Introduction
Recently, textual content-to-image synthesis and image-text contrastive learning have emerged as two leading multimodal research areas gaining significant traction. With their advanced capabilities for imaginative visual representation and manipulation, these styles have dramatically transformed the research community, attracting considerable public interest and scrutiny?
Launched with the intention of facilitating additional analysis, Imagen was deployed.
This diffusion model excels at generating photorealistic images and demonstrates exceptional comprehension of language, seamlessly integrating the power of transformer-based language models with high-fidelity diffusion techniques to achieve cutting-edge text-to-image synthesis capabilities.
The coaching and evaluation of Google’s latest Image model, ImageNet 3. Image 3 can be set to default to a resolution of 1024 × 1024 pixels, allowing users to upscale their photos by factors of 2, 4, or 8 if desired. Our analyses and assessments are benchmarked against leading-edge trends in translation technology innovation.
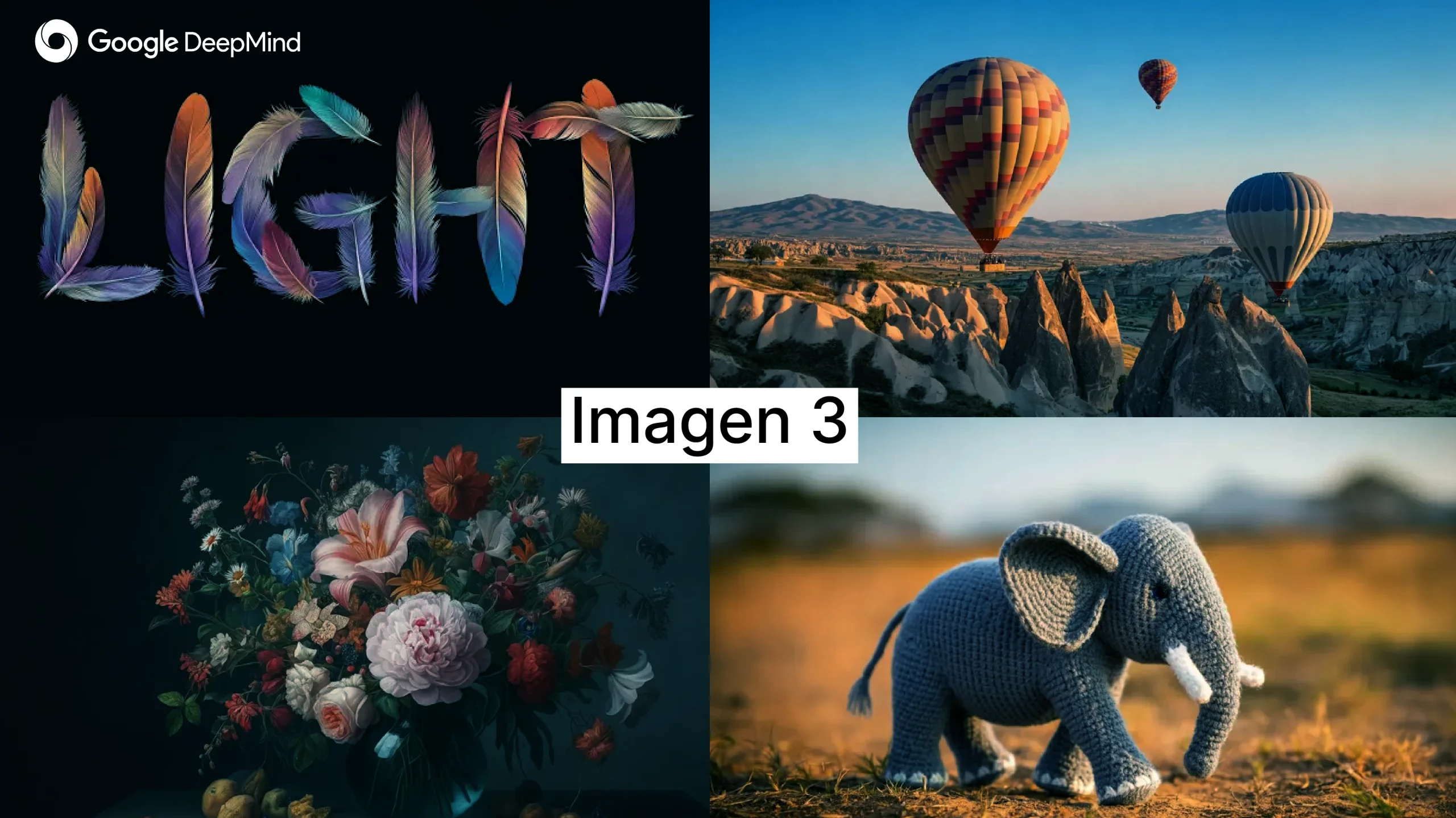
Overview
- Google’s Imagen AI, a cutting-edge text-to-image diffusion model, achieves unparalleled photorealism and accuracy in interpreting intricate customer requests with precision.
- Imagen 3 outshines its competitors, including DALL·E 3 and Stable Diffusion, in both machine-driven and human-led assessments of prompt-image alignment and visual allure.
- The coaching dataset undergoes rigorous filtering to eliminate low-quality or potentially harmful content, thereby ensuring safer and more accurate outcomes.
