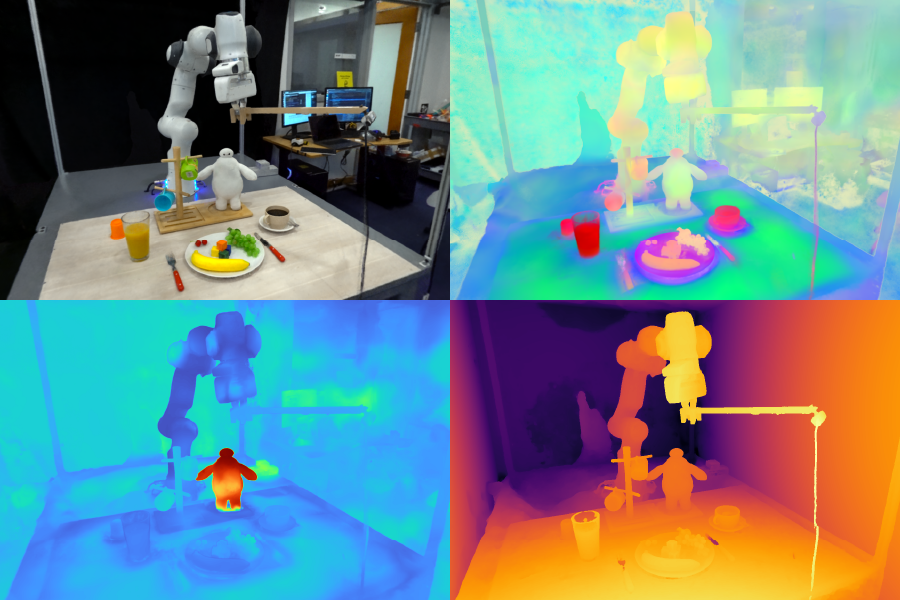
The Function Fields for Robotic Manipulation (F3RM) framework empowers robots to comprehend and respond to open-ended, text-based input, enabling them to adaptively grasp and manipulate previously unseen objects through natural language commands. In complex settings featuring numerous objects, such as large-scale warehouses, the system’s ability to generate three-dimensional characteristic fields may prove particularly valuable.
What’s the first thing that catches your eye when you open your friend’s fridge? The array of gadgets appears foreign at first, their unfamiliar packaging and containers a puzzle to decipher. Despite initial differences, you begin to comprehend the purposes behind each and arrange them according to your preferences.
Researchers at MIT’s Computer Science and Artificial Intelligence Laboratory (CSAIL) have developed Function Fields for Robotic Manipulation, a groundbreaking system that seamlessly integrates 2D images with basic model options into 3D scenes to enable robots to accurately recognize and grasp nearby objects, showcasing humans’ remarkable ability to adapt to unfamiliar situations. Can accurately interpret open-ended language prompts to facilitate seamless interactions within complex real-world environments, including vast warehouses and households featuring hundreds of distinct objects.
F3RM empowers robots with the ability to understand and respond to open-ended, nuanced textual prompts, enabling them to accurately interact with and manipulate physical objects. As a result, machines are capable of understanding more general commands from humans and still fulfill their intended purpose. Upon receipt of a request such as “pick out a tall mug”, our robotic system can swiftly locate and retrieve the product that best matches that criteria.
“Creating robots capable of genuine generalization in real-world scenarios is an incredibly arduous task,” remarks a postdoctoral researcher at the National Science Foundation’s AI Institute for Artificial Intelligence and Basic Interactions and MIT CSAIL. To successfully execute this mission, we must first develop a plan to generalize our findings from just a few objects to a comprehensive dataset discovered within MIT’s renowned Stata Center. We aimed to develop robots capable of versatility similar to our own, as we effortlessly manipulate and position objects without prior knowledge or experience.
Studying what’s there by trying?
The tactic could potentially assist robots in selecting equipment in large-scale achievement centers, navigating the complexities and uncertainties that often arise. Robots in these warehouses are occasionally provided with a detailed outline of the inventory they need to organize. To ensure accurate shipping, robots must effectively match textual descriptions of products despite differing packaging configurations, guaranteeing client orders are fulfilled correctly.
The achievement capabilities of main online retailers encompass vast catalogues, boasting tens of thousands of items, many of which an AI may not have previously encountered. To operate effectively on this massive scale, robots must comprehend the intricate geometries and semantic meanings of numerous devices, including those situated in confined spaces. As F3RM’s exceptional spatial and semantic comprehension abilities develop, robots may become increasingly adept at locating objects, placing them in designated containers, and then dispatching them for efficient packaging. Ultimately, this innovation may enable manufacturing unit personnel to fulfill customer orders more efficiently.
