Developing an architecture that effortlessly integrates visual cognition with linguistic comprehension to form a unified model? Here’s a next-generation vision-language model that revolutionises the frontiers of multiform tasks.
PaliGemma 2 boasts a remarkable enhancement over its predecessor, showcasing exceptional scalability and precision in applications such as fine-grained picture captioning, spatial reasoning, and medical imaging.
This article delves into the essential features, advancements, and capabilities of a particular topic, providing an in-depth exploration of its architecture, practical applications, and step-by-step execution within Google Colab. Regardless of whether you’re a researcher or a developer, PaliGemma 2 is poised to revolutionize your approach to integrating vision and language capabilities.
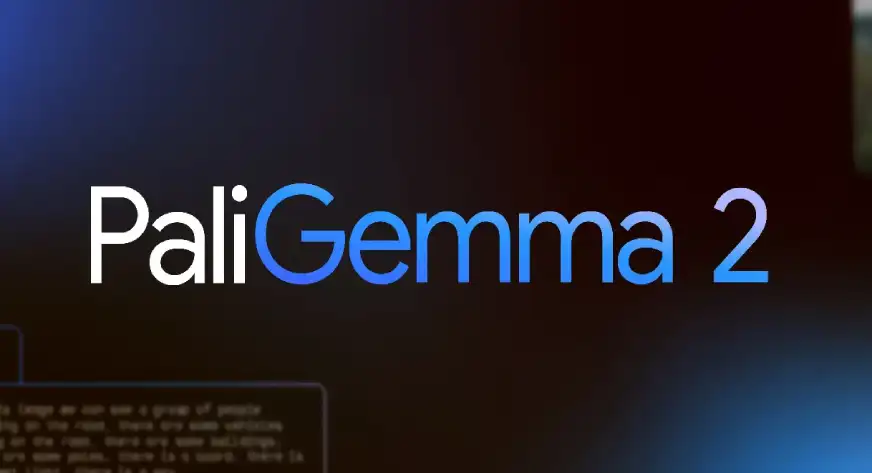
Studying Targets
- Explore the convergence of creative vision, linguistic styles, and technological advancements in PaliGemma 2 and its evolutionary trajectory across previous iterations?
- Exploiting the capabilities of PaliGemma 2 across diverse realms, including but not limited to optical character recognition, spatial reasoning, and medical imaging applications.
- Uncover the full potential of PaliGemma 2 within Google Colab for a multitude of multimodal tasks. With tasks such as organizing the surroundings, setting up the mannequin, and generating image-text combinations completed.
- Unravel the interplay between mannequin dimensions and spinal alignment to optimize operational performance? PaliGemma 2’s adaptability to specific tasks and operations could be refined by calibrating its parameters and configuring its architecture to suit the requirements of a particular duty or function, thereby optimizing its performance and accuracy in that context.
What’s PaliGemma 2?
PaliGemma revolutionizes Switch learning by seamlessly integrating the SigLIP vision encoder with the Gemma language model, pioneering a novel approach to neural network development. Given its compact 3B parameters, the device demonstrated an efficiency comparable to much larger vapor liquid mixers. Building on the foundation of its predecessor, PaliGemma 2 delivers significant enhancements. This innovative approach integrates the esteemed Gemma 2 family of linguistic styles effectively. These fashions come in three size options: 3B, 10B, and 28B. Additionally, they support resolutions of 224×224, 448×448, and 896×896 pixels. The comprehensive programme comprises a rigorous three-stage coaching process. This course equips fashion professionals with advanced fine-tuning skills, enabling them to excel in a wide range of responsibilities.
Building on the success of its precursor, PaliGemma 2 boasts a range of upgraded features that further elevate its performance capabilities. The technology’s capabilities are expanded to encompass a variety of fresh applications. These advanced tools incorporate optical character recognition, molecular construction identification, audio assessment analytics, spatial cognition capabilities, and radiology reporting systems. The mannequin has undergone rigorous evaluation across more than 30 comprehensive tutorials. Consistently exceeding expectations, this iteration surpasses its antecedent in larger model sizes and higher resolutions.
