
What a remarkable week we’ve experienced so far at ! If you haven’t had the opportunity to explore our collective’s blog yet, we highly recommend browsing through our thought-provoking articles on and .
As we speak, we have just been informed by Dr. AWS CTO Swami Sivasubramanian’s Keynote Address at AWS re:Invent 2023. Dr. Sivasubramanian serves as Vice President of Knowledge and Artificial Intelligence at Amazon Web Services (AWS). With generative AI companies proliferating at an unprecedented rate, the time has never been riper for innovative breakthroughs and fresh service offerings to revolutionize the industry. What exciting developments are on the horizon for this year?
Over two centuries of cumulative technological advancements in mathematical computation, innovative architectures and algorithms, and emerging programming languages have collectively propelled our journey towards an inflection point in the development of generative artificial intelligence, Swami posited at the onset of his keynote address. He issued a challenge to reexamine the possibilities offered by generative AI in terms of intelligence enhancement. As humans collaborate with generative AI, fostering a harmonious synergy, we accelerate innovation and liberate our creative potential.
The impact of every bulletin from Immediately can be viewed through multiple lenses, shedding light on the intricate dynamics between information, generative AI, and humanity. To complete his vision, Swami outlined the essential prerequisites for building a generative AI tool:
- Access to an extensive array of diverse fashion styles.
- As you navigate through life, consider how your personal surroundings impact your ability to access and utilize information.
- Innovative tools for seamless function creation
- Goal-built ML infrastructure
Here is the rewritten text in a professional style:
This presentation summarized the key takeaways from Swami’s keynote address, including:
- Amazon SageMaker?
- Amazon introduces Titan multimodal embeddings, textual styles, and image generator capabilities within the Amazon Bedrock platform.
- Amazon SageMaker HyperPod
- What makes this search functionality truly innovative is its seamless integration with Amazon OpenSearch Serverless, allowing developers to build highly scalable and cost-effective applications.
- What companies are leveraging Amazon DocumentDB and Amazon MemoryDB for their NoSQL databases?
Amazon customers such as eBay, Airbnb, and Expedia use Amazon DocumentDB to power their e-commerce platforms, while others like Uber and Netflix rely on Amazon MemoryDB for their real-time analytics and personalized recommendations.
- Amazon Neptune Analytics
- Amazon OpenSearch Service seamlessly integrates with Amazon S3 to provide a scalable and cost-effective solution for ingesting and processing large volumes of data. With this integration, you can leverage the power of OpenSearch’s real-time search capabilities without having to write complex ETL (Extract, Transform, Load) scripts or manage additional infrastructure.
- AWS Clear Rooms ML
- Amazon Redshift’s latest advancements in artificial intelligence (AI) are revolutionizing data warehousing and business analytics. With the integration of machine learning algorithms, users can now seamlessly inject AI-driven insights into their business operations, empowering data-driven decision making like never before.
- What’s next for analytics on Amazon? Amazon QuickSight now supports generative SQL in Amazon Redshift.
- Amazon QLDB’s (Quantum Ledger Database) immutable ledger technology, seamlessly integrated with AWS Glue, enables you to create a tamper-evident and transparent audit trail for your data processing pipelines. This fusion of services empowers you to track the origin, validity, and modifications made to your datasets throughout their lifecycle within AWS Glue’s robust data integration capabilities.
- Mannequin Analysis on Amazon Bedrock
Let’s explore some of the latest trends and styles emerging on Amazon Bedrock today.
