.
As we move forward with shipping on our buyer commitments to cloud and AI innovation, our unwavering commitment to driving sustainable progress remains steadfast. As we strive to achieve our ambitious goal of becoming carbon neutral by 2030, a pivotal step involves rethinking our cloud and AI infrastructure, prioritizing energy efficiency and sustainability above all else.
We are committed to achieving a carbon-neutral objective through three key initiatives: reducing carbon emissions, generating carbon-free electricity, and actively removing carbon from the environment. Across the pillars of carbon reduction, energy efficiency, and power efficiency, fundamental principles underpin sustainability progress for both our organization and the industry at large.
What’s next for sustainable AI?
Unlocking innovative possibilities, we concentrate on three distinct realms:
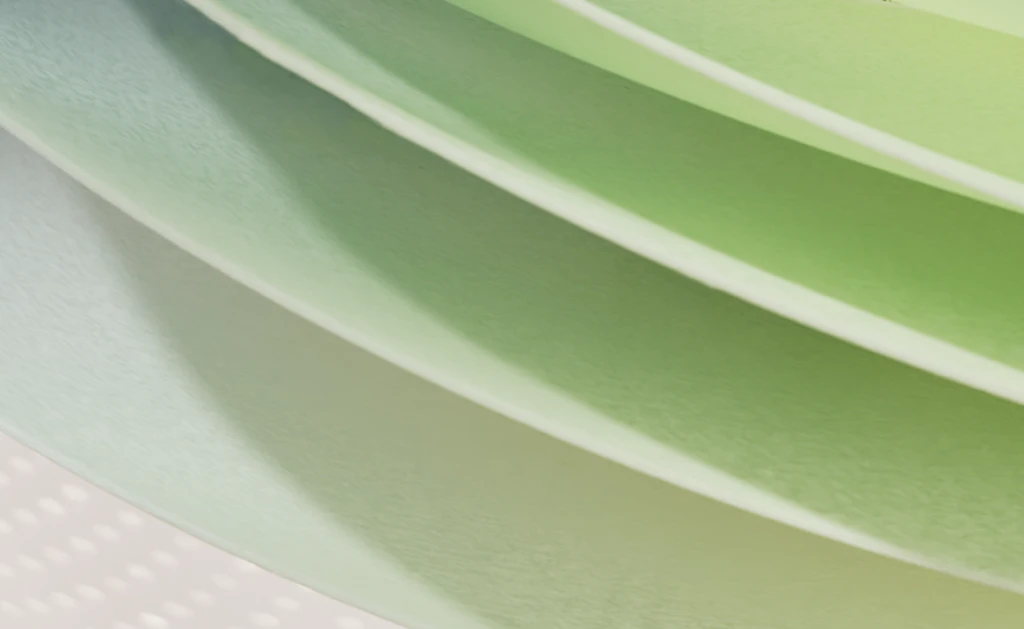
While “energy” and “power” are often synonymous, a crucial distinction exists: energy effectiveness focuses on optimizing usage patterns to mitigate peak demands, whereas power effectiveness targets reducing overall energy consumption over a prolonged period.
The distinction proves pivotal when considering the intricacies of analysis and software, as the efficacy at play dictates the specificity required. As a demonstration of efficiency, consider selecting models that require fewer parameters, allowing them to run seamlessly on your smartphone using minimal processing power. To maximise energy efficiency, consider seeking out strategies that optimise the utilisation of available energy resources through effective improvements.
Optimizing the efficiency of a hyperscale cloud and AI infrastructure system requires a holistic approach, spanning datacenters, servers, silicon, and code – algorithms and fashions that drive effectivity throughout the entire system, ultimately optimizing the performance of each component and the system’s overall operation. Significant breakthroughs in effectiveness have arisen from the tireless efforts of our research teams over time, driven by a passion for uncovering innovative ideas and advancing the global scientific community’s understanding.
On this blog, we’re excited to showcase a few compelling case studies that demonstrate the successful translation of promising effectiveness research from the laboratory to industrial operations.
