ukrainian languages. In a comprehensive analysis, Phi-3.5-mini showcases exceptional efficiency among sub-8B language models, including English. It is essential to note that while the model has been optimized for high-resource languages and features 32K vocabulary, it is not recommended for low-resource languages without additional fine-tuning.
Lengthy Context

In a context of 128KB, the Phi-3.5-mini offers an exceptional solution for applications such as information retrieval, comprehensive document analysis, and condensing lengthy reports or meeting transcripts to ensure high-quality outputs. Compared to the Gemma-2 household, which is limited to handling an 8K content size, Phi-3.5 demonstrates superior performance. Despite its compact size, the Phi-3.5-mini faces intense competition from larger, open-weight fashion lines such as those offered by top-tier brands like 7, and . The Phi-3.5-mini-instruct model stands alone in its class, boasting a mere 3.8 billion parameters, an impressive 128K context size, and multilingual capabilities for enhanced language understanding. Azure opted to support additional languages while maintaining consistent English proficiency across diverse tasks. Given the limitations of mannequins’ capabilities, English language data may actually surpass that of other languages in certain aspects. Within the context of RAG setups, Azure recommends utilizing the mannequin for tasks necessitating an exceptionally high level of multilingual comprehension.
Additionally learn:
Phi-3.5-vision with Multi-frame Enter
The Art of Coaching: Elevating Your Game to Three-and-a-Half Vision.
Azure’s coaching expertise encompasses an extensive range of sources, seamlessly blending together.
- Publicly accessible documentation, thoroughly vetted for exceptional quality, features select top-tier educational content and code.
- Chosen high-quality image-text interleave knowledge;
-
Novel artificial intelligence-generated content aims to educate students in mathematics, programming, critical thinking, and everyday life concepts, including science, daily habits, cognitive frameworks, and more. This innovative approach includes newly created visual aids such as charts, diagrams, slides, and multimedia presentations featuring quick video clips or pairs of related images.
- Supervising high-quality conversations requires robust knowledge management systems that can effectively replicate human preferences for instructive dialogue, unwavering truthfulness, honest feedback, and practical helpfulness in diverse topics.
The information curation process involved sourcing data from publicly accessible documents and meticulously filtering out unwanted documents and images. To ensure privacy, we thoroughly screened a range of image and text data sources to remove or sanitize any potentially sensitive information from the training data.
Powered by groundbreaking consumer insights, Phi-3.5-vision boasts cutting-edge multi-frame picture comprehension and logical processing abilities. This groundbreaking technology enables precise image comparisons across various contexts, facilitates the creation of compelling multi-image narratives and stories, as well as concise video summaries.
Notably, Phi-3.5-vision has demonstrated impressive efficacy across multiple single-image benchmarks. By serving as a benchmark, this initiative successfully boosted MMBench efficiency from 80.5 to 81.9 and MMMU efficiency from 40.4 to 43.0. The usual trend in doc comprehension and TextVQA metrics saw a notable improvement, with scores rising from 70.9 to 72.0.