PaliGemma 2 offers an open-weight design, boasting notable versatility. This innovative technology serves as a powerful tool for both researchers and builders alike, offering unparalleled insights and capabilities. The mannequin enables investigation into the interplay between size, selection, and operational effectiveness within a controlled environment. These advancements offer more profound understandings into scaling imagination-driven vision and linguistic capabilities. The effective comprehension of this concept enables enhanced learning outcomes in switch study settings. PalisGem 2 paves the way for progressive advancements in vision-language capabilities.
Key Options of PaliGemma 2
The mannequin is capable of handling a wide range of responsibilities, including:
- Captions that evoke emotions, telling stories and transporting viewers into the heart of a moment.
- What type of information do you need to know about a photograph? Is it the date taken, location, description of what’s happening in the image, or perhaps details about the subject?
- Identifying and analyzing visual text within photographic images.
- Identifying and categorizing entities within observable information.
- Compared to the pioneering PaliGemma, this innovative model excels in terms of its significantly improved scalability and precision. The 10B parameter model exhibits a notable reduction in Non-Entailment Sentence (NES) ratings, thereby suggesting an improved accuracy of its generated outputs with regard to factual correctness.
- The PaliGemma 2 is engineered for seamless fine-tuning across a range of applications. The system accommodates various mannequin sizes (3B, 10B, and 28B parameters) and resolutions, enabling users to select configurations tailored to their specific requirements.
Innovating Futuristic and Visionary Language Styles: PaliGemma 2 Evolution
The evolution of vision-language models (VLMs) has transitioned from rudimentary architectures, including dual-encoder designs and encoder-decoder frameworks, to more sophisticated approaches that combine pre-trained vision encoders with large-scale language models. The latest advancements incorporate instruction-sensitive styles that enhance user experience by adapting answers to specific queries. Despite a surge in research focusing on scaling individual components such as vision encoders, language models, or compute resources, few studies have comprehensively examined the interplay between the selection of vision encoder and the size of language model.
Paligemma 2 effectively bridges this gap by examining the interplay between imaginative and prescriptive encoder choices and language model size. The proposed approach combines a unified strategy by harnessing the strengths of Gemma 2’s language styles and the SigLIP vision encoder, effectively integrating cutting-edge innovations to drive meaningful insights. PaliGemma 2 makes a substantial and notable addition to the field, elevating its significance within the academic or professional realm. This innovative approach enables comprehensive process comparisons, exceeding previous cutting-edge methodologies.
Mannequin Structure of PaliGemma 2
The PaliGemma 2 marks a significant leap forward in vision-language convergence, seamlessly integrating the SigLIP-So400m visual encoder with the advanced Gemma 2 family of language models to create a powerful fusion of capabilities. This integration provides a unified architecture that efficiently handles a wide range of vision-language tasks seamlessly. We delve further into the individual components and the structured coaching program behind the model’s enhanced performance capabilities.
SigLIP-So400m Imaginative and prescient Encoder
This image-to-token encoder converts photographs into discernible linguistic units. Based on the chosen resolution (224px², 448px², or 896px²), the encoder generates a series of tokens, yielding more detailed elements at higher resolutions. The tokens are then projected onto the input space of the language model using a linear transformation, enabling them to interact with the model’s architecture. This visual encoder translates images into textual tokens that can be processed and understood by the system. Based on the chosen resolution – 224px², 448px², or 896px² – the encoder generates a sequence of tokens, yielding a corresponding increase in the number of elements at higher resolutions. The tokens are then projected onto the input space of the language model through a linear transformation.
Gemma 2 Language Fashions
The Language Mannequin Element is based on the Gemma 2 platform, offering three distinct variants: 3B, 10B, and 28B. While these fashion models vary in terms of measurement and capability, larger variants are notable for their advanced language comprehension and reasoning abilities. The mixing permit’s the system to produce textual content output by autoregressively sampling from the model, leveraging concatenated input tokens.