Anthropic Claude 2.1
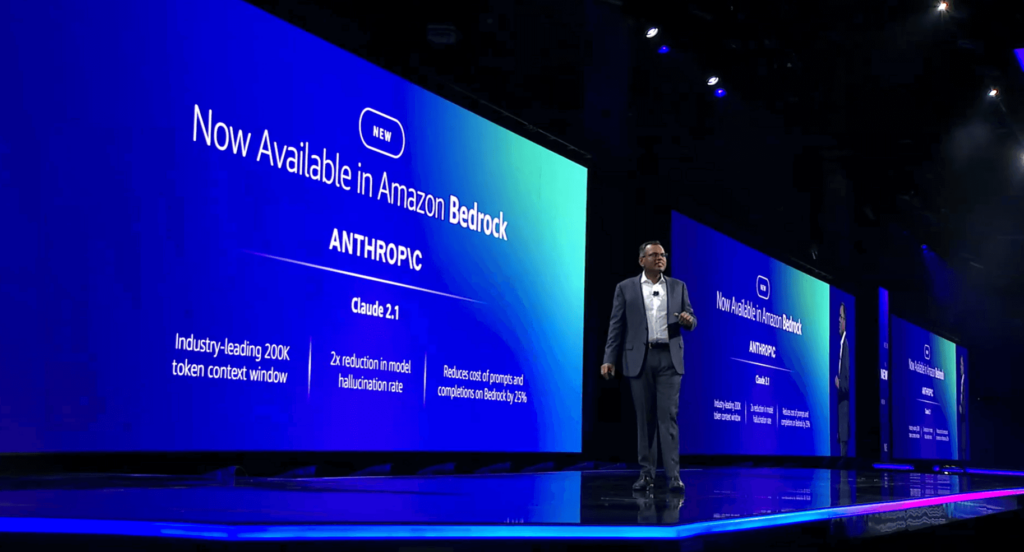
Recently, AI company Anthropic unveiled the latest iteration of its groundbreaking language model, Claude 2.1. Presently, this doll figurine is readily available within the Amazon marketplace. This latest iteration of Claude offers a range of crucial benefits compared to earlier versions, including:
- A 200,000 token context window
- A two-thirds reduction within the mannequin’s hypothetical valuation?
- Would you like to take advantage of a limited-time offer: get 25% off all Bedrock prompts and completions?
These advancements enhance the dependability and credibility of generative AI capabilities built upon the Bedrock foundation. Swami emphasizes the importance of accessing a diverse array of foundation models (FMs), noting that “no single model will dominate all others.” To this end, Bedrock provides support for a wide range of FMs, including Meta’s Llama-2 70B, which was also unveiled today.
Amazon’s Titan multimodal embeddings are now available in Amazon SageMaker Ground Truth, providing a powerful tool for developers to create custom AI models that can process multiple types of data simultaneously. This feature allows for the creation of more accurate and robust AI models by incorporating text, images, and other forms of data into a single framework.

Swami pioneered the concept of vector embeddings, which transform textual content into numerical representations. These embeddings play a pivotal role in refining and augmenting generative AI capabilities, particularly when tackling complex tasks such as multimodal search, where queries may comprise both textual inputs and uploaded visual or auditory content. To drive innovation, he unveiled Amazon Titan Multimodal Embeddings, a revolutionary technology that seamlessly integrates text, images, or their combination to deliver enhanced search, suggestion, and personalization capabilities within the realm of generative AI applications. To streamline complex family renovation projects, he showcased a practical tool utilizing multimodal search functionality. By integrating user-input text and image-based design preferences, the utility facilitates prospect discovery of necessary tools and assets required to complete a project.
The company also unveiled the global availability of Amazon Kindle Lite and Amazon Kindle Edition. While Titan Textual Content Lite excels at condensing information into concise summaries or crafting compelling copy, Titan Textual Content General shines in generating open-ended text and facilitating natural-sounding conversations. Titan also enables retrieval-augmented generation (RAG), a valuable feature for training your own fine-tuned models using your organization’s data.
He subsequently unveiled Titan Picture Generator, demonstrating its ability to create entirely new images from scratch and edit existing ones using solely natural language commands. Titan’s Picture Generator also promotes responsible AI utilization by covertly incorporating a unique identifier into every image produced, thereby explicitly acknowledging its AI-generated origin.