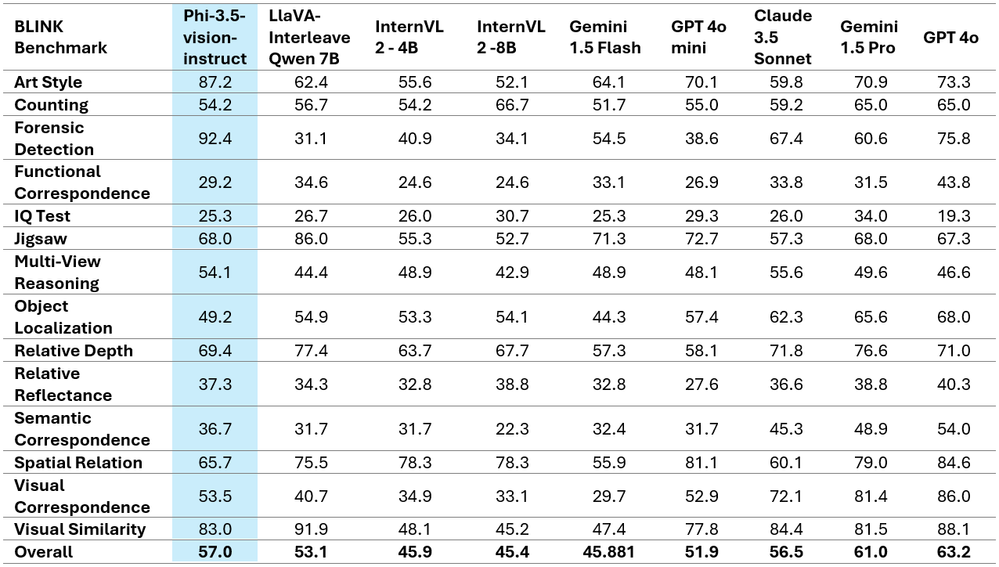
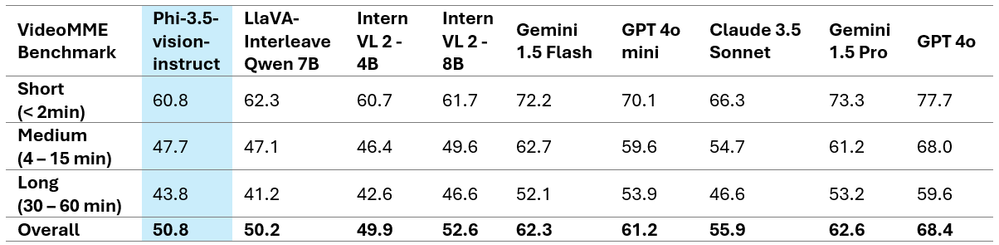
The tables presented above demonstrate a notable enhancement in efficiency metrics and highlight compelling comparative insights on two prominent multi-image/video benchmarks. While it’s crucial to acknowledge the limitations of Phi-3.5-Imaginative and prescient, it’s equally vital to recognize its inability to facilitate seamless multilingual usage scenarios. Without further refinement, it is strongly cautioned against employing this solution for multilingual contexts.
Attempting out Phi 3.5 Mini
Utilizing Hugging Face
To implement a Phi 3.5 Mini-based solution, we will leverage its capabilities to support larger models like the Phi 3.5 mini mannequin, surpassing the limitations of Google Colab. Ensure that you permit the accelerator to utilize the GPU for optimal performance.
1st Step: Importing essential libraries
torch.manual_seed(0)
Loading the mannequin and tokenizer is a crucial step in the process. The mannequin serves as a template for the model’s output, while the tokenizer breaks down input text into individual words or tokens that can be processed by the algorithm. Effective loading of these components is essential to ensure accurate predictions and consistent results.
mannequin = AutoModelForCausalLM.from_pretrained(
"microsoft/Phi-3.5-mini-instruct",
device_map="cuda",
torch_dtype="auto",
trust_remote_code=True,
)
tokenizer = AutoTokenizer.from_pretrained("microsoft/Phi-3.5-mini-instruct")
third Step: Making ready messages
messages = [
{"role": "system", "content": "I'm happy to provide information about Microsoft! Would you like to know more about their history, products, or achievements?"},
{"role": "user", "content": "Tell me about Microsoft"}
]
“Function”: The AI model’s purpose unites the conduct of the artificial intelligence system – specifically, it serves as a “useful AI assistant”.
The concept of “function”: “consumer” represents the entry point for the consumer’s input.
Step 4: Creating the Pipeline
pipe = pipeline(
"text-generation",
mannequin=mannequin,
tokenizer=tokenizer,
)
This script establishes a pipeline for generating textual content using the preferred model and tokenizer. The pipeline seamlessly abstracts away the intricacies of tokenization, model execution, and decoding, providing a straightforward interface for generating text.