Coaching Strategy of PaliGemma 2
PaliGemma 2 leverages a carefully crafted three-stage coaching framework, designed to optimize performance and streamline a wide range of responsibilities with maximum efficacy.
-
The imaginative and visionary encoder and language model, both pre-trained separately, are jointly trained on a combined dataset of one billion multimodal examples.
- Coaching occurs on a foundational basis of 224 pixels squared, thereby ensuring a robust and comprehensive grasp of multimodal understanding.
- Throughout this stage, all mannequin parameters remain unfrozen to allow for seamless integration of the two components.
- As the stage progresses, the mannequin is upgraded to higher resolutions – specifically, 448 pixels squared and 896 pixels squared – allowing it to excel in tasks that capitalize on increased visual detail, such as advanced optical character recognition (OCR) and enhanced spatial reasoning capabilities.
- To optimize the duty combinations, we’ve prioritized tasks demanding higher decision-making authority, while expanding the output sequence size to effectively manage complex results.
- The mannequin is fine-tuned for specific downstream tasks by utilising the checkpoints from earlier stages.
- This stage encompasses a range of educational milestones, including vision-language tasks, document comprehension, and medical imaging capabilities. Ensuring the mannequin consistently outperforms industry standards across all key performance indicators.
The desk juxtaposes distinct sizes of PaliGemma 2 fashions, each utilizing the Gemma 2 language model but potentially different vision encoders, particularly notable for employing SigLIP-So400m in the 10B model. The trade-off between manual measurements (considering multiple parameters), image selection, and computational cost during training is highlighted. Higher-end fashion demands and high-definition photography requirements significantly increase coaching costs. The availability of resources and efficiency requirements are crucial factors in determining the most suitable mannequin to utilize.
Benefits of the Structure
This modular and scalable architecture yields numerous pivotal benefits:
- PaliGemma 2’s versatility stems from its broad range of model sizes and resolutions, making it compatible with a diverse array of computational resources and processing demands.
- Enhanced Efficiency: The structured coaching model guarantees effective learning at every stage, ultimately leading to enhanced performance on complex and diverse tasks.
- Area Versatility: The ability to tailor-made for specific tasks broadens its applicability to realms encompassing molecular structure identification, musical evaluation transcription, and radiology report processing.
By converging cutting-edge visual comprehension and linguistic processing within a robust scientific training framework, PaliGemma 2 sets a groundbreaking precedent for the seamless fusion of vision and language. The platform provides a robust and flexible solution for investigators and developers addressing complex multimodal challenges.
Complete Analysis Throughout Numerous Duties
This study presents a comprehensive series of experiments assessing the efficacy of PaliGemma 2 across a broad range of vision-language tasks. These trials demonstrate the mannequin’s adaptability and capability to navigate intricate obstacles through strategic utilisation of its modular architecture, advanced training protocols, and robust vision and linguistic capabilities. Here: Underneath, we concentrate on the pivotal tasks and PaliGemma 2’s productivity in executing those tasks seamlessly.
Investigating Mannequin Dimension and Decision
One major advantage of PaliGemma 2 is its capacity for seamless scaling. We conducted experiments to investigate how scaling mannequin measurements and image choices impact efficiency. By testing the mannequin across various configurations – including models of different sizes (3B, 10B, and 28B) and resolution settings (224px², 448px², and 896px²) – we observed significant improvements in performance with larger models and higher resolutions. Notwithstanding the disparities, the benefits varied depending on the role. While certain tasks thrived on the provision of more detailed images, others derived greater value from larger linguistic models endowed with enhanced information capacity. Discoveries underscore the crucial importance of calibrating a model’s parameters to match the unique demands of a given task.