-
Using a powerful frozen T5-XXL encoder in conjunction with advanced multi-step upsampling technology, Imagen 3 produces exceptionally high-quality images at resolutions of up to 1024 x 1024 pixels.
- Imagen 3 is seamlessly integrated with Google Cloud’s Vertex AI, enabling effortless combination with manufacturing environments to pioneer innovative image technologies.
- With the launch of Imagen 3 Quick, businesses can now benefit from a 40% reduction in latency without sacrificing image quality?
What measures can be taken to ensure high-quality and secure coaching for individuals?
The model is trained on a vast dataset comprising textual data, images, and corresponding labels. DeepMind employed multiple layers of filtering to guarantee the highest standards of quality and security requirements. Photos deemed harmful, violent, or of poor quality are promptly removed from consideration. Subsequently, DeepMind removed photos generated by AI to prevent the model from learning biases or artifacts inherent in such images. To mitigate the risk of overfitting, DeepMind implemented a combination of techniques, including down-weighting similar images and deduplication methods, to prevent the model from relying too heavily on specific training data features.
Within the dataset, every image is accompanied by both artificially generated and genuine captions, which are derived from a combination of alt text, human-written descriptions, and other sources. Geminifashions crafts innovative, synthetic captions that diverge from traditional cues entirely. To enhance the linguistic diversity and accuracy of generated artificial captions, DeepMind employed various Gemini styles and guidelines. DeepMind employed a range of sophisticated filters to scrub potentially hazardous captions and eliminate personally identifiable information.
Structure of Imagen
The Imagen model employs a large-scale frozen T5-XXL encoder to convert all textual input into meaningful embeddings. A conditional model maps the textual content embedding into a 64×64 image. Imagining additional applications harnesses the power of text-conditioned super-resolution diffusion models to upscale images from 64×64 to 256×256 and 256×256 to 1024×1024.
Analysis of Imagen Fashions
DeepMind assesses its state-of-the-art model against top-notch configurations from DALL·E 3, Midjourney v6, Steady Diffusion 3 Giant, and Steady Diffusion XL 1.0 to determine the premier setup. DeepMind has achieved a groundbreaking milestone in text-to-image technology, demonstrating unparalleled excellence through exhaustive human and machine assessments. The study’s results demonstrated significant improvements in patient satisfaction, with 85% reporting higher levels of care compared to previous years? Product integrations with Imagen 3 may yield a level of efficiency that is significantly different from what was initially configured.
Additionally learn:
What factors contributed to raters’ high judgments of ImageNet 3’s output quality?
Candidates are assessed across five key evaluation criteria: Total Desire, Prompt-Image Alignment, Visible Enchantment, Detailed Prompt-Image Alignment, and Numerical Reasoning. To ensure independent assessments and avoid conflation in raters’ judgments, these points are evaluated separately. Quantitative judgments are typically assessed through side-by-side comparisons, whereas numeric reasoning can be evaluated instantly by simply counting the number of specific objects present in an image.
The entire Elo scoreboard is computed through a comprehensive pairwise comparison of all fashion models. Each examination comprises 2,500 points, evenly dispersed across numerous prompts within a specific set. Fashions are anonymized within the rater’s interface, with edge randomness applied to each scored instance. With a focus on data quality and employee well-being, our knowledge assortment process adheres to Google DeepMind’s highest standards for information enrichment, ensuring that all personnel involved in this process receive a living wage commensurate with their skills and expertise. The examiner gathered a total of 366,569 scores, resulting from 5943 submissions made by 3225 distinct raters. Each rater contributed minimally to the study, comprising no more than 10% of the overall research, while providing approximately 2% of the total scores to mitigate potential biases stemming from individual raters’ assessments. Raters from 71 distinct nationalities worldwide took part in the research.
Total Consumer Demand Drives Imagene 3 to Leadership in Artistic Image Processing Technology?
Customers’ initial impression of the generated picture remains an open question, as evaluators prioritize the most critical high-quality aspects. If both photos were equally captivating, I’d remain impartial.
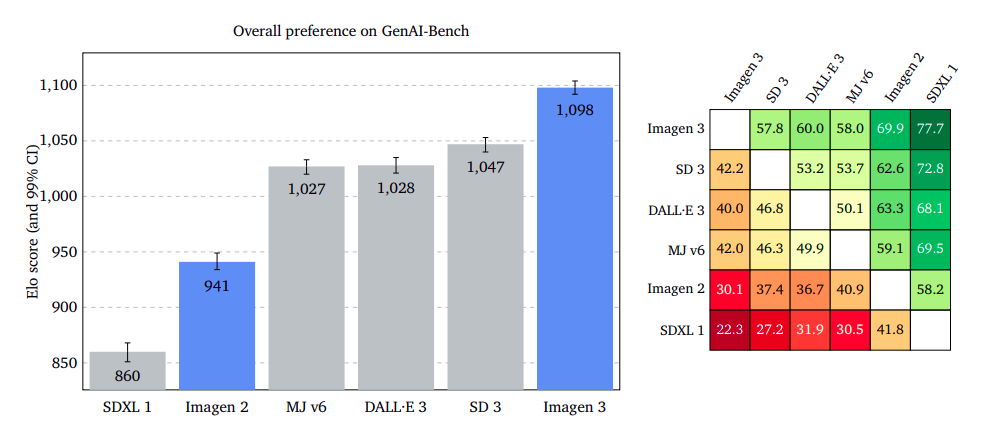
According to the outcomes, Imagen 3 emerged as a clear favorite across GenAI-Bench, DrawBench, and DALL·E 3 Eval, demonstrating a significant edge in popularity. The three models, namely Imagen, 3, led with a slightly reduced margin on the DrawBench platform, whereas they maintained a slim lead on the DALL·E 3 Eval dataset.