“One surprising aspect of the FERM system, according to Yang, is its scalability: it not only applies to individual factors but also effectively translates to room and building scales, enabling the creation of simulation environments for robotics learning and large-scale mapping.” Before we consider scaling up this effort, we need to ensure that the current approach is efficient and effective. We’ll utilize this approach to empower the execution of complex robotic management tasks in real-time, enabling robots handling sophisticated duties to leverage its benefits.
MIT staff observes that F3RM’s capacity to comprehend diverse scenarios could prove valuable in both urban and familial settings. The proposed approach could facilitate the development of personalized robots to identify and manipulate specific objects. The system assists robots in adapting to their environment – both physically and perceptually.
According to senior creator and MIT affiliate professor of electrical engineering and computer science, along with being a principal investigator at CSAIL, David Marr’s concept of visible notion revolves around the challenge of grasping “what’s the place by trying,” they explain. The latest basis fashions have demonstrated significant improvement in their ability to recognize objects, capable of identifying hundreds of object classes and providing detailed textual descriptions of images. Meanwhile, radiance fields have become increasingly adept at rendering the spatial layout of objects within a scene. The combination of these two methods produces a vivid depiction of a three-dimensional space, showcasing its potential benefits in robotic applications where precise object manipulation is crucial.
Making a “digital twin”
As F3RM starts to comprehend its surroundings, it captures moments with a selfie stick, snapping photographs that help it better understand the world around it. The mounted digital camera captures 50 diverse photographs, allowing it to generate a NeRF (Neural Radiance Field), a cutting-edge deep learning method that transforms 2D images into a lifelike 3D scene. This collage of RGB photographs generates a virtual replica of its surroundings in the form of a comprehensive, 360-degree visual representation of nearby environments.
Alongside an exceptionally refined neural radiance discipline, F3RM develops a characteristic discipline to elevate geometry by integrating semantic data seamlessly. The system leverages an imaginative and visionary foundation model trained on hundreds of millions of images, enabling it to learn visual concepts with remarkable effectiveness. Through innovative reconstruction of two-dimensional computer-generated imagery (CLIP) options from selfies captured with a selfie stick, F3RM seamlessly transforms these 2D options into immersive three-dimensional illustrations.
Retaining issues open-ended
Following mere exposure to demonstrations, the robot seamlessly applies its grasp of geometry and semantics to comprehend novel objects it had previously never encountered. When someone asks a text-based question, the AI system rapidly scans its vast database of relevant information to identify the most pertinent answers and present them to the user in a concise and accurate manner. Potential possibilities are scored according to their relevance, similarity to the robotic’s training demonstrations, and whether they would result in collisions. The highest-scoring solution is subsequently selected and implemented.
Researchers tested the system’s capacity for open-ended requests by prompting the robot to grasp Baymax, a Disney character from “Big Hero 6.” Although F3RM had not been directly trained on this specific toy, it employed its spatial awareness and vision-language capabilities from pre-trained models to identify and pick up the object.
F3RM enables customers to define the specific object they require the robot to interact with, across various levels of linguistic complexity. If a person holds a steel mug and a glass mug, they can request “the glass mug” from the robotic assistant. If the bot detects two glass mugs – one filled with espresso and the other with juice – the user can inquire about “the glass mug with espresso.” The underlying mechanisms in this characteristic domain empower such nuanced comprehension.
If someone taught me how to pick up a mug by its lip, I would easily adapt this skill to grasp other objects with similar shapes like bowls, measuring cylinders, and even rolls of tape. Researchers at MIT have faced significant challenges in developing robots with such high levels of adaptability, notes CSAIL affiliate and co-lead researcher. “F3RM seamlessly integrates geometric insight with semantic learning from large-scale fashion datasets, enabling aggressive generalization capabilities even with limited demonstration data.”
Under the guidance of Isola, Shen and Yang co-authored a paper alongside co-authors including Leslie Pack Kaelbling, principal investigator at MIT’s CSAIL, and undergraduate students Alan Yu and Jansen Wong. The staff received support from a diverse range of organizations, including Amazon.com Providers, the National Science Foundation, the Air Force Office of Scientific Research, the Office of Naval Research’s Multidisciplinary University Initiative, the Army Research Office, the MIT-IBM Watson Lab, and the MIT Quest for Intelligence. Their research will be presented at the 2023 Convention on Robotics and Artificial Intelligence.
MIT Information