Textual content Detection and Recognition
The PaliGemma 2’s effectiveness in detecting and recognizing textual content was assessed via OCR-centric benchmarks such as those from the ICDAR’15 competition and the Whole-Text benchmark. The mannequin demonstrated exceptional prowess in identifying and parsing textual information amidst challenging scenarios, including diverse font styles, unconventional orientations, and image distortions. By integrating the SigLIP imaginative and prescient encoder’s capabilities with those of Gemma 2’s language model, PaliGemma 2 achieved groundbreaking results in both text localization and transcription, surpassing existing OCR models in terms of accuracy and reliability.
Desk Construction Recognition
The desk construction recognition process involves extracting tabular data from document images and converting it into structured formats compatible with HTML. PaliGemma 2 was trained on large-scale datasets such as PubTabNet and FinTabNet, encompassing a diverse range of tabular data. The artificial intelligence-powered mannequin showcased exceptional proficiency in analyzing desk structures, retrieving cell data, and accurately modeling table relationships. PaliGemma 2’s ability to navigate complex document layouts and constructions renders it a valuable tool for streamlining document review processes.
Molecular Construction Recognition
PalGemma 2 has also demonstrated its efficiency in molecular construction recognition tasks. Trained on a dataset of molecular drawings, the AI-powered mannequin is capable of extracting molecular graph constructions from images and generating corresponding SMILES strings with precision. With unparalleled accuracy, the PaliGemma 2 model efficiently translates molecular representations from images into text-based formats, surpassing current benchmarks and highlighting its capabilities in scientific applications demanding exceptional visual recognition and interpretation?
Optical Music Rating Recognition
The PaliGemma 2 demonstrated exceptional proficiency in optical music recognition ratings. The AI system seamlessly converted images of piano sheet music into a digital notation format. The mannequin was precisely calibrated on the extensive GrandStaff dataset. This finetuning significantly reduced errors in character, image, and line recognition compared to current methods. The display demonstrated the mannequin’s ability to understand and interpret complex visual information. Capable of transforming visible information into meaningful, structured outputs, this technology also showcased its ability to process and convert data into actionable insights. This success further underscores the model’s versatility across domains such as music and the humanities, showcasing its adaptability in a range of areas.
Producing Lengthy, Wonderful-Grained Captions
Crafting precise and informative photo captions demands an in-depth comprehension of the visual elements and their nuanced connections. The PaliGemma 2 model was assessed using the DOCCI dataset, comprising images paired with human-provided captions. The AI-powered mannequin showcased its ability to generate accurate, detailed descriptions of visual content, accurately capturing complex information about depicted objects, their spatial arrangements, and the actions they perform within the image. Compared to various vision-language frameworks, PaliGemma 2 excelled in factual alignment, generating more coherent and contextually accurate descriptions.
Spatial Reasoning
The assessment of spatial reasoning abilities, which involve comprehending object relationships within an image, has been investigated through the Visible Spatial Reasoning benchmark. The PaliGemma 2 system excelled in its task performance, accurately verifying the truthfulness of claims regarding spatial connections within visual images. The mannequin’s ability to process and manipulate complex spatial configurations enables it to handle tasks demanding exceptional visual comprehension and logical reasoning capabilities.
Radiography Report Technology
In the medical field, PaliGemma 2 was applied to radiology report technology, leveraging chest X-ray images and corresponding narratives from the MIMIC-CXR dataset. A machine learning-based algorithm successfully created comprehensive radiology reports, achieving unprecedented levels of accuracy and effectiveness in metrics such as the RadGraph F1-score. By harnessing the power of artificial intelligence, this innovative technology enables automated generation of accurate, text-based summaries from radiological images, thereby streamlining the workflow and enhancing the decision-making capabilities of healthcare professionals.
These trials demonstrate the adaptability and robust effectiveness of PaliGemma 2 across a range of vision-language tasks. Regardless of whether it’s document comprehension, molecular assessment, music identification, or medical imaging, the model’s capacity to handle complex multimodal challenges renders it a potent tool for both research and practical applications. What’s more, its impressive scalability and efficiency across various domains make PaliGemma 2 an outstanding model in the ever-changing landscape of vision-language convergence?
