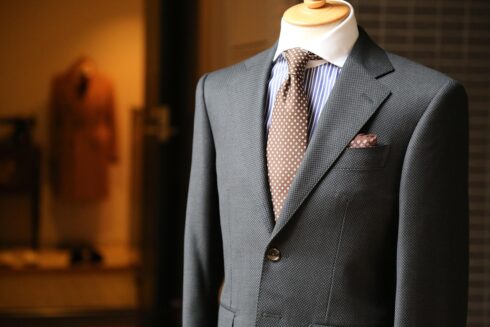
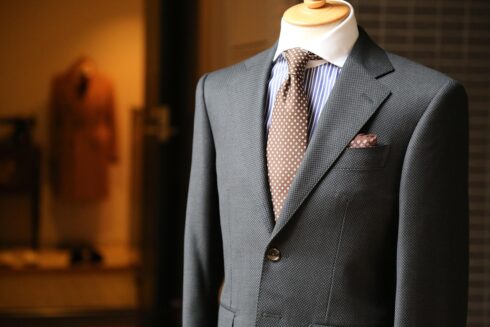
For 3 years, I’ve watched the AI coding revolution unfold with a mixture of fascination and frustration. As somebody who’s constructed and led AI engineering and product groups at Google, SwiftKey, Yahoo, and past, I’m calling it: we’re collectively falling for a productiveness mirage.
We’re celebrating typing pace whereas ignoring the precise bottlenecks that cripple software program growth and most annoy dev groups.
Builders solely spend a few hours day by day writing code, they usually hate this. They spend most of their time “not making their beer style higher”, and doing soul-sucking boring work. Scaffolding initiatives, managing tickets, refining tales, fixing bugs, dealing with tech debt, writing checks, fixing construct points… you solely want to have a look at the Stack Overflow weblog to listen to the outcry. But, as a society, we’re pouring thousands and thousands into making these 1-2 hours marginally sooner – these hours the place devs even have essentially the most enjoyable.
The issue with dopamine
We’re witnessing what I name “the 80% downside” throughout the business – a dopamine hit that hides the actual ache. What do I imply by this? I used to joke that, with dangerous administration, “the primary 80% takes 20% of the time, and the remaining 20% takes… 80% of the time”. Properly, we’re managing AI badly. Present AI instruments get you 80% of the way in which shortly, making a dopamine hit of productiveness. It’s the final 20% that wastes all of your time financial savings. It’s like sprinting the primary 19 miles of a marathon and feeling nice, however then having completely no legs to complete off the remaining 7 miles you may have left.
It’s that 20% that comprises many of the delicate bugs as a result of AI has made a litany of minute errors which have piled up, with out oversight. It’s this closing 20% that represents the precise design problem requiring human experience – and actually, that ought to have been performed in collaboration with the AI, not after the AI. The result’s a psychological mirage the place builders really feel productive initially, however crew velocity stays stubbornly unchanged. Even worse, you’re build up technical debt within the type of poor high quality that, over time, means your product and know-how begins to slowly crumble below the AI code-slop.
This tunnel imaginative and prescient is baffling when you’re a reliable supervisor. To attract on one other analogy – think about Toyota revolutionizing manufacturing by solely optimizing how shortly employees insert screws, whereas ignoring the complete manufacturing line. We’d giggle at such restricted considering. But that is exactly what’s taking place with AI coding instruments.
A current Wired survey of 730 builders captured this completely, with many seeing AI as “helpful, however clueless”—primarily a hyperefficient intern that also can’t deal with context, edge instances, or actual problem-solving. This matches precisely what I’ve noticed in enterprise environments.
Talking with CTOs throughout the business, I’ve but to search out one who can display constant, measurable enchancment in supply metrics from their AI investments. The place are the SEC filings exhibiting dependable 20+% speedups? They don’t exist, as a result of we’re optimizing the improper issues.
As an alternative, we’re reworking builders into glorified secretaries for AI – manually ferrying context between techniques, copy-pasting specs, and cleansing up hallucinated code. The bitter irony is that instruments meant to get rid of tedious work have created a brand new type of drudgery, eliminated the enjoyable, and even created new messes. You’re not utilizing the AI—you’re serving it. It’s simple to really feel productive initially, nevertheless it’s not sustainable until you convey full context to the AI… at which level you’re primarily working for the AI, not the opposite means round.
Affected by purchaser’s regret?
Given the frenzy of spending over the previous few years, I can’t simply complain, so right here’s some remedial recommendation to these CTOs who didn’t maintain the receipt on these AI coding instruments:
First, demand measurement past vainness metrics. Focus solely on time from a well-written ticket to deployment – that’s the one throughput that issues. Don’t ask devs whether or not they “really feel extra productive” as a result of they’ve outsourced considering to AIs.
Second, prioritize high quality alongside pace. You may’t settle for that writing delicate defects into your code sooner is an efficient trade-off. Context is all the pieces in engineering, and instruments that may’t entry and perceive your full growth context will all the time ship subpar outcomes. Instruments ought to uncover the context for you; why are you chasing down context for the AI? Are the instruments checks and structure docs routinely? Are they operating what they write in opposition to your checks routinely and fixing the problems? Are they operating your linters or following your most elementary coding necessities?
Third, widen your scope of optimization, don’t slender it. This feels counterintuitive – we’re taught to ship in skinny slices. However the best system enhancements come from world optimizations, not native ones. It’s like my expertise constructing IoT units: moderately than squeezing 15% higher efficiency by optimizing a power-hungry GPS chip, we solved the native downside by fixing it globally: we added a 2-cent movement sensor and a 5-cent low-power processor that triggered the GPS solely when wanted, reworking battery life solely.
The really transformative alternative lies in eradicating total steps out of your course of, not optimizing particular person ones. Why are we paying senior engineers £150k to manually create branches and scaffold boilerplate code? Why can we care in any respect about typing pace now?
Think about Stripe and Netflix – their aggressive benefit comes not from typing code sooner however from ruthlessly eliminating handoffs and bottlenecks between groups. Stripe invested closely in streamlining code critiques, testing automation, and deployments between engineering, product, QA, and operations groups. Netflix centered on automated supply pipelines and chaos engineering practices that minimized bottlenecks between dev, ops, and deployment groups, enabling fast world deployments.
This isn’t nearly effectivity – it’s a strategic benefit. Whereas others have a good time marginal coding positive aspects, firms addressing the complete growth lifecycle are getting into markets sooner and responding to buyer wants earlier than opponents even perceive the necessities. It’s the distinction between market management and irrelevance. And you’ve got a little bit of time earlier than your opponents get up and transfer on this earlier than you do – however time is shortly operating out.
The trail ahead is evident: deal with AI as a system that completes total duties, not as a glorified autocomplete. Measure success by significant DORA metrics, not strains of code generated. And demand that AI adapts to your crew’s established processes, not vice versa.
The query isn’t whether or not AI will rework software program growth. It completely will. The query is whether or not we’ll optimize what really issues. And whether or not you’re main or following.