Step 5: Setting Technology Arguments
generation_args = {"max_new_tokens": 500, "return_full_text": False, "temperature": 0.0, "do_sample": False}
The parameters govern how the model produces written output.
- Maximum new tokens allowed: 500
- What is the current status of the project?
- temperature=0.0: Regulates the degree of unpredictability in the output’s outcome. A price of 0.0 makes the mannequin deterministic, thereby guaranteeing a predictable outcome.
- Disables sampling, ensuring the model always chooses its most likely next token.
Step 6: Producing Textual content
output = pipe(messages, **generation_args)
print(output[0]['generated_text'])
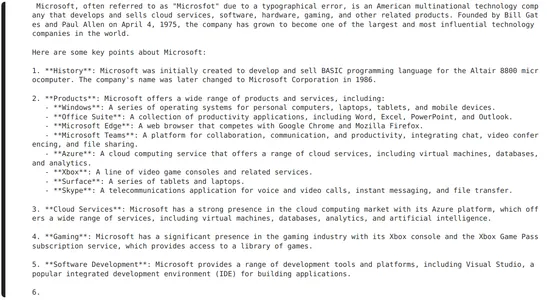
Utilizing Azure AI Studio
We’re capable of attempting a Phi 3.5 mini installation using their proprietary interface seamlessly. The “Strive It Out” feature is located within the Azure AI Studio. Utilizing the Phi 3.5 Mini: A Snapshot
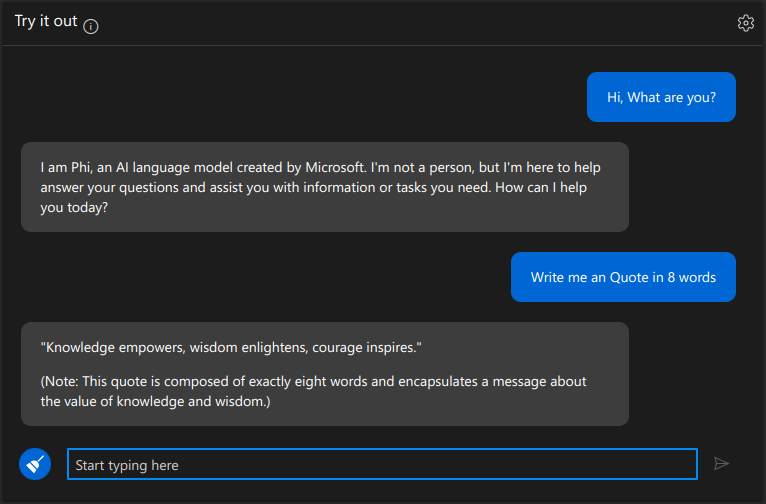
Utilizing HuggingChat from Hugging Face
The HuggingFace Conversational AI Chat.
