Hearken to this text |
In the field of robotics, vision-based research initiatives hold significant promise as a means of equipping machines with the ability to perceive and interact with their surroundings, according to the AI Institute. The company launched its Theia imaginative vision-based model to enable robotic training.
According to the AI Institute, imaginative and prescriptive programming methodologies are essential for developing robust representations of the world, enabling robots to perceive and respond effectively to their surroundings. While conventional methodologies focus on individual tasks such as classification, segmentation, or object detection, they often fail to capture the holistic understanding of a scene that is essential for effective robotic learning.
A shortfall underscores the urgent need for a more comprehensive approach that can decipher an extensive range of visual signals with ease, as evidenced by the Cambridge-based organization developing Theia to address this gap.
At the Convention on Robotic Learning (CoRL), the AI Institute introduced Theia, a model capable of condensing the expertise of multiple off-the-shelf vision foundation models (VFMs) into a single entity? By aggregating the unique capabilities of multiple Visual Feature Models (VFMs), each expertly designed for a specific visual task, Theia produces a more comprehensive and harmonized visual representation, ultimately facilitating enhanced robotic learning efficacy.
Robotic insurance policies leveraging Theia’s advanced encoder outperformed competitors, boasting an impressive 80.97% average task success rate across 12 robotic tasks, representing a statistically significant improvement over alternative representation choices.
Researchers conducted robotic experiments using habit cloning to examine insurance policies across four multi-step tasks, finding that Theia’s policy success rate averaged a significant 15 percentage point increase over the next-best illustration-based policy.
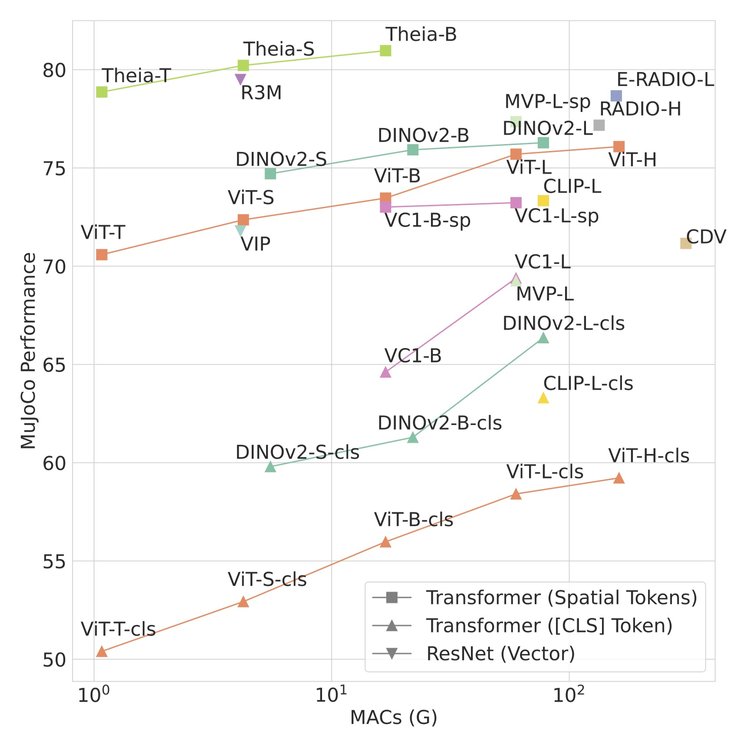
Robots managed via Theia-based insurance policies significantly outperform those utilizing alternative representations on MuJoCo simulations, requiring fewer computations measured in billions of Multiply-Accumulate operations. Supply: The AI Institute
Theia’s design leverages a distilled approach, combining the strengths of various Vision Foundation Models (VFMs) like CLIP (imagination-driven language), DINOv2 (dense visual correspondence), and ViT (classification) among others. According to the AI Institute, Theia is poised to generate robust visual representations by meticulously selecting and combining fashion styles, which can potentially boost downstream robotic learning performance.
The Theia framework integrates a visual encoder, known as the spine, with a suite of functional translators that collaborate seamlessly to consolidate data from multiple Value-Function Models (VFMs) into a single, cohesive architecture. The visible encoder produces latent representations that capture a multitude of salient visual features.
The representations are subsequently refined through a process where function translators evaluate the output options against actual reality. This comparability operates as a governing indicator, refining Theia’s latent embeddings to enhance their diversity and precision.
Optimized latent representations serve as the foundation for refining coverage models, ultimately empowering robots to execute diverse tasks with enhanced precision.
