Introduction
Here are numerous datasets being collected and shared regularly? The impact has indeed been a significant challenge in the market. A sense of excitement hasn’t been felt in quite some time within the machine learning community, as a groundbreaking study by an exceptional team of researchers has just been published and is sending shockwaves throughout the field. Here is the rewritten text in a professional editor’s style:
Researchers Dora Zhao and Jerome T. [last name], whose work recently received the prestigious ICML 2024 Finest Paper Award, penned a seminal paper that has made a significant impact on the field. A. Researchers Andrews, Papakyriakopoulos, and Xiang tackle a pressing issue in machine learning: the often vague and unsubstantiated claims of “diversity” in datasets. Researchers propose a framework for measuring dataset variety, dubbed “Measure Dataset Variety, Don’t Simply Declare It”, which formalizes the process of conceptualizing, operationalizing, and assessing ML dataset diversity by drawing on measurement theory principles.
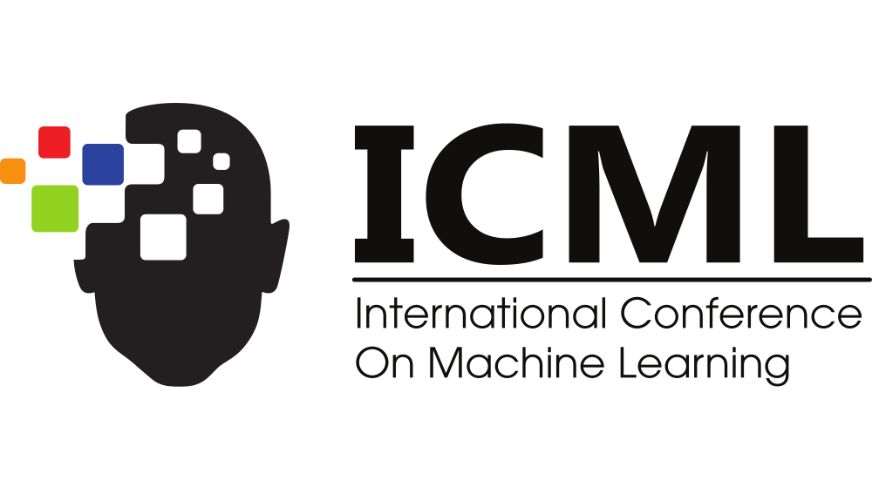
You are weighing your options carefully before making a decision, aren’t you? Is there another research paper that explores dataset variety? Don’t we already know this? But genuinely, this one’s a game-changer. Researchers scrutinize the misuse of terms like “variety,” “high quality,” and “bias” without empirical support to justify their claims. We’ve been freely exploring these concepts, which frequently prompt us to examine them more closely.
Moreover, they’re not merely identifying the problem; A novel framework has been designed to support the quantification and verification of diverse assertions. A comprehensive kit is being offered to enable us to effectively address and rectify this complex situation.
Get ready for a comprehensive examination of this pioneering research by fastening your seatbelt. Let’s explore how we’ll transition from conceptualizing measurement to quantifying it effectively. Trust us: after reading this, you’ll never examine an ML dataset in the same way again?
The Drawback with Variety Claims
Without precise definitions or validation methods, dataset curators casually employ terms like “diversity,” “biases,” and “high-quality” in their work, raising concerns about the reliability of these datasets. The absence of precision undermines replicability and reinforces the misconception that datasets are objective entities rather than value-infused products shaped by their creators’ biases and cultural backdrops?
A Framework for Measuring Variety
Researchers drawing on concepts from social sciences, particularly measurement theory, present a framework for reframing abstract notions of diversity as quantifiable constructs, thereby enabling more precise analysis and understanding. The process involves a tripartite approach:
- What does “variety” mean in the context of our dataset?
- Developing concrete approaches to quantify the distinct characteristics of diversity?
- Evaluating the dependability and accuracy of the diverse data collections.
The proposed paper calls for more precise definitions and robust validation methods in developing various datasets, suggesting the measurement concept framework as a structural support for this endeavor?
Key Findings and Suggestions
Through a comprehensive examination of 135 diverse picture and textual content datasets, the researchers unearthed several pivotal findings that shed light on the subject.