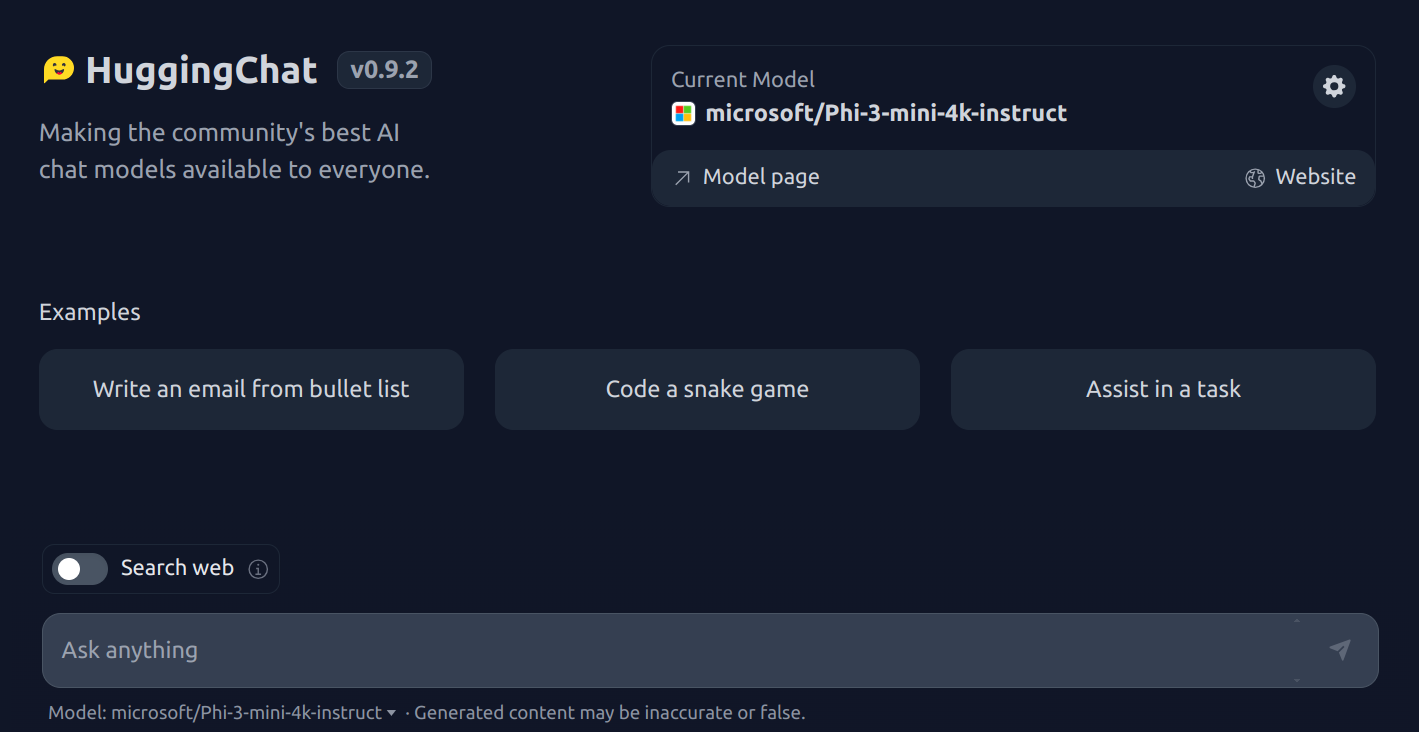
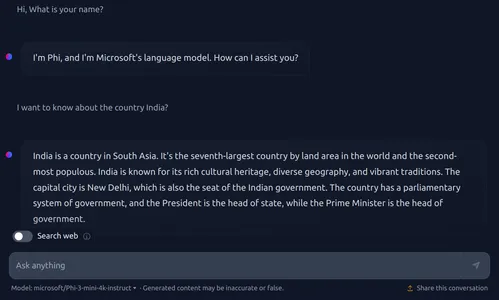
As I venture deeper into the realm of uncharted thought, I begin to grasp the intricacies of Phi 3.5’s imaginative and prescient. The harmony of disparate elements coalesces into a profound understanding that transcends the boundaries of mere mortal comprehension.
Utilizing Areas from Hugging Face
As Phi 3.5 Imaginative and prescient requires significant GPU resources, it’s incompatible with free tiers on Colab and Kaggle, necessitating an upgraded environment or alternative platforms. So therefore, I have attempted to apply philosophical principles to a futuristic scenario of imagination and foresight.
We are expected to utilize the accompanying image.