Instant-Image Synching: Pinpointing Customer Purpose with Unwavering Accuracy
The examination assesses the representation of an input image within a given output content, disregarding any imperfections or visual appeal. Raters are asked to evaluate a picture and determine which one most effectively conveys the intended message, regardless of its genre or style. Our results indicate that Imagen 3 surpasses GenAI-Bench, DrawBench, and DALL·E 3 Eval, as evidenced by the convergence of confidence intervals. The examination suggests that discounting possible flaws or inferior quality in images can boost the precision of image-to-prompt alignment.
What drives user engagement across various digital platforms? It’s the subtle yet powerful allure of aesthetic excellence.
Measuring visible enchantment provides an objective assessment of the captivating nature of generated images, regardless of their subject matter or context. Raters evaluate two photographs element-by-element without any specific instructions. Midjourney v6 excels on multiple fronts, closely matching Imagen 3’s performance on the GenAI-Bench, while boasting a slight edge on DrawBench and demonstrating a significant advantage on DALL·E 3 Eval.
Detailed Immediate-Picture Alignment
The examiner assesses the DOCCI’s ability to align textual prompts with corresponding images by generating photographs from lengthy descriptions significantly surpassing traditional input lengths. The researchers found that attempting to evaluate over 100 phrase prompts proved a daunting task for human assessors. By utilizing high-caliber captioned reference images, they effectively paired the generated photographs with trusted benchmark reference visuals. The raters focused primarily on the semantic content of the photographs, disregarding camera types, capture methods, and image quality. The results validated that ImageNet 3 exhibited a substantial gap of +114 Elo points, coupled with a 63% winning rate against its closest competitor, underscoring its exceptional aptitude for accurately tracking intricate details within input prompts.
Numerical Reasoning: Optimizing Object Recognition Precision through Data-Driven Insights
The examination assesses the capacity of fashion designs to yield a genuine array of items through the GeckoNum benchmarking methodology. The task requires swift assessment of visual elements against predetermined targets within a specified timeframe. Fashion considerations often revolve around attributes such as color and spatial relationships. According to the results, ImageNet 3 emerges as the most effective model, surpassing DALL·E 3 by a significant margin of 12 times. This AI model exhibits enhanced precision in generating images featuring 2-5 subjects, while also demonstrating increased efficiency in processing complex sentence structures.
Can we benchmark fashion trends against AI-generated scores?
Recently, automatic evaluation metrics such as CLIP and VQA Score have become increasingly popular for assessing the quality of text-to-image models. This examination concentrates on automatic evaluation metrics for real-time image alignment and picture quality enhancement to complement human assessments.
Immediate–Picture Alignment
The researchers choose three robust auto-eval prompt-image alignment metrics, consisting of contrastive twin encoders (CLIP), VQA-based (Gecko), and a language-visual learning model (LVLM) prompt-driven approach, an implementation of VQAScore2. While CLIP frequently falls short in predicting correct model rankings, Gecko and VQAScore demonstrate impressive accuracy, concordant in their assessments a remarkable 72% of the time. While VQAScore’s accuracy is impressive at 80%, outperforming Gecko’s 73.3%, one wonders what drives this significant difference. The Gecko’s utilization of a PALI spine with reduced strength enables it to achieve superior efficiency.
The examiner assesses four datasets to investigate model variations under different scenarios: Gecko-Rel, DOCCI-Test-Pivots, DALL-E 3 Evaluation, and GenAI-Bench. The outcomes demonstrate that Image 3 consistently achieves the highest alignment efficiency. The SDXL 1 and Imagen 2 models consistently demonstrate subpar performance compared to other styles?
Picture High quality
Researchers assess the distribution of images produced by Imagen 3, SDXL 1, and E 3 on a 30,000-sample subset of the MSCOCO-caption validation set, employing various feature spaces and distance metrics to evaluate high-quality picture generation. By optimizing these three key performance indicators, they acknowledge a delicate balance must be struck – prioritizing the precision of pure colors and textures while sacrificing some accuracy in detecting distortions on object forms and features. The Imagen 3 model showcases a notable decline in CMMD value across its three fashion variations, with impressive performance on state-of-the-art functionality benchmarks.
What qualitative outcomes can we expect when Imagen 3 prioritizes the element of?
The image below showcases two photographs upscaled to 12 megapixels, featuring cropped segments that demonstrate the level of detail achieved through this process.