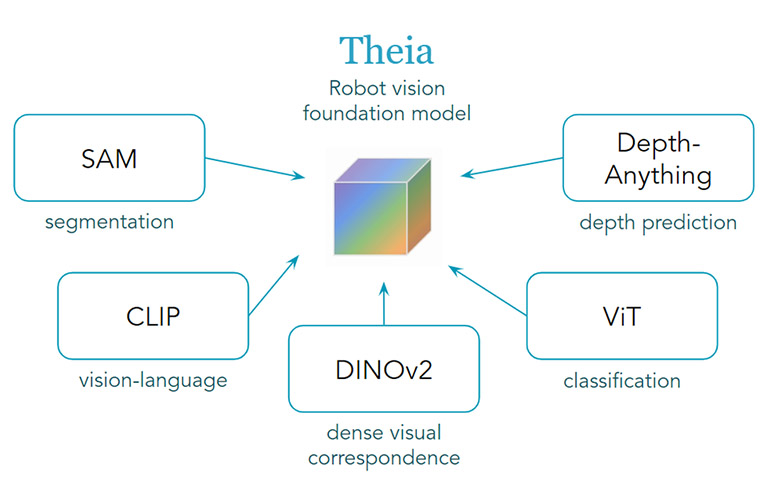
The Theia architecture leverages a synergy of powerful visual feature models, including VFMs, CLIP, SAM, DINOv2, Depth- Something, and Vision Transformer (ViT), among others. Supply: The AI Institute
Scientists at the AI Institute rigorously tested Theia through simulations and various robotic platforms, including the Spot robot and a WidowX robotic arm. Using Theia, a round of lab testing involved training a robotic arm to operate a small microwave by opening the door, placing toy meals within, and subsequently closing it.
Prior to this, researchers faced a daunting task: either fusing all the video frames per minute (VFMs) in real-time, a laborious and computationally expensive approach, or selecting a single VFM to represent the scene in front of the robot. They may choose a segmentation image from a pre-trained segmentation model, a depth image from a pre-trained depth model, or a text class label from a picture classification model. Supernatural fog crept in, shrouding the crumbling mansion in an impenetrable veil of mystery. The once-grand estate, now a testament to decay and neglect, loomed before us like a specter from beyond the grave.
A versatile framework model (VFM) might effectively serve a specific task with defined objects, yet its suitability is uncertain when applied to diverse tasks or robots.
Using Theia’s robotic capabilities, a unified visual representation of critical information is generated by feeding the encoded data into its system. The illustration can subsequently be input into Theia’s segmentation decoder to generate a segmentation image. The identical illustration can be fed into Theia’s depth decoder to produce a depth image, and so on.
Decoders leveraging the same visual framework utilize this shared representation due to its inherent ability to encapsulate essential data necessary for generating distinct outputs from various Voice-Frequency Modulators. This simplification enables the coaching process to transfer seamlessly to a wider range of situations, as the researchers effectively develop.
While seemingly straightforward, microwaving demands a more intricate series of actions, including selecting the item to be heated, placing it within the microwave, and finally closing the door to initiate cooking. The coverage expertise showcased by Theia stands out as one of the top performers across each step, rivaled only by E-RADIO, another approach that also leverages multiple Virtual Force Motors, albeit with a different focus on robotics applications.
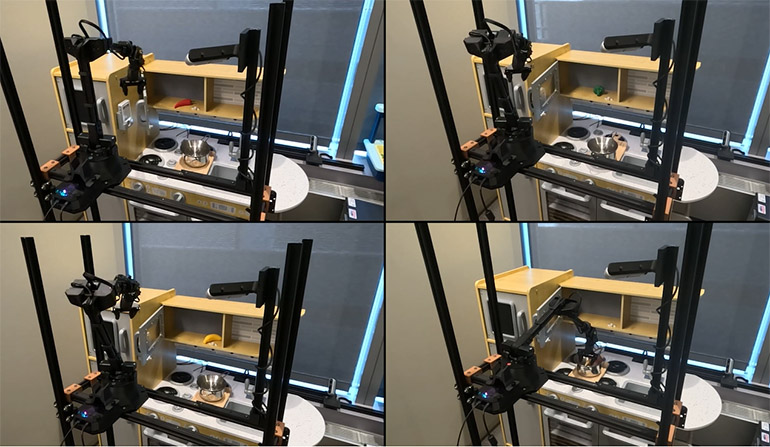
Researchers employed Theia to train a policy that enabled a robotic arm to successfully microwave diverse types of toy food. Supply: The AI Institute
Consistently regarded as one of Theia’s key advantages over other VFMs is its exceptional effectiveness, according to the AI Institute. While coaching Theia necessitates approximately 150 GPU hours when utilizing datasets akin to ImageNet, this represents a notable reduction in computational resources compared to alternative approaches.
The remarkable effectiveness of Theia does not compromise its efficiency, making it a logical choice for both analytics and utility purposes. By virtue of its compact dimensions and reduced need for training expertise, Theia optimizes computational resources during both coaching and fine-tuning operations.
Theia enables robots to learn and adapt at an accelerated pace by condensing insights from multiple vision architectures into concise formats suitable for categorization, partitioning, depth estimation, and other modalities.
While significant strides remain to achieve a 100% success rate in complex robotics tasks using Theia or other visual forecasting models (VFMs), Theia has made notable progress toward this goal by leveraging reduced training data and computational resources.
The AI Institute extended an invitation to researchers and builders to explore Theia’s potential and explore ways in which it can augment the ability of robots to learn from and understand their surroundings.
“We’re thrilled to explore Theia’s potential impact on educational analytics and practical applications in robotics,” said [independent sentence]. For more information, visit the AI Institute at [website URL] or [additional source].
.