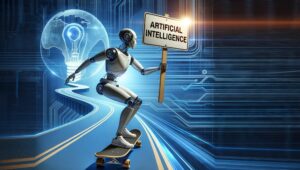
(AI Generated/Shutterstock)
The lack of a transformative “killer app” for generative AI beyond chatbots and copilot tools may impede widespread adoption. What GenAI aims for, according to analysts, involves AI-integrated goals that enable autonomous decision-making and self-execution. Can a novel, large-scale humanoid robot, referred to as the Massive Motion Mannequin (LMM), fulfill this demand?
As a response to the remarkable success of massive language models (LLMs) that captivated global attention with their human-like textual output, the LAM concept began to take shape in late 2023. Large language models (LAMs) surpass the textual content technological capabilities of large language models by genuinely performing specific actions within a software program.
According to Pankaj Chawla, chief innovation officer at Virginia-based tech consultancy , “Large Language Models excel in a conversational approach where users pose their queries and await responses.” What’s next for this project once I’ve reached this milestone? As that’s where the magic of enormous motion graphics unfolds.
Three Pillar is building Language Acquisition Modules (LAMs) for retailers who recognize the value of Large Language Models (LLMs), yet want to take it a step further by automating routine tasks to maximize their investment’s return on investment, according to Chawla, also known as PC.
Low-Code Automation Models (LAMs) execute actions by leveraging existing programmatic pathways, mirroring interactions with APIs or directly engaging with a software’s user interface, akin to robotic process automation (RPA).
As an illustration, when a government embarks on an enterprise journey, a Learning Analytics Model (LAM) can be constructed to respond to a human instruction such as “Discover economy-plus flights and a four-star resort for Milan, Italy, from October 10 through the seventeenth.” The LAM would not only provide answers but also navigate relevant systems and retrieve necessary information to secure reservations.
While LAMS may consider an alternative approach, they typically clarify where co-pilots diverge from their trajectory.
As a partner, I acknowledge that a co-pilot plays a vital role in supporting my endeavors; yet, they are distinct from a true collaborator, who actively assembles diverse elements to achieve a tangible outcome, whether business-driven or personally significant. The co-pilot briefly deviates from the main trajectory; nonetheless, the primary focus of [LAM] lies in developing an autonomous learning algorithm. Since this motion is repeated with increasing frequency, the script can refine its understanding of this behavior.
Companies employ distinct linguistic frameworks. Neurosymbolic AI refers to the fusion of neural networks and symbolic programming – a blend that enables machines to reason logically while still leveraging the strengths of deep learning. conventional deterministic programming).
And its AWS subsidiary has invested substantially in developing autonomous brokers, referred to as semi-autonomous entities that go beyond acting as coding co-pilots to handle core programming tasks. According to Andy Jassy, the former head of Amazon Web Services (AWS), third-party brokers have reportedly helped the company save 4,500 developer-years worth of time and resources spent on maintaining its Java codebase over the past two years since he took over from Jeff Bezos.
Another notable LAM instance is the private assistant, which leverages GPT-3.5-based technology to implement a LAM model interface for seamless interactions with popular websites such as Spotify, Apple Music, Midjourney, Suno, Uber, and DoorDash.
According to sources at PC Magazine, besides the current pilot, there is another instance of a LAM-type system being implemented by an organization that is also leveraging its enterprise computing suite to streamline processes and increase efficiency. According to him, Salesforce is discussing leveraging Low-Code Application Machines (LAMs) to operate seamlessly in conjunction with its own data, enabling a range of actions such as launching a marketing campaign and tracking its outputs.
In July, consulting firm McKinsey released a report highlighting the potential for brokers to fuel the next generation of Generative Artificial Intelligence (GenAI).
“We’re witnessing an evolution from knowledge-based, Gen-AI-powered instruments – such as chatbots that respond to questions and generate content – to Gen-AI-enabled ‘brokers’ that leverage foundation models to execute sophisticated, multi-step workflows across a digital realm,” consultants note. “The seamless transition from conceptual understanding to tangible action highlights our team’s exceptional expertise.”
According to McKinsey, AI brokers will be empowered to automate complex and open-ended scenarios due to their possession of several key traits, including the ability to navigate complexity; the capacity to be guided by natural language; and the capability to integrate seamlessly with existing software tools and platforms.
“Mightily visible in certain domains, such as mortgage underwriting, code documentation and modernization, and online advertising campaign creation, these ‘hyper-efficient digital coworkers’ – dubbed by McKinsey – are poised to make a significant impact.”
“While still emerging, the growth of investment in these instruments could lead to significant milestones for agent expertise and widespread deployment within the next few years.”
While acknowledging the challenges, PC recognizes that automating purposes within the LAM framework at this level presents certain obstacles to overcome. Large language models are inherently probabilistic and can easily diverge from their intended outputs, making it crucial to implement monitoring mechanisms that combine their capabilities with deterministic programming techniques to ensure optimal performance.
Currently, 3Pillar is developing a Language-Aided Model (LAM) utility designed to engage with users by posing questions; however, the Large Language Model (LLM) often deviates from its intended purpose or proposes topics not within scope.
“So, deterministic programming keeps it on track and within boundaries while still harnessing the power of large language models,” he explains. “We leverage data visualizations to inform our decisions, ensuring that solutions are meticulously tailored, precise, and free from subjective biases, as they’re grounded in concrete data sets.”
Back-office purposes offer a suitable testing ground for language AI models (LAMs), allowing companies to gauge their performance without incurring significant legal liability, according to PC. Massive software programme corporations’ built-in ERP suites possess extensive cross-industry data and multi-disciplinary workflows, capable of influencing and driving the development of LAMs and agent-based AI systems.
While the LAM concept remains a theoretical architectural idea for now, it is likely to evolve into a tangible framework as time passes. In the future, software-based frameworks may emerge, enabling corporations to expedite the development of LAM and integrate AI-powered agents more efficiently, according to PC.
“With the emergence of new frameworks, users can leverage preconfigured integrations and APIs to achieve seamless connections between commonly used software applications, much like adapters facilitate communication between enterprise systems today.” There exists potential for developing adapters for Oracle, as well as leveraging available APIs to facilitate action execution. This would enable the construction of these actions through a framework that can be further configured and customized via an intuitive interface, such as a level-and-click system with visual coding capabilities like ClickUp.
Despite initial doubts, the vast potential for consumer-facing LAMs and AI-powered brokerage platforms remains substantial, leading PC to predict that widespread adoption is simply a matter of time away.
“I foresee this development unfolding over the next two to five years,” he remarks. As you explore these emerging applications, you’ll start noticing that actual AI-driven solutions are taking shape, with the chatbot and large language model serving as fundamental building blocks. We still encounter points with hallucinations and other such issues. I anticipate that our innovations will have practical applications in the real world within a timeframe of 2-5 years.