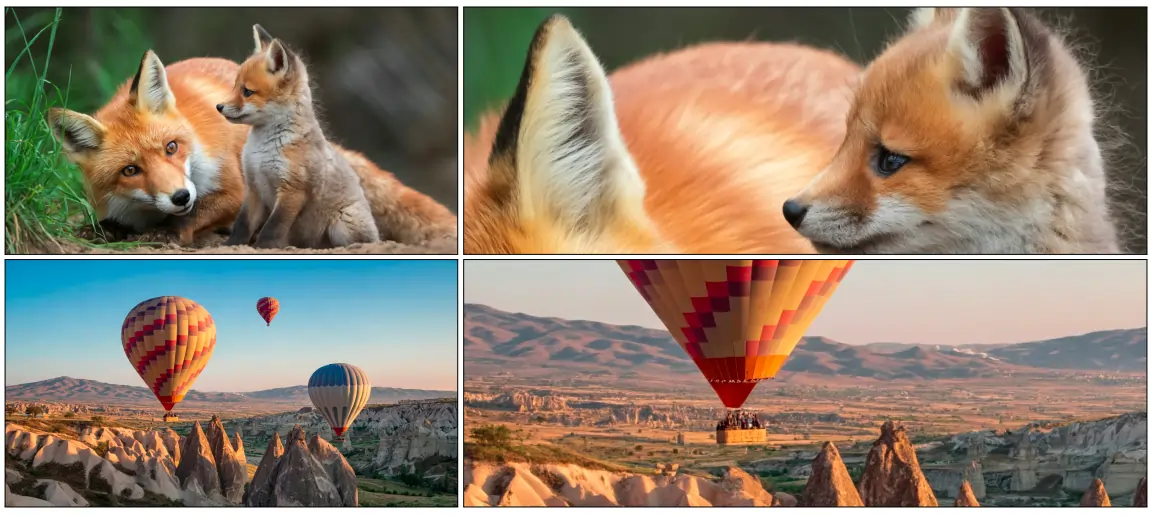
Inference on Analysis
Image 3 stands out as the most accurate in terms of prompt-image alignment, particularly when it comes to processing intricate details and numeracy skills. By virtue of its visible allure, V6 seizes the top spot, with Imagen 3 following closely behind in second place. Despite its strengths, the model still exhibits limitations in areas such as numerical reasoning, scaling concepts, complex sentence structures, action comprehension, spatial awareness, and nuanced linguistic understanding. Fashions grapple with the demands of numerical comprehension, spatial scaling, and linguistic complexity, as well as the need for swift execution. Ultimately, Image 3 stands out as the sole viable choice for producing high-caliber results that accurately align with consumer preferences.
Unlocking Seamless Integration with Imagen 3 and Vertex AI
Utilizing Vertex AI
To start using this, you need to have an existing Google Cloud project set up. Study extra about .
Additionally, the GitHub hyperlink is:
import vertexai from vertexai.preview.vision_models import ImageGenerationModel project_id = "PROJECT_ID" vertexai.init(venture=project_id, location="us-central1") generation_model = ImageGenerationModel.from_pretrained("imagen-3.0-generate-001") immediate_description = """A photorealistic image depicts a cookbook resting on a wooden kitchen desk, with a quilt flowing forward showcasing a smiling family seated at the same desk. Delicate overhead lighting illuminates the scene, with the cookbook serving as the main focal point.""" picture = generation_model.generate_images(immediate=immediate_description, number_of_images=1, aspect_ratio="1:1", safety_filter_level="block_some", person_generation="allow_all")
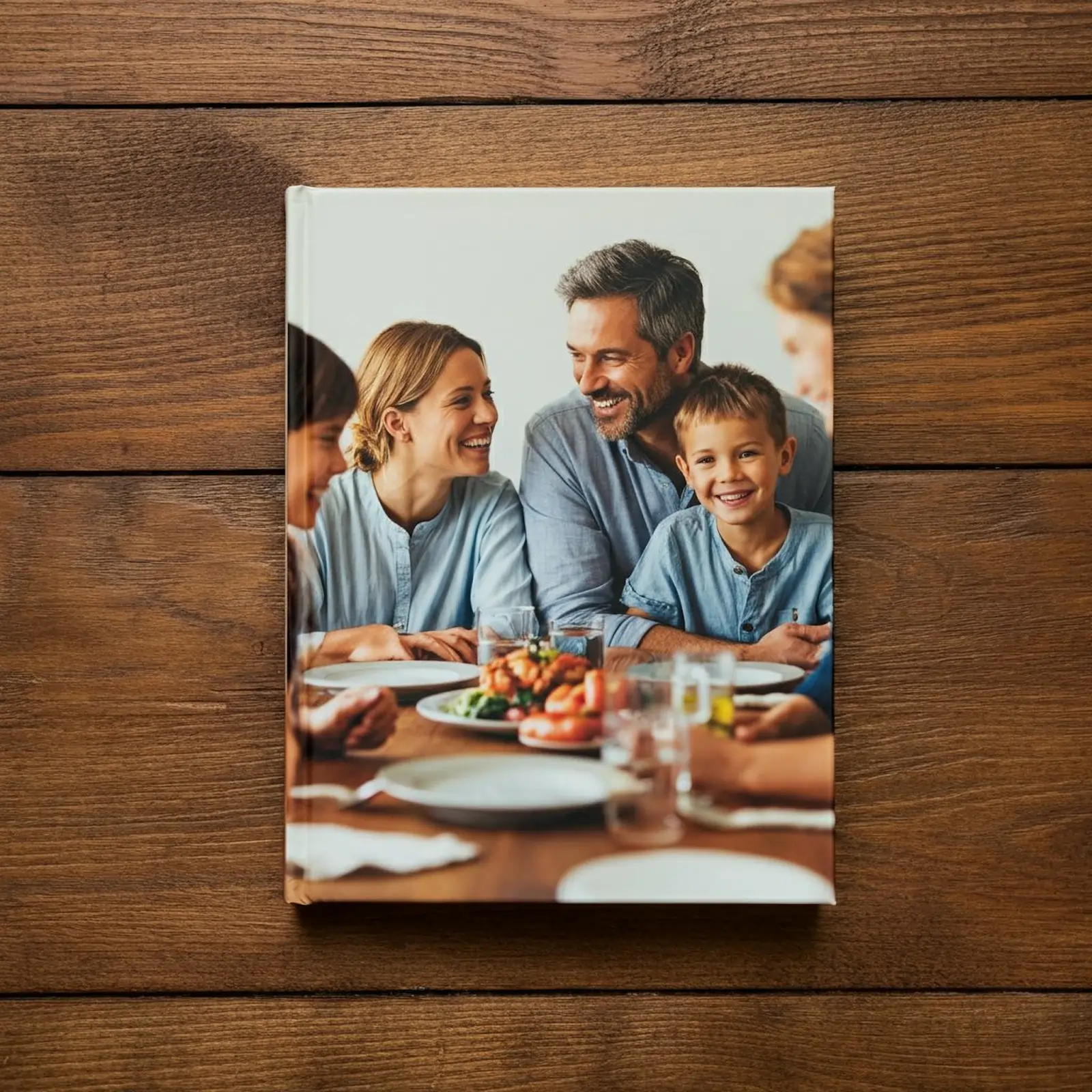
Textual content rendering
Imagine 3 enables innovative opportunities for rendering textual content within images. Experimenting with creative designs by generating images of posters, playing cards, and social media posts, incorporating varied font styles and vibrant color palettes, proves a highly effective method for exploring the capabilities of this software. To utilize this feature, simply provide a concise summary of your desired output in the space provided. What’s for Breakfast? A Morning Collection of Recipes
