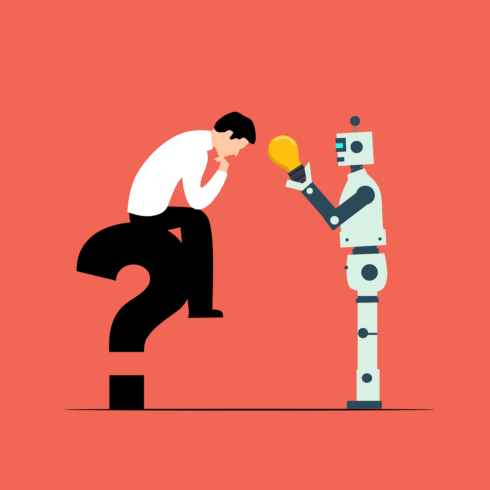
Many growth groups are starting to experiment with how they’ll use AI to profit their effectivity, however with the intention to have a profitable implementation, they should have methods to evaluate that their funding in AI is definitely offering worth proportional to that funding.
A current Gartner survey from Could of this 12 months mentioned that 49% of respondents claimed the first impediment to AI adoption is the issue in estimating and demonstrating the worth of AI initiatives.
On essentially the most current episode of our podcast What the Dev?, Madeleine Corneli, lead product supervisor of AI/ML at Exasol, joined us to share recommendations on doing simply that. Right here is an edited and abridged model of that dialog:
Jenna Barron, information editor of SD Instances: AI is all over the place. And it nearly appears unavoidable, as a result of it seems like each growth software now has some kind of AI help constructed into it. However regardless of the supply and accessibility, not all growth groups are utilizing it. And a current Gartner survey from Could of this 12 months mentioned that 49% of respondents claimed the first impediment to AI adoption is the issue in estimating and demonstrating the worth of AI initiatives. We’ll get into specifics of the way to assess the ROI later, however simply to start out our dialogue, why do you suppose corporations are struggling to reveal worth right here?
Madeleine Corneli: I believe it begins with really figuring out the suitable makes use of, and use circumstances for AI. And I believe what I hear loads each within the trade and form of simply on the earth proper now’s we now have to make use of AI, there’s this crucial to make use of AI and apply AI and be AI pushed. However for those who form of peel again the onion, what does that truly imply?
I believe a whole lot of organizations and lots of people really wrestle to reply that second query, which is what are we really making an attempt to perform? What downside are we making an attempt to unravel? And for those who don’t know what downside you’re making an attempt to unravel, you’ll be able to’t gauge whether or not or not you’ve solved the issue, or whether or not or not you’ve had any impression. So I believe that lies on the coronary heart of the wrestle to measure impression.
JB: Do you may have any recommendation for a way corporations can ask that query and, and unravel what they’re making an attempt to attain?
MC: I spent 10 years working in varied analytics industries, and I received fairly practiced at working with clients to attempt to ask these questions. And regardless that we’re speaking about AI right this moment, it’s form of the identical query that we’ve been asking for a few years, which is, what are you doing right this moment that’s exhausting? Are your clients getting annoyed? What could possibly be sooner? What could possibly be higher?
And I believe it begins with simply analyzing your enterprise or your workforce or what you’re making an attempt to perform, whether or not it’s constructing one thing or delivering one thing or creating one thing. And the place are the sticking factors? What makes that onerous?
Begin with the intent of your organization and work backwards. After which additionally whenever you’re fascinated with your folks in your workforce, what’s exhausting for them? The place do they spend a whole lot of their time? And the place are they spending time that they’re not having fun with?
And also you begin to get into like extra guide duties, and also you begin to get into like questions which are exhausting to reply, whether or not it’s enterprise questions, or simply the place do I discover this piece of knowledge?
And I believe specializing in the intent of your enterprise, and in addition the expertise of your folks, and determining the place there’s friction on these are actually good locations to start out as you try and reply these questions.
