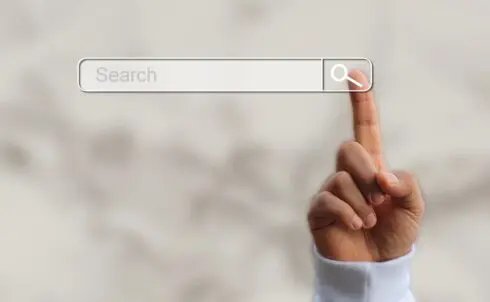
The vector database Qdrant has developed a brand new vector-based hybrid search functionality, BM42, which offers correct and environment friendly retrieval for RAG functions.
The identify is a reference to BM25, which is a textual content primarily based search that has been used as the usual in search engines like google and yahoo for the final 40 years.
In line with Qdrant, the introduction of RAG has made a number of of BM25’s assumptions now not related. As an example, the standard size of paperwork and queries is sort of totally different in RAG in comparison with net search.
“By shifting away from keyword-based search to a totally vector-based method, Qdrant units a brand new trade commonplace,” stated Andrey Vasnetsov, CTO & co-founder of Qdrant. “BM42, for brief texts that are extra distinguished in RAG situations, offers the effectivity of conventional textual content search approaches, plus the context of vectors, so is extra versatile, exact and environment friendly.”
BM42 combines the capabilities of textual content search and vector search to offer higher outcomes at decrease prices. With BM42, each sparse and dense vectors are used to pinpoint related data. The sparse vectors are used for precise time period matching, whereas dense vectors are used for semantic matching.
“Qdrant doesn’t specialise in mannequin coaching,” Vasnetsov wrote in a weblog put up. “Our core undertaking is the search engine itself. Nonetheless, we perceive that we’re not working in a vacuum. By introducing BM42, we’re stepping as much as empower our group with novel instruments for experimentation. We really consider that the sparse vectors technique is at precise degree of abstraction to yield each highly effective and versatile outcomes.”
You may additionally like…
RAG is the subsequent thrilling development for LLMs
Elastic launches low-code interface for experimenting with RAG implementation
DataStax releases plenty of updates to higher facilitate RAG implementation