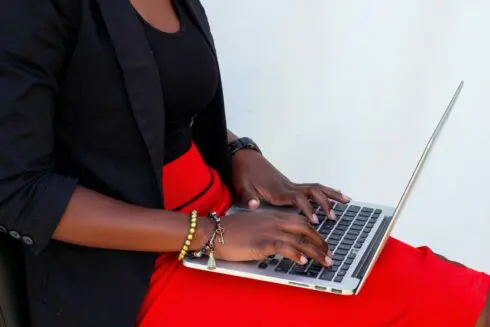
Think about looking for an important piece of knowledge in a conventional search engine, solely to be overwhelmed with hundreds of irrelevant outcomes. This limitation is very problematic in important industries like nuclear energy, the place precision and reliability are paramount. Enter sentence embeddings—a strong, but usually neglected know-how that’s set to remodel how we entry and make the most of data.
Focused sentence embedding know-how represents a major leap ahead in search platform capabilities. As an alternative of counting on easy key phrase matching, sentence embeddings convert sentences into vector representations, enabling a deeper, extra contextual understanding of queries. This implies search outcomes usually are not simply related however exact, capturing the true intent behind a question.
Traditionally, search know-how has advanced from easy key phrase matching to extra subtle semantic search. This evolution has been pushed by the necessity to enhance accuracy and relevance, particularly in domains the place precision is important and data sources are giant. Emphasis on sentence embedding know-how basically permits search platforms to know and course of data at a a lot deeper stage over huge quantities of information.
The Retrieval Problem in Essential Industries
In synthetic intelligence, it’s important to distinguish between giant language fashions (LLMs) and the specialised wants of search platforms, significantly in important industries like nuclear energy. Whereas LLMs are highly effective, they don’t seem to be a one-size-fits-all resolution. The nuclear business requires search know-how able to dealing with particular jargon and sophisticated terminology with unparalleled accuracy.
Essential purposes in nuclear energy and healthcare demand extraordinary precision. As an illustration, when a medical skilled searches for “newest pointers on radiation remedy dosage,” even a slight misinterpretation may result in dangerous outcomes. In these fields, the stakes are excessive, and even minor errors can have important penalties. Due to this fact, it’s important to develop foundational applied sciences that may precisely comprehend complicated jargon and guarantee exact data retrieval.
Hallucinations, AI, and the Fragility of the Nuclear Business
One of many challenges of generative synthetic intelligence is its tendency to hallucinate, producing inaccurate or nonsensical data. This threat is especially pronounced within the nuclear business, the place typical AI fashions, even with sturdy Retrieval Augmented Era (RAG) know-how, can falter because of the specialised language used. Retrieving inaccurate data in such a context can have dire penalties.
To mitigate this threat, it’s essential to construct a foundational understanding of nuclear phrases and nomenclature. Solely by precisely decoding and retrieving the suitable data can we make sure the reliability and security of AI purposes within the nuclear sector.
RAG know-how performs an important position in enhancing the accuracy and precision of AI outputs in instances the place updated and related data is essential. By integrating retrieval mechanisms with generative AI fashions, RAG ensures that the data generated is predicated on dependable and contextually related information. Offering irrelevant and conflicting data to an LLM results in confusion (hallucinations). This strategy is important for growing accountable and correct AI fashions in important industries like nuclear energy.
Contemplate a situation within the nuclear business the place a search question about reactor security protocols yields outdated or incorrect data. Such an error may result in the implementation of flawed security measures, placing lives and the atmosphere in danger. This instance highlights the significance of sturdy retrieval methods that precisely interpret and reply to complicated queries.
Open-source collaboration is essential for growing sentence embedding fashions in important industries. By fostering transparency and collective experience, open-source initiatives be certain that the fashions are constantly improved and validated. This strategy is especially essential within the nuclear business the place accuracy, reliability, and transparency are paramount.
Synthetic intelligence has the potential to revolutionize nuclear energy, however its utility have to be dependable and exact. Sentence embedding fashions are foundational to reaching this reliability, making an open-source strategy with business companions indispensable. As we proceed to innovate and collaborate, we’re assured that AI will play a transformative position in the way forward for nuclear energy, making certain security and effectivity at each step.