CPU Inference and Quantization
The PaliGemma 2’s efficiency was further assessed in terms of its ability to perform inference tasks on CPUs, examining the extent to which quantization affects both effectiveness and accuracy. While graphics processing units (GPUs) and tensor processing units (TPUs) are often preferred for their computational prowess, central processing unit (CPU) inference remains crucial in scenarios where resources are limited, such as in edge devices and cellular environments.
CPU Inference Efficiency
Numerous CPU architecture tests have validated that, despite inferencing being slower compared to GPUs or TPUs, the PaliGemma 2 can still deliver efficient performance. This ensures a cost-effective option for deploying systems where dedicated hardware accelerators are unavailable, thereby providing fast processing capabilities for everyday tasks.
Impact of Quantization on Efficiency and Precision?
To further enhance effectiveness, we leveraged quantization techniques in conjunction with 8-bit floating-point and combined precision, successfully reducing memory usage and accelerating inference. Quantization was shown to significantly accelerate processing speed without incurring a significant degradation of accuracy. The quantized model performed virtually indistinguishably from its full-precision counterpart in tasks such as image captioning and question answering, offering a more resource-efficient solution for constrained environments.
With its ability to efficiently execute computations, particularly when combined with quantization techniques, the PaliGemma 2 model demonstrates exceptional versatility and performance, making it an ideal candidate for deployment across diverse hardware platforms. These capabilities render it an ideal choice for deployment in resource-constrained environments without sacrificing performance.
Purposes of PaliGemma 2
PaliGemma 2 possesses vast potential applications across numerous disciplines, including but not limited to.
- It has the potential to create descriptions that can aid visually impaired customers in comprehending their surroundings more effectively.
- Artificial intelligence models show potential in generating narratives from medical images such as chest X-rays, with the mannequin serving as a promising tool for this purpose.
- By potentially facilitating comprehension of intricate visual data linked to charts or spreadsheets,
General, PaliGemma 2 marks a significant advancement in vision-language modeling, facilitating more nuanced interactions between visual inputs and natural language processing.
Can you enhance picture-to-text technology using PaliGemma 2 in Google Colab by tweaking the parameters and fine-tuning the model for optimal performance, considering factors such as image resolution, object detection, and contextual understanding to produce accurate and descriptive text summaries?
To utilize PaliGemma2 for Picture-to-Text technology within Google Colab, we will examine the necessary steps.
Step1: Set Up Your Setting
Before leveraging PaliGemma2, we need to configure our environment within Google Colab. You will need to install a limited number of libraries, specifically those related to transformers, PyTorch, and the Python Imaging Library (Pillow). The necessary libraries for loading the mannequin and processing photographs include:
Run the next instructions in a Colab cell:
!pip install transformers !pip install torch !pip install pillow
Step2: Log into Hugging Face
To successfully authenticate and access entry fashions hosted on the Hugging Face platform, you will need to log in using your valid Hugging Face credentials. When using a personalized mannequin, you’ll need to log in to access it.
Please run the following command in a Colab cell to login:
from google.colab import drive
drive.mount(‘/content/gdrive’)
!huggingface-cli login
You will be prompted to enter your Hugging Face authentication token. You can obtain this token by accessing your Hugging Face account settings directly.
The processor loads a mannequin with intricate details, precision-crafted to simulate human-like movements.
Let’s load the PaliGemma2 mannequin and processor from Hugging Face. The AutoProcessor handles preprocessing for visual and textual inputs, while PaliGemmaForConditionalGeneration generates the desired output seamlessly.