A photorealistic depiction of a cookbook situated on a wooden kitchen desk, with a quilt stretching across in front, featuring a warm and welcoming household seated at the same desk. Soft overhead lighting gently illuminates the scene, placing the cookbook at the visual forefront. A title is added to the center of the cookbook cover, emblazoned in bold orange letters: "Regular Recipes".
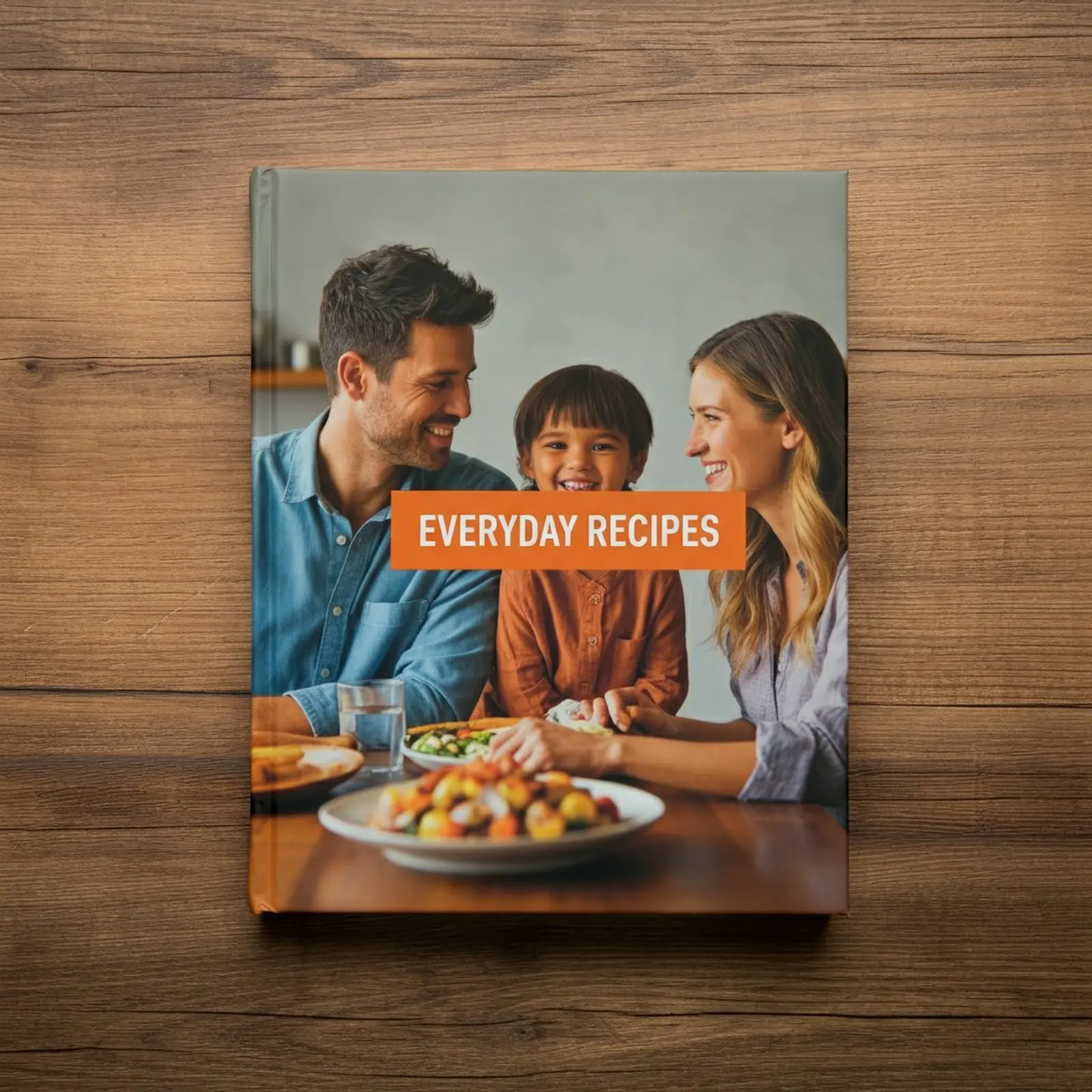
Decreased latency
DeepMind introduces Imagen 3 Quick, an optimised mannequin designed to accelerate technological advancements, alongside its flagship model Imagen 3, boasting unparalleled quality thus far. The Imagen 3 camera system is well-suited for capturing high-quality images with enhanced clarity and luminosity. You may notice a 40% reduction in latency compared to Image 2. Identical models are used to create two distinct photographs that showcase these unique styles. Let’s consider two alternative salad options that align with the cookbook’s theme: What about a “Summer Harvest Salad” featuring heirloom tomatoes, fresh mozzarella, basil, and a balsamic glaze? Alternatively, we could create a “Winter Greens Salad” with roasted butternut squash, crumbled goat cheese, toasted almonds, and a citrus vinaigrette?