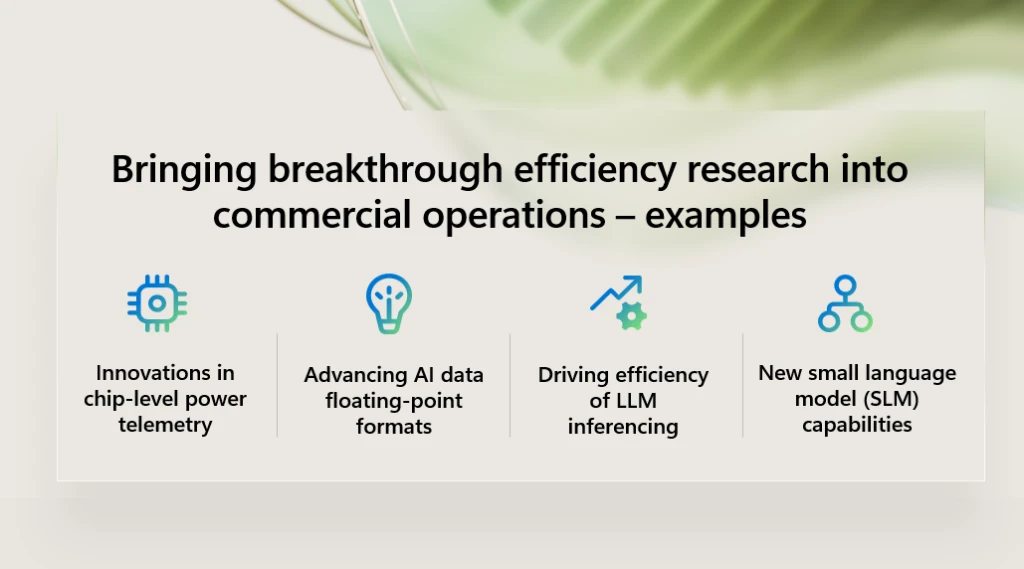
Real-time energy telemetry at the silicon level enables precise, accurate utilization insights.
With groundbreaking advancements in delivering energy telemetry, we’ve successfully extended our reach to the very edge of the silicon, thereby providing an unprecedented level of precision in energy management. Energy telemetry on the integrated circuit leverages firmware to provide insight into the facility profile of a workload while safeguarding the confidentiality of customer data and information. The software program automates air traffic control within a data center, efficiently distributing workloads across suitable servers, processors, and storage devices to maximize performance.
Developing synergies to propel forward-thinking innovation in AI-driven data compression standards.
Algorithms embedded within silicon work tirelessly to resolve complex problems by integrating existing knowledge, systematically processing it through a series of defined steps, and generating a meaningful outcome. Gigantic language models (LLMs) are trained using machine learning algorithms that process vast amounts of data to discover patterns, relationships, and structures within language.
To boost algorithm efficiency, consider reducing the precision of floating-point data formats, which are optimized numerical representations designed to process real numbers efficiently? As part of the Open Compute Challenge, we have partnered with various business leaders to develop and standardize cutting-edge 6- and 4-bit knowledge representations for AI training and inference.
By leveraging narrower codecs, silicon is empowered to perform additional environmentally sustainable AI computations per clock cycle, thereby significantly accelerating both model training and inference times. These fashion trends occupy significantly less memory space, suggesting that they demand fewer cognitive retrievals from long-term storage, allowing them to operate more efficiently and effectively. Furthermore, reducing the number of bits used in data transmission enables the conveyance of significantly less information across the interconnection, potentially leading to enhanced software performance or lower community costs.
Driving Efficiencies in Large Language Model (LLM) Inference via Phase-Splitting?
The analysis reveals a promising approach, which successfully segregates the two phases of LLM inference into distinct machines, each tailored to its respective requirements. Given the diverse needs of each phase’s resources, certain machines can deliberately reduce the power consumption of their AI accelerators or even utilize legacy hardware to achieve optimal performance. Compared to current designs, this innovative system can increase its capacity by a remarkable 2.35 times while maintaining the same energy and cost efficiency.2
What are the most impactful studies on AI effectiveness?
To enhance the efficiency of our operations, we’re focusing on empowering builders and data scientists to develop and refine AI models that deliver comparable results while minimizing resource consumption. While previous discussions have touched on the topic of small language models (SLMs), it is crucial to consider their potential advantages over large language models (LLMs) in various scenarios. For instance, SLMs can offer a more sustainable alternative for fine-tuning experiments across multiple tasks and datasets.
By April 2024, we will have established a household of open, highly successful, and cost-effective Small Language Models (SLMs) that consistently outperform their larger counterparts across a broad spectrum of language, reasoning, coding, and math benchmarks. This latest release amplifies the portfolio of premium fashion offerings, empowering customers with informed choices to design and develop innovative AI applications. We subsequently introduced a novel consulting framework, combining the expertise of 16 specialists under a single entity: Phi-3.5-MoE. Additionally, we developed the Phi-35-mini model. Each of these fashion styles is multilingual, supporting more than 20 languages.
Explore how we’re pushing the boundaries of sustainability through our thought-provoking blog series, “Sustainable by Design,” starting with…
1Algorithms play a crucial role in Large Language Models (LLMs), as they enable the processing and manipulation of vast amounts of linguistic data. By leveraging various algorithmic techniques, LLMs can learn patterns, relationships, and context-specific nuances within language, allowing for improved understanding and generation capabilities.
2, Microsoft Analysis.