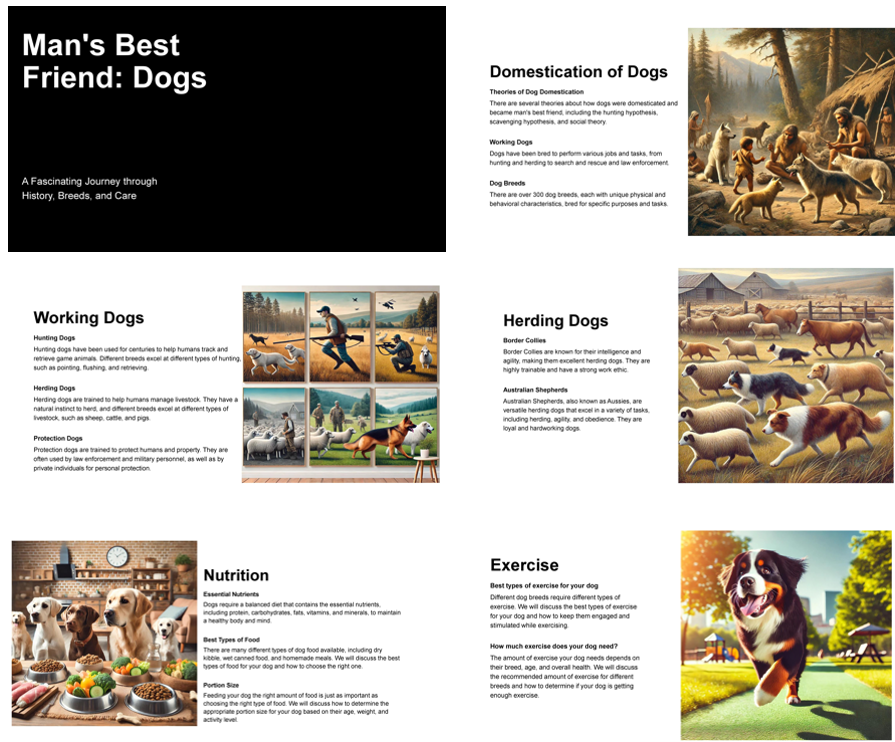
Output
The historical past and care of canine species are the subject of this presentation. The evolution of canine companions: A comprehensive exploration of dog domestication, occupations, herd management prowess, nutritional requirements, and training capabilities? A visual showcase features canines engaged in diverse activities, including herding sheep, collaborating with humans, and exercising amidst urban backdrops.
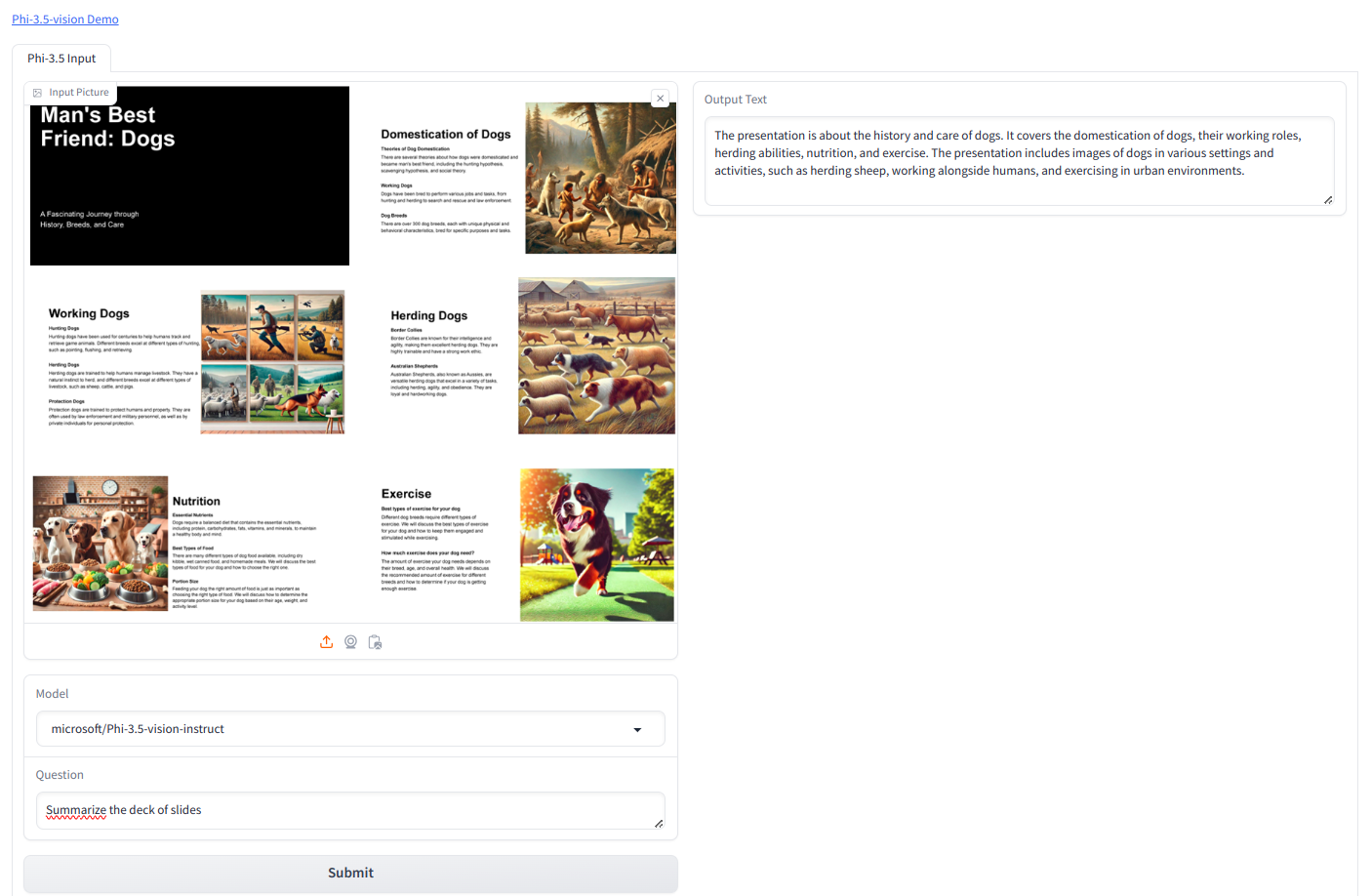
Conclusion
The Phi-3.5-mini is a groundbreaking AI model boasting an impressive 3.8 billion parameters, 128 kilobytes of contextual processing capacity, and multilingual support for seamless assistance across languages. It effectively reconciles expansive linguistic assistance with English knowledge compression. Utilized most effectively in setups requiring multilingual capabilities. The Phi-3.5-MoE boasts an impressive team of 16 small consultants, harnessing their expertise to deliver exceptional efficiency while minimizing latency. This innovative solution also accommodates a substantial 128k context size, supporting a diverse range of languages. With its versatility allowing customization for diverse applications and boasting an impressive 6.6 billion energetic parameters, this remarkable entity truly stands out. The enhancement of single-image benchmark efficiency improves significantly. The Phi 3.5 single-lens module (SLM) provides cost-effective, high-capability options for both the open-source community and Azure clients.
Continuously Requested Questions
Ans. Microsoft’s latest innovation is the Phi-3.5 fashion, a game-changer within the Small Language Models (SLMs) family, engineered to deliver unparalleled efficiency and effectiveness in processing complex tasks such as natural language, logical reasoning, programming, and mathematical calculations.
Ans. The Phi-3.5-MoE model is a unique combination of consultant-based architecture featuring 16 consultants that support over 20 languages, with a context size of 128K, specifically designed to surpass larger models in reasoning and multilingual tasks.
Ans. The Phi-3.5-Mini boasts an impressive compact design, packing 3.8 billion parameters into its diminutive form, alongside a generous 128K context size, and further enhances its linguistic capabilities with advanced multilingual support. The language proficiency program excels in multiple languages, including English.
Ans. You can attempt to deploy Phi-3.5 SLMs on platforms such as Hugging Face and Azure AI Studio, where they are readily available for diverse AI applications.