- While 52.9% of datasets provide explicit justification, it is unclear what proportion truly necessitate a wealth of information. While highlighting the importance of defining diversity in a specific manner,
- Few papers presenting datasets neglect to provide comprehensive information regarding sampling strategies and methodological choices. The authors strongly urge that dataset documentation be significantly more transparent.
- Only approximately 56.3% of datasets demonstrated robust implementation of high-quality management processes. To assess dataset homogeneity, we propose employing inter-rater agreement and test-retest reliability methods for evaluating consistency.
- Most variety claims are often unsupported by robust evidence. To ensure that datasets accurately capture the intended range of constructs, researchers are advised to employ methods from construct validity, specifically convergent and discriminant validity, in order to gauge whether these datasets genuinely represent the desired constructs.
The Sensible Software Phase Something Dataset:
The study’s foundation is exemplified through an in-depth examination of the Phase Something dataset (SA-1B), showcasing its unique characteristics. While highlighting the strengths of the SA-1B methodology, the researchers also identify opportunities for improvement, such as increasing transparency throughout data collection processes and providing robust validation for geographic diversity claims.
Broader Implications
The findings of this analysis have far-reaching consequences that directly impact the Machine Learning team.
- While some might posit that dataset size alone is sufficient to guarantee diversity, this study instead underscores the importance of purposeful selection and management in fostering a rich and varied collection.
- While pushing for increased transparency, the authors recognize the significant investment needed to make meaningful progress and urge a fundamental shift in valuing information work within machine learning research.
- The paper underscores the imperative to consider how diverse constructs may evolve over time, thereby impacting the pertinence and comprehension of datasets.
Conclusion
This ICML 2024 Finest Paper pioneers a pathway for more rigorous, lucid, and replicable investigation through the innovative application of measurement concept principles to machine learning dataset construction. As the sphere confronts challenges related to bias and representation, this framework provides invaluable tools for ensuring that claims of diversity are not merely rhetorical, but rather quantifiable and meaningful contributions to developing fair and robust AI systems.
This seminal research sparks a call to action for the ML community to elevate standards of dataset curation and documentation, ultimately yielding more reliable and fair machine learning models.
When I initially encountered the authors’ proposals for documenting and validating datasets, a portion of me reacted with skepticism, thinking, “That sounds like an awful lot of effort.” And honestly, it is. However you realize what? The task at hand. We cannot build reliable AI systems on unsteady grounds and merely expect the best results? What truly got me energized was the realization that this paper fails to significantly enhance our datasets. We aim to enhance our entire region by fostering a culture of meticulousness, transparency, and dependability. As artificial intelligence becomes increasingly pervasive, its profound impact on our lives is undeniable.
So, what do you assume? Can you quantify the diversity of datasets by meticulously calculating metrics like cardinality, uniqueness, and entropy? I’m intrigued by your enthusiasm, let’s dive into the meat of the matter and explore novel approaches to this groundbreaking study. What specific aspects would you like to dissect?
‘s right here:
Incessantly Requested Questions
Ans. Measuring dataset diversity is crucial since it guarantees that the datasets employed to train machine learning models represent a wide range of demographics and scenarios. This approach enables the mitigation of biases, boosts the transferability of fashion styles, and fosters a culture of impartiality and justice within AI applications.
Ans. Diverse datasets can significantly boost the efficacy of machine learning models by subjecting them to a range of scenarios, thereby reducing the likelihood of overfitting to specific groups or circumstances. These outcomes lead to exceptionally robust and accurate fashion solutions that perform consistently well across diverse populations and settings?
Ans. Wide-ranging hurdles arise from establishing a consensus on what constitutes diversity, translating this understanding into quantifiable metrics, and verifying the validity of diversity assertions. Furthermore, achieving transparency and ensuring reproducibility in documenting diverse datasets requires meticulous effort and a high degree of sophistication.
Ans. Sensible steps embrace:
a. Establishing distinct criteria for measuring diversity and inclusivity aligned with the organization’s core objectives.
b. Gathering data from a diverse array of sources to encompass various demographic profiles and scenarios.
c. Measuring diversity in datasets using standardized methods: A crucial step in ensuring data quality and integrity.
d. Consistently refresh and update datasets to account for evolving diversity over time.
Ensuring robust data validation techniques are in place to reliably mirror the intended data spectrum.