JB: So what are among the particular metrics that could possibly be used to point out the worth of AI?
MC: There’s numerous various kinds of metrics and there’s totally different frameworks that folks use to consider metrics. Enter and output metrics is one frequent method to break it down. Enter metrics are one thing you’ll be able to really change that you’ve got management over and output metrics are the issues that you simply’re really making an attempt to impression.
So a typical instance is buyer expertise. If we need to enhance buyer expertise, how will we measure that? It’s a really summary idea. You’ve got buyer expertise scores and issues like that. Nevertheless it’s an output metric, it’s one thing you tangibly need to enhance and alter, nevertheless it’s exhausting to take action. And so an enter metric is perhaps how shortly we resolve help tickets. It’s not essentially telling you you’re creating a greater buyer expertise, nevertheless it’s one thing you may have management over that does have an effect on buyer expertise?
I believe with AI, you may have each enter and output metrics. So for those who’re making an attempt to really enhance productiveness, that’s a fairly nebulous factor to measure. And so it’s important to choose these proxy metrics. So how briskly did the check take earlier than versus how briskly it takes now? And it actually depends upon the use case, proper? So for those who’re speaking about productiveness, time saved goes to be probably the greatest metrics.
Now a whole lot of AI can also be centered not on productiveness, however it’s form of experiential, proper? It’s a chatbot. It’s a widget. It’s a scoring mechanism. It’s a advice. It’s issues which are intangible in some ways. And so it’s important to use proxy metrics. And I believe, interactions with AI is an effective beginning place.
How many individuals really noticed the AI advice? How many individuals really noticed the AI rating? After which was a choice made? Or was an motion taken due to that? When you’re constructing an utility of virtually any form, you’ll be able to sometimes measure these issues. Did somebody see the AI? And did they make a selection due to it? I believe for those who can deal with these metrics, that’s a extremely good place to start out.
JB: So if a workforce begins measuring some particular metrics, and so they don’t come out favorably, is {that a} signal that they need to simply quit on AI for now? Or does it simply imply they should rework how they’re utilizing it, or possibly they don’t have some essential foundations in place that basically have to be there with the intention to meet these KPIs?
MC: It’s essential to start out with the popularity that not assembly a purpose at your first strive is okay. And particularly as we’re all very new to AI, even clients which are nonetheless evolving their analytics practices, there are many misses and failures. And that’s okay. So these are nice alternatives to study. Sometimes, for those who’re unable to hit a metric or a purpose that you simply’ve set, the very first thing you need to return to is double examine your use case.
So let’s say you constructed some AI widget that does a factor and also you’re like, I would like it to hit this quantity. Say you miss the quantity otherwise you go too far over it or one thing, the primary examine is, was that truly use of AI? Now, that’s exhausting, since you’re form of going again to the drafting board. However as a result of we’re all so new to this, and I believe as a result of folks in organizations wrestle to establish acceptable AI purposes, you do have to repeatedly ask your self that, particularly for those who’re not hitting metrics, that creates form of an existential query. And it is perhaps sure, that is the best utility of AI. So for those who can revalidate that, nice.
Then the subsequent query is, okay, we missed our metric, was it the way in which we have been making use of AI? Was it the mannequin itself? So that you begin to slim into extra particular questions. Do we want a special mannequin? Do we have to retrain our mannequin? Do we want higher knowledge?
After which it’s important to take into consideration that within the context of the expertise that you’re making an attempt to offer. It was the best mannequin and all of these issues, however have been we really delivering that have in a approach that made sense to clients or to folks utilizing this?
So these are form of just like the three ranges of questions that you should ask:
- Was it the best utility?
- Was I hitting the suitable metrics for accuracy?
- Was it delivered in a approach that is smart to my customers?
Take a look at different current podcast transcripts:
Why over half of builders are experiencing burnout
Getting previous the hype of AI growth instruments