Amazon SageMaker HyperPod
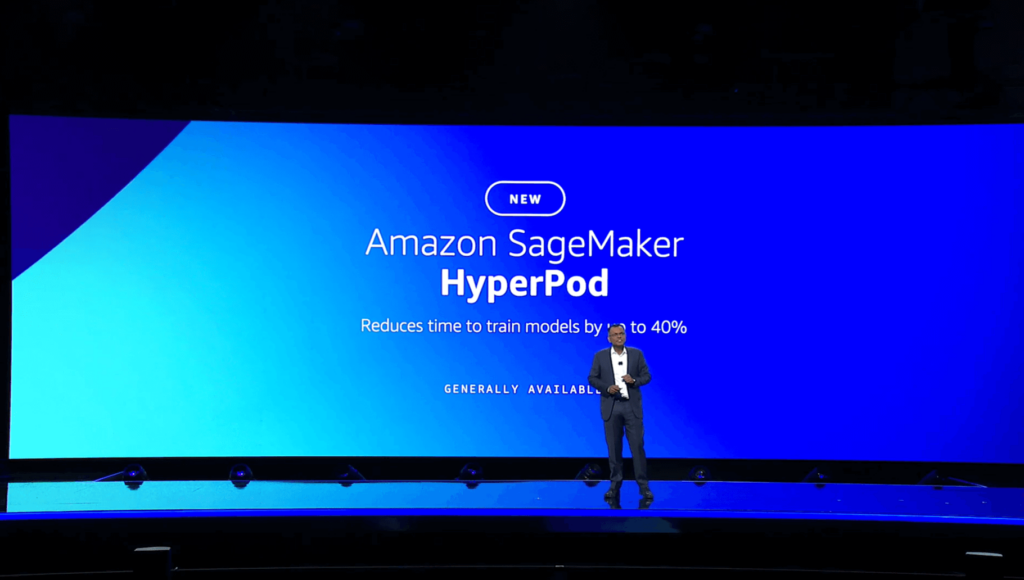
Organizations frequently encounter intricate issues when coaching their in-house facilities managers, prompting Swami to proceed with a discussion on this very topic. Data needs to be partitioned into manageable portions that can be distributed across nodes within a training cluster for effective processing. To mitigate against potential data loss in the event of a node failure, it is crucial to establish regular checkpoints within the process, thereby introducing a controlled delay that can help safeguard against such losses while still allowing for efficient use of resources. SageMaker HyperPod enables seamless coaching of Feature Managers (FMs) by isolating training data and models across resilient nodes, allowing for extended model training periods without disruptions. This results in a significant reduction in training time, potentially up to 40%, while optimizing use of cluster compute and network resources.
What’s driving your search requirements? With Vector, you can now harness the power of Amazon OpenSearch Serverless to fuel your business insights. By offloading computation and storage tasks to AWS, you’re free to focus on what matters most – delivering exceptional user experiences.
Skip
Returning to the topic of vectors, Swami emphasized the crucial requirement for a comprehensive information foundation that is both self-contained and governed, as a fundamental cornerstone in designing generative AI functions. To further enhance data utilization, AWS has developed a suite of companies based on your organization’s information foundation, which includes investments in integrated storage and retrieval of vectors and data collectively. By utilizing familiar tools, you avoid additional licensing and administrative burdens, provide a faster experience for end-users, and minimize the need for data migration and synchronization. AWS is heavily investing in enabling vector search capabilities across all its subsidiary companies. The primary announcement is the general availability of Amazon OpenSearch Serverless’ vector engine, which enables seamless storage and querying of embeddings alongside business data, facilitating more relevant similarity searches with a 20x increase in queries per second, without the need for maintaining a separate underlying vector database.
What organizations are looking to leverage Amazon DocumentDB (with MongoDB compatibility) and Amazon MemoryDB for Redis?
