Take heed to this text |
A robot, initially trained through observation of films featuring seasoned surgeons, performed complex surgical procedures with the same level of expertise as its human counterparts.
By leveraging imitation learning in coaching, robotic surgery can transcend individualized programming for each patient transfer, bringing the field closer to achieving true autonomy, where robots can perform complex surgeries independently without human intervention?
“Skeptical at first glance, I found it astonishing to learn that a mannequin, fed only digital camera input, can accurately predict robotic actions necessary for surgery,” remarked senior creator Axel Krieger, an assistant professor in Johns Hopkins University’s Department of Mechanical Engineering. As we envision, this breakthrough represents a pivotal leap forward into uncharted territory in the realm of medical robotics.
Researchers from Stanford College collaborated with Intuitive to employ imitation learning techniques to train the da Vinci Surgical System’s robotic arm to execute three fundamental tasks: manipulating a needle, lifting physical tissue, and performing suture procedures. The robotic surgeon, trained on a human-sized mannequin, replicated complex operations with the same level of proficiency as skilled human physicians.
The mannequin merged imitation learning with the same machine learning architecture underlying ChatGPT. Despite operating on phrases and textual content, this model communicates in a “mechanical” tone, dissecting the components of robotic movement into mathematical equations.
Researchers trained a mannequin by feeding it a large dataset of videos captured from wrist cameras mounted on Da Vinci robots during various surgical procedures. Surgeons from around the globe have captured footage of their procedures, utilizing these films as a tool for assessing patients’ recoveries following surgery; once this process is complete, they are stored in an archive. More than 7,000 Da Vinci robots are utilized globally, with over 50,000 surgeons trained on the system, generating a substantial repository of expertise that can be leveraged by the robots to learn from.
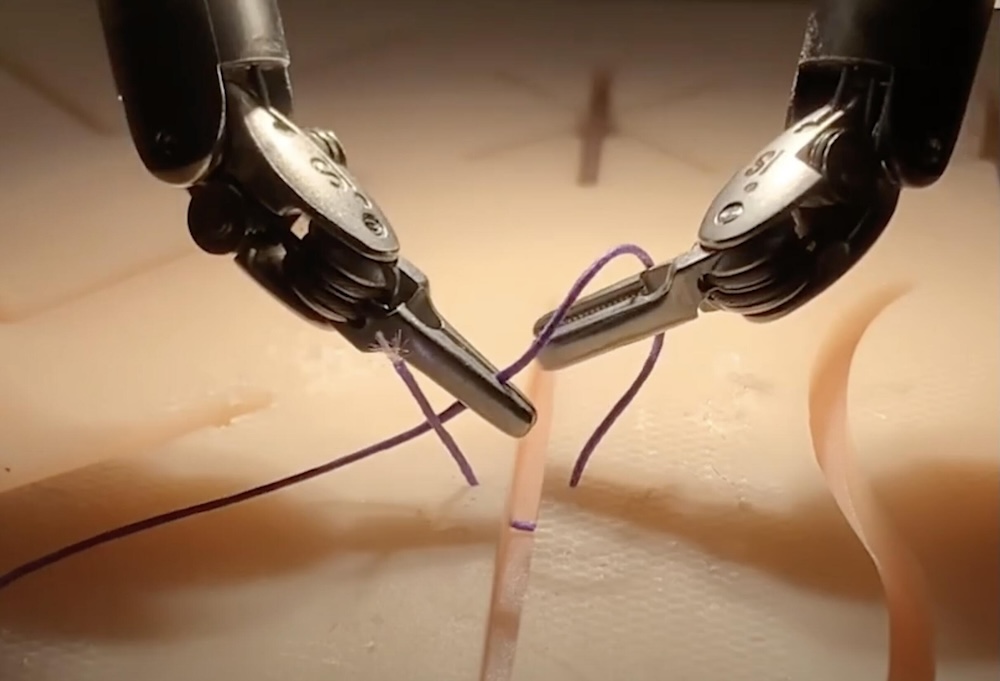
The researchers combined generative imitation learning with a modified version of the Transformer architecture used in ChatGPT. Your credit score: A vital metric measuring your financial health, just like a doctor’s report monitors your physical well-being.
Despite its widespread adoption, researchers have consistently criticized the Da Vinci system for its notorious lack of precision. Despite initial setbacks, the staff successfully found a solution that enabled the problematic input to function. What truly mattered was training the mannequin to execute relatable actions rather than rigidly adhering to absolute ones, which were inherently flawed.
Ji Woong “Brian” Kim, lead creator and postdoctoral researcher at Johns Hopkins, explained that their primary request is for users to upload a picture, after which the AI system will locate the corresponding motion. As it turns out, even after being exposed to mere hundreds of demonstrations, the algorithm is primed to learn the procedure and adapt to novel situations unseen during training.
Krieger noted that the mannequin was excelling in studying topics they hadn’t explicitly taught it. When the stylus drops, this device can effortlessly pick it back up and continue playing as usual. “This wasn’t something I ever taught it to do.”
Researchers suggest that a mannequin could be utilized to expedite the preparation of surgical robots for various surgical procedures. Staff is leveraging imitation learning techniques to train a robot to perform not just minor surgical tasks but a comprehensive surgical procedure.
.
Prior to this breakthrough, scripting every stage of a robotic’s involvement in a surgical operation demanded meticulous hand-coding. Someone might devote a decade to attempting to simulate suturing, as Krieger noted. And this is merely a small sample of the many surgical procedures that require precision suturing skills?
“It’s very limiting,” Krieger stated. “What’s truly innovative is our ability to rapidly collect imitation data on diverse processes, enabling us to train a robot to learn from them in just a few days.” It enables us to accelerate towards autonomy while significantly reducing medical errors and achieving more precise surgical procedures.
Researchers from Johns Hopkins, comprising PhD student Samuel Schmidgall, Affiliate Research Engineer Anton Deguet, and Affiliate Professor of Mechanical Engineering Marin Kobilarov, collaborate on a groundbreaking project. The research was authored by Tony Z., a PhD student at Stanford University. Researchers Zhao and Assistant Professor Chelsea Finn