generation_model_fast = ImageGenerationModel.from_pretrained("imagen-3.0-fast-generate-001") description = "A vibrant backyard salad overflows with an assortment of colourful greens – bell peppers, cucumbers, tomatoes, and leafy greens – nestled in a rustic wood bowl on a pristine white marble desk." Soft, serene light bathes the setting, delicately tracing the contours of each element and imbuing the atmosphere with a sense of freshness.
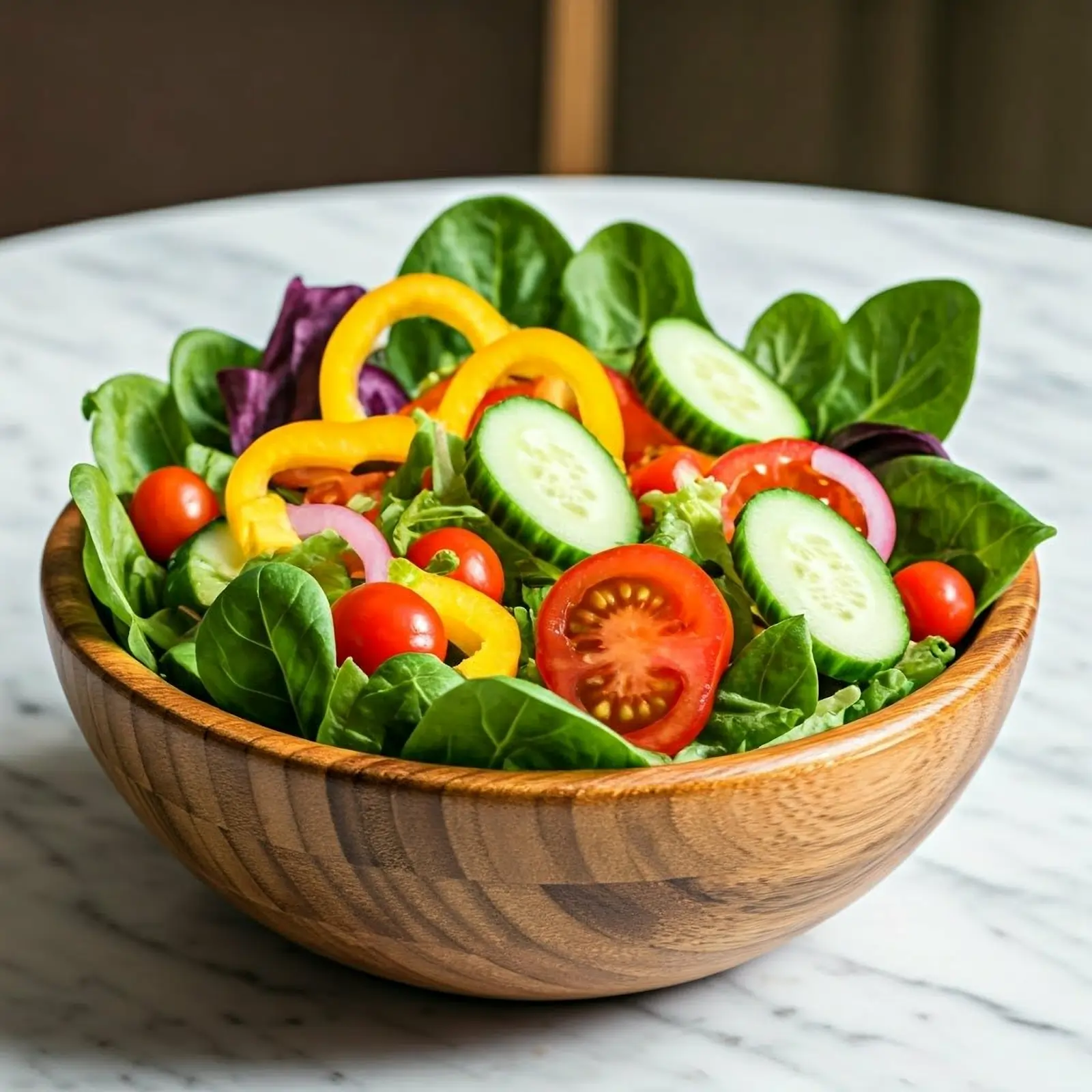
An alluring still life portrait presents a vibrant salad arrangement in a rustic wooden bowl atop a pristine white marble pedestal, amidst a backdrop of lush, verdant foliage. Soft, golden light envelops the setting, throwing delicate silhouettes and emphasizing the crispness of the surroundings. picture = generation_model.generate_images( immediate=True, number_of_images=1, aspect_ratio='1:1', safety_filter_level='block_some', person_generation='allow_all', )
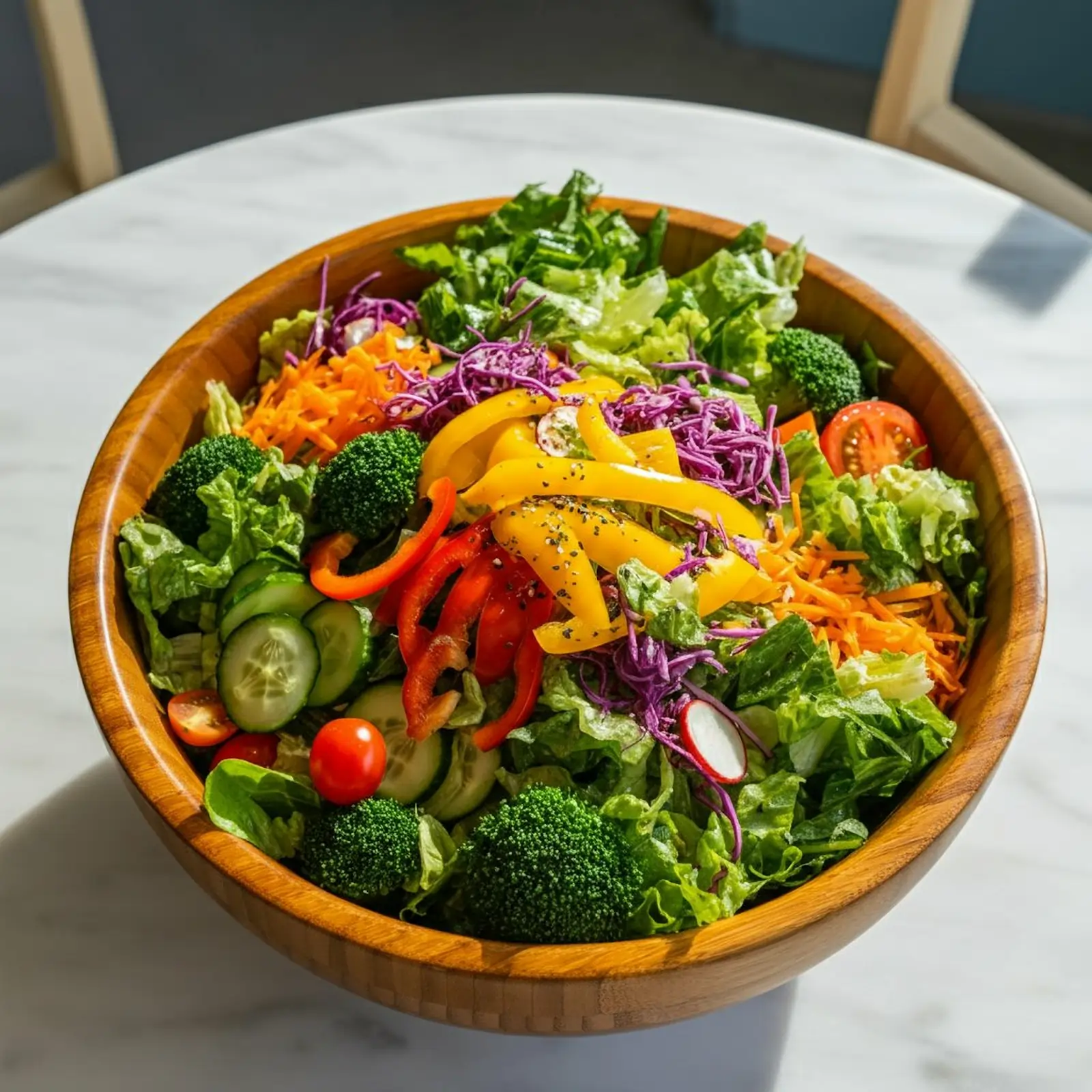
Utilizing Gemini
Utilizing the brand-new Imagene 3, we’re leveraging Gemini to access this innovative tool seamlessly. In the image below, we observe that Gemini is creating photographs using Imagen 3.
What if an iconic lion casually paces along metropolitan streets, its majestic mane rustling in the urban breeze, as skyscrapers and neon lights provide a striking backdrop for this unlikely stroll? Roadways accommodate various modes of transportation, including motorized vehicles, bicycles, and public buses. Can we ensure a genuine appearance?
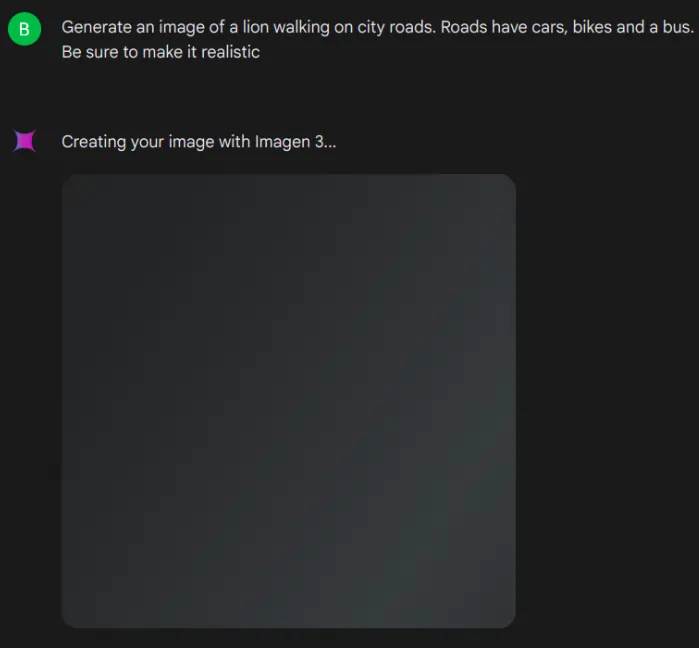
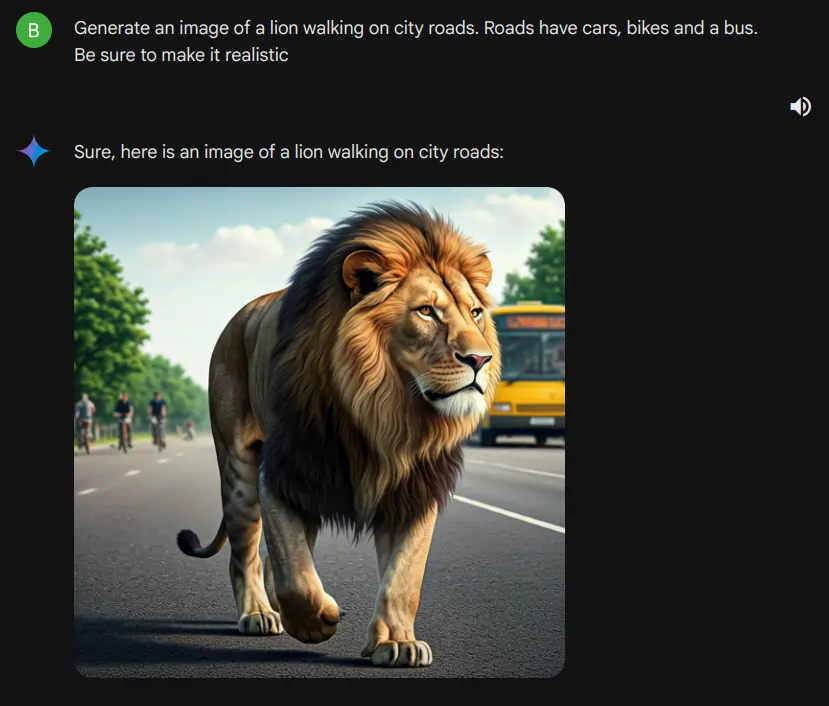
Conclusion
Google’s Imagen AI sets a new standard for text-to-image synthesis, surpassing expectations in photorealism while demonstrating exceptional accuracy in handling complex prompts. This model’s impressive performance across multiple analysis benchmarks showcases its exceptional abilities in precise prompt-image alignment and visual realism, outperforming leading models such as DALL·E 3 and Stable Diffusion, solidifying its position at the forefront of AI-generated imagery. Despite these efforts, the individual still encounters difficulties when tasks require strong numerical and spatial skills. The introduction of Imagen 3 Quick enables seamless collaboration between computers and creative minds, revolutionizing the realm of multimodal AI by significantly reducing latency and effortlessly integrating with a wide range of instruments?
Incessantly Requested Questions
Answer: Ans by Imagin 3 surpasses peers in photorealistic capabilities and instantaneous responsiveness, providing exceptional image quality and seamless alignment with user input, outperforming comparable models such as DALL-E 3 and Stable Diffusion.
Ans. The Imagen 3 model showcases exceptional proficiency in processing complex and lengthy input queries, consistently delivering accurate image representations that accurately reflect the nuances of the provided prompts.
Ans. The mannequin is trained on a vast, diverse dataset comprising textual content, images, and annotated data, carefully curated to eliminate AI-generated content, objectionable imagery, and low-quality information that may compromise its learning process.
Ans. Imagen 3 Quick boasts a significant 40% reduction in latency, ensuring seamless performance while maintaining exceptional image quality.
Ans.
Imagen 3 can seamlessly integrate with Google Cloud’s Vertex AI, enabling effortless collaboration between image technology and innovative tasks.