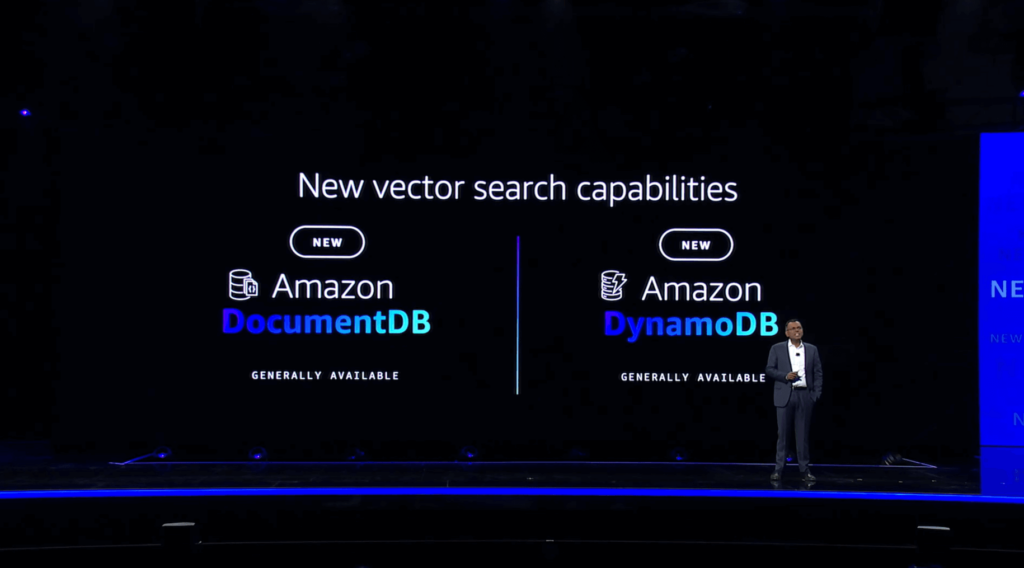
Amazon has further enhanced its offerings by introducing vector search capabilities in Amazon DocumentDB (compatible with MongoDB), as well as in Amazon MemoryDB for Redis, thereby expanding the existing vector search feature available in DynamoDB. These vector search options provide simultaneous support for both high throughput and high recall, boasting millisecond response times even under concurrency rates of tens of thousands of queries per second. This level of efficiency is crucial within functions that involve fraud detection or interactive chatbots, where even a slight delay can be costly.
Amazon Neptune Analytics
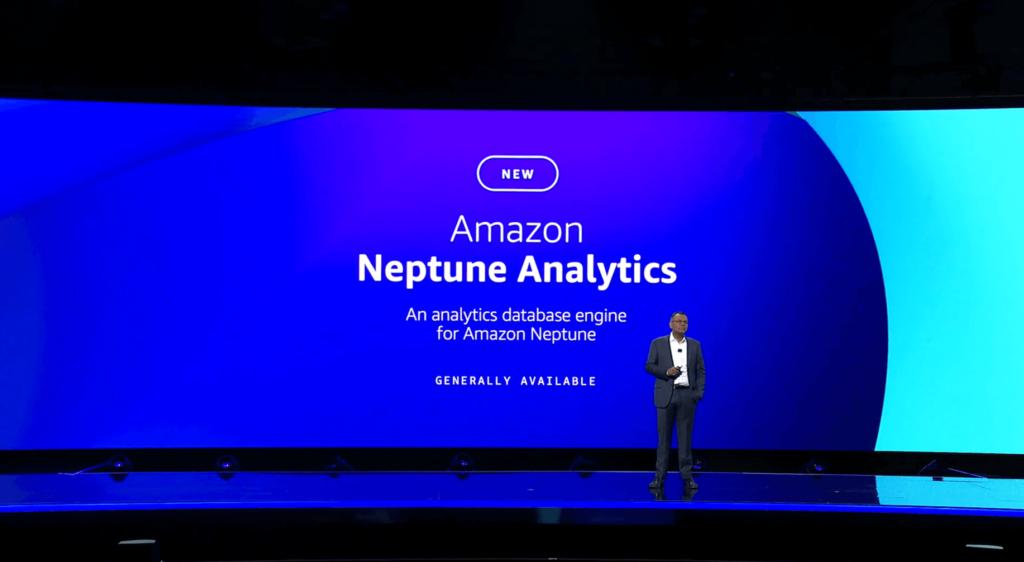
Within the realm of AWS database services, a recent announcement highlighted Amazon Neptune, a graph database solution empowering users to effectively model relationships and connections among data entities. Amazon Neptune’s analytics capabilities are now broadly available, empowering data scientists to swiftly analyze vast amounts of data stored within the graph database with unprecedented ease and speed. Neptune Analytics enables swift vector searches by storing both graph and vector data in a unified manner. Discover and unlock valuable insights within your graph data up to 80 times faster than current AWS capabilities, by processing tens of billions of connections in mere seconds through our integrated graph algorithms.
Amazon OpenSearch Service seamlessly integrates with Amazon S3, enabling real-time data ingestion and processing without the need for extract-transform-load (ETL) tools. With this seamless integration, you can efficiently ingest massive amounts of structured and unstructured data from various sources into your OpenSearch cluster, and then search, analyze, and visualize it to gain valuable insights. By leveraging Amazon S3’s scalability and reliability, you can store large datasets and retrieve them quickly for efficient querying and analytics.
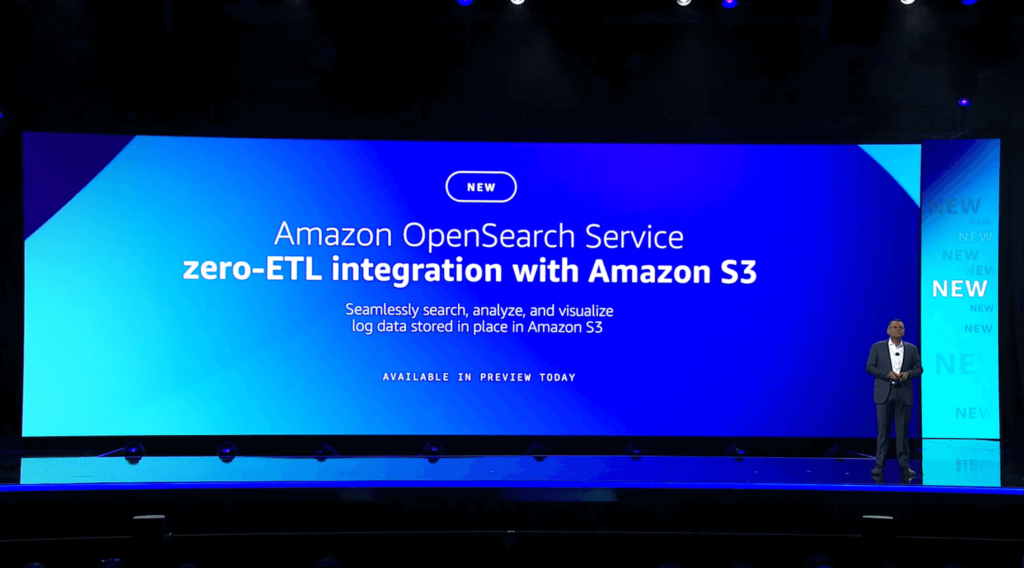
AWS is poised to revolutionize data management by offering seamless vector search capabilities across its database services, as announced by Swami. This move further solidifies the company’s commitment to a “zero-ETL” future, where businesses can thrive without the need for elaborate and expensive extract, transform, and load (ETL) pipeline development. This week, AWS announced the launch of multiple new zero-ETL integrations, including Amazon DynamoDB’s seamless integration with Amazon OpenSearch Service, as well as various zero-ETL connections with Amazon Redshift. At this moment, Swami announced yet another novel zero-ETL integration, specifically connecting Amazon OpenSearch Service with Amazon S3. Out there, our integration enables seamless exploration, analysis, and visualization of operational data stored in Amazon S3, similar to VPC Flow Logs and Elastic Load Balancer logs, as well as data lakes built on S3. You’ll also gain the flexibility to harness OpenSearch’s pre-built dashboards and visualizations, extending your analytics capabilities.
AWS Clear Rooms ML

AWS recently introduced the “debate” feature, allowing customers to collaborate safely with partners in “clear rooms” where they can work together without needing to share or replicate their original data. Currently, Amazon Web Services (AWS) has unveiled a preview release of AWS Clear Rooms ML, further expanding the concept of clear rooms to facilitate collaborative development of machine learning models through the use of managed lookalike models provided by AWS. With this feature, you can design unique styles tailored to your preferences and collaborate with others without sharing your raw data. AWS plans to introduce a healthcare-focused model within its Clear Rooms Machine Learning (ML) environment over the next few months.
Amazon Redshift now supports advanced AI capabilities that enable users to build machine learning models directly within the data warehouse. This integration allows for seamless blending of structured and unstructured data, unlocking new possibilities for predictive analytics and real-time insights. With this update, developers can leverage popular open-source libraries such as TensorFlow and PyTorch, while also benefiting from Redshift’s scalable architecture and optimized performance.
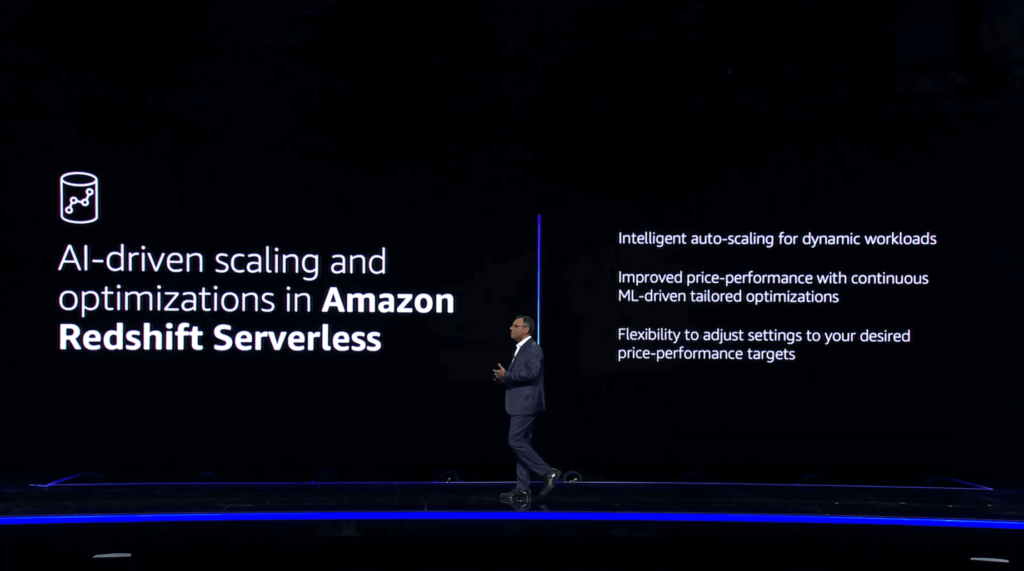
Two upcoming bulletins feature Amazon Redshift, beginning with AI-powered scaling and optimization enhancements in Amazon Redshift Serverless. These advancements feature intelligent autoscaling capabilities, empowering proactive scaling decisions based on nuanced usage patterns that incorporate query complexity, frequency, and data set sizes. By focusing on extracting valuable insights from your data rather than optimizing the performance of your data warehouse, you can achieve better results. By establishing price-performance targets, you can leverage machine learning-driven optimizations that dynamically adjust compute resources and modify database schema to optimize for value, efficiency, or a balance between the two, aligned with your specific needs.
What do you get when you combine Amazon’s scalability with SQL’s simplicity? The answer is Amazon Redshift, a fully-managed data warehouse that makes analyzing all your data as easy as running SQL queries.

The subsequent Redshift announcement is undoubtedly one of my personal standouts.
Immediately following yesterday’s bulletins on Amazon’s new generative AI-powered assistant, designed specifically for businesses with unique needs and data requirements, attention was drawn to Amazon Q, a generative SQL solution within Amazon Redshift.
Amazon’s latest innovation, generative SQL in Amazon Redshift, takes a significant leap forward by allowing users to write natural language queries directly against data stored in Redshift, much like Amazon Q’s “pure language to code” capabilities unveiled yesterday with Amazon Q Code Transformation. Amazon Q leverages contextual information about your database’s schema, as well as historical query patterns against it, to dynamically generate the optimal SQL queries aligned with your specific request. You can potentially configure Amazon QuickSight to utilize the query history of other customers within your AWS account when generating SQL queries, thereby streamlining your analytical workflow and minimizing manual data retrieval efforts. You too can pose queries about your data, akin to “what was the best-selling item in October” or “show me the top 5 rated items in our inventory,” without needing to grasp complex database structures, schema, or SQL syntax.
What are the key benefits of integrating Amazon QuickSight with AWS Glue for data processing and analytics?
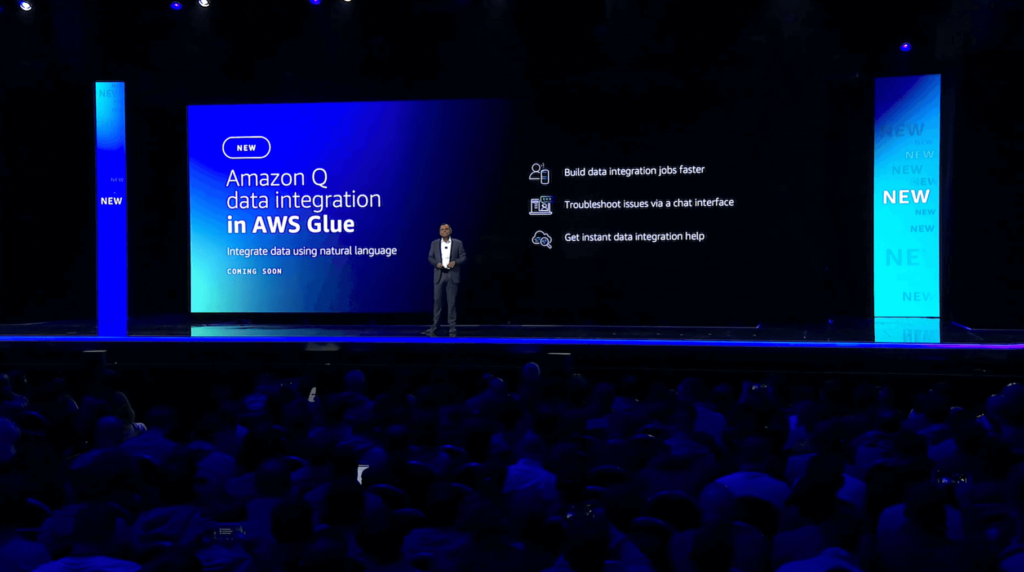
Amazon has announced a forthcoming enhancement to AWS Glue, focusing on the seamless integration of disparate data sets.
This compelling feature simplifies the process of building customised ETL pipelines where AWS lacks zero-ETL integration, enabling seamless integration with Amazon Brick by translating natural language commands into a series of actionable tasks using brokers.
You can also leverage Amazon’s capabilities by asking Alexa or Amazon SageMaker to write a Glue ETL job that reads data from S3, cleans the data by removing all null values, and then loads the information into Redshift.
Mannequin Analysis on Amazon Bedrock