SKIP
from transformers import AutoProcessor, PalabGemmaForConditionalGeneration from PIL import Image import requests mannequin = PalabGemmaForConditionalGeneration.from_pretrained('google/palab-gemma-test-224px-hf') processor = AutoProcessor.from_pretrained('google/palab-gemma-test-224px-hf')
The mannequin asks, “In what location does this cow stand?” The image is retrieved from a specified URL by leveraging the capabilities of the requests library, after which it is seamlessly displayed using the versatile Pillow tool. The processor instantly converts pictures and text into a format compatible with the expected model.
url = "https://huggingface.co/gv-hf/PaliGemma-test-224px-hf/fundamental/cow_beach_1.png" picture = Picture.open(requests.get(url, stream=True).raw) inputs = processor(photos=picture, text="What is the place where the cow stands?", return_tensors="pt") generate_ids = model.generate(**inputs, max_length=30) output = processor.batch_decode(generate_ids, skip_special_tokens=True, clean_up_tokenization_spaces=False)[0] print(output)
The mannequin produces a solution primarily driven by the image and the query prompt. The response is then converted from the mannequin’s output tokens into human-readable written text. The results displayed as an easy-to-read reply, corresponding to “beach”, based on the content of the image.
To swiftly integrate PaliGemma2 into your Google Colab workflow for image-text applications, simply follow these straightforward procedures: This setup enables users to process images and text, generating meaningful responses across various scenarios. Unleash the versatility of this cutting-edge model by testing its abilities with an array of diverse prompts and images.
Conclusion
PaliGemma 2 represents a significant milestone in vision-language paradigms, integrating the highly successful SigLIP visual encoder with the Gemma 2 language model. This cutting-edge technology outshines its precursor, boasting superior performance in a range of applications, including optical character recognition, spatial analysis, and medical diagnostics. With its modular architecture, seamless fine-tuning options, and adaptable weight configuration, the PaliGemma 2 delivers robust performance across an array of tasks. It’s designed to efficiently run on CPUs, making it a preferred choice for deployment in resource-constrained environments due to its quantization capabilities.
PaliGemma 2 represents a significant leap forward in the convergence of visual perception, language understanding, and artificial intelligence capabilities, opening up new possibilities for AI-driven applications.
Key Takeaways
- The PaliGemma 2 fusion of SigLIP’s imaginative and prescient encoder and Gemma 2’s language model excels in applications such as optical character recognition, spatial reasoning, and medical image analysis.
- The mannequin offers a range of configurations (3B, 10B, and 28B parameters) and picture resolutions (224px, 448px, and 896px), allowing for adaptability across various tasks and computing resources.
- The model consistently yields outstanding results across more than 30 performance metrics, outperforming its predecessors in both precision and efficacy, with notable improvements observed at higher resolutions and larger model architectures.
- PaliGemma 2 is optimised to operate efficiently on CPUs utilising quantisation techniques, thereby enabling seamless deployment on edge devices without compromising performance.
Ceaselessly Requested Questions
A. The PaliGemma 2 is a cutting-edge vision-language model that seamlessly fuses the SigLIP visual encoder with the Gemma 2 language architecture. The advanced AI system is engineered to tackle an array of complex tasks, including optical character recognition, spatial analysis, medical image processing, and more, showcasing enhanced productivity compared to its preceding iteration.
A. PaliGemma 2 leverages the advanced Gemma 2 language model to significantly enhance the original mannequin’s capabilities, offering a range of scalable configurations with increasingly larger parameter sizes (3 billion, 10 billion, and 28 billion) and higher image resolutions (224 pixels, 448 pixels, and 896 pixels). It surpasses its predecessor in terms of precision, adaptability, and versatility across diverse tasks.
A. Paligenome 2 excels in its ability to perform a wide range of tasks, including picture captioning, visual question answering, optical character recognition, object detection, molecular structure recognition, and medical imaging report analysis.
A. In Google Colab, PaliGemma 2 can be readily employed for image-text applications, requiring the installation of fundamental libraries such as Transformers and PyTorch to streamline the environment. After loading the mannequin and processing images, you can generate responses to text-based prompts related to visual content.
A. PaliGemma 2 optimizes quantization for enhanced efficiency, allowing deployment on CPUs, making it suitable for resource-constrained environments such as edge devices or mobile applications.