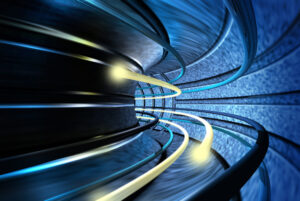
(anterovium/Shutterstock)
In today’s data-driven landscape, the ability to tap into, navigate, and capitalize on vast amounts of knowledge assets sets successful organizations apart from the rest? To stay ahead, companies must master the intricacies of synthetic intelligence’s knowledge pathways.
While the utilization of knowledge analytics, business intelligence functions, and data warehousing for structured information has reached maturity, the techniques to derive value from such data remain widely understood. Notwithstanding the explosive proliferation of generative AI, the technology now holds immense promise for unlocking the latent value in unstructured information with unprecedented efficacy. Knowledge within an enterprise often becomes fragmented across distinct silos, each possessing its unique architecture, formatting, and access procedures. Integrating diverse knowledge sources poses a significant challenge, yet it represents a crucial initial step in establishing a streamlined AI knowledge infrastructure.
As the landscape of artificial intelligence continues to rapidly evolve, companies are constantly seeking to fully leverage the power of AI-generated intelligence. Any successful AI endeavour hinges on a robust knowledge infrastructure, facilitating the smooth transfer of information from source to insight.
Breaking Down Barriers: Accelerating AI Pipelines by Bridging Information Silos
As the chasm between isolated knowledge repositories continues to widen, it has become a major impediment to the swift implementation of AI workflows within IT organizations, where the absence of streamlined processes, effective governance, and manageable complexity can lead to costly inefficiencies.
Organizations seek to harness their existing knowledge without incurring the costs of migrating unstructured data to new platforms, leveraging AI capabilities while building upon their current infrastructure. As technological advancements unfold at an accelerating pace, savvy business leaders demand the flexibility to adapt and adjust their operations seamlessly, scaling up or down, or bridging multiple platforms with existing infrastructure, without compromising access to vital information for existing customers or users. In light of the diverse applications where AI is employed, a common thread among them is the need to access information from multiple sources, often spanning disparate domains.
The fundamental issue lies in the fact that access to knowledge for individuals and AI systems alike has always been funnelled through an outdated file system, which has traditionally been integrated within the storage infrastructure, ultimately limiting its capabilities. When knowledge outstrips its storage capacity or when differing efficiency requirements or value profiles necessitate the use of alternative storage types, users must traverse multiple access paths to reach their data across incompatible systems.
The inherent limitation of AI workloads lies in their initial requirement to integrate insights from multiple sources, thereby providing a comprehensive understanding across the entirety of the information. AI workloads require unrestricted access to the entire dataset to effectively categorize and label the records, identifying which ones need refinement for the next step in the process?
As the AI journey progresses, information will continue to be refined further. The refinement process may involve refining a large language model through a combination of cleaning and fine-tuning, potentially requiring multiple iterations to achieve the desired output. As data-intensive workloads evolve, every step necessitates distinct compute and storage efficiency requirements, spanning from low-bandwidth, cost-effective mass storage devices and archives to high-speed, premium NVMe storage solutions?
The storage-centric lock-in of file techniques on the infrastructure layer has long been a source of fragmentation, and it’s not a novel challenge unique to AI applications. IT professionals have long grappled with the dilemma of either overspending on storage infrastructure to ensure seamless access to data, thereby sacrificing efficiency, or tolerating the “data duplication tax” by manually managing file replicas across disparate systems. A lingering limitation is further exacerbated in the training of AI models and during the Extract Transform Load process.
What benefits would a file system have if it were designed to separate itself completely from the infrastructure layer?
Traditional storage platforms integrate the file system into the infrastructure layer’s fabric. Despite the potential for a software-defined solution harmonious with any on-premises or cloud-based storage system from various vendors, a high-performance, cross-platform parallel file system is born, bridging disparate storage silos across multiple domains seamlessly.
By abstracting the file system from its infrastructure dependencies, automated knowledge orchestration enables unparalleled efficiency for GPU clusters, AI models, and data scientists. Everyone with a need-to-know access has read-only entry to all information across the organization. To avoid filing duplicates of identical records through this unified global metadata management system.
Streamlining Business Efficiency: Leveraging Self-Service Workflow Automation in IT Organizations
As industries such as pharmaceuticals, finance, and biotechnology rely heavily on archiving training data alongside its resulting models, the ability to automatically integrate these datasets into cost-effective resources becomes a crucial requirement. With metadata tags tracking knowledge origins, iteration details, and workflow stages, effortlessly recalling outdated model knowledge for reuse or applying a novel algorithm becomes a seamless operation that can be seamlessly automated in the background?
As IT organizations rapidly adapt to handle AI workloads, they are inadvertently perpetuating the same siloed challenges that have long plagued them. The problems have accumulated:
To effectively tackle aggressive workloads generated by emerging AI applications, knowledge entry must seamlessly span across native silos, regions, and cloud ecosystems while supporting extremely high-performance computing demands.
In highly adaptable environments where infrastructure may pose significant barriers to scaling, the need for agility becomes particularly crucial. As a result, the ability of corporations to seamlessly integrate automation across disparate data sources and rapidly scale up to cloud-based computing and storage platforms has become increasingly crucial.
Simultaneously, organizations must seamlessly integrate their existing infrastructure with these novel distributed resources while ensuring that the cost of deploying AI workloads does not outweigh the potential returns.
To stay ahead of growing demands for AI pipeline efficiency, a fresh paradigm is crucial to seamlessly connect on-premise silos and the cloud. Will innovative solutions and open-source technologies enable seamless integration, ensuring seamless scalability and interoperability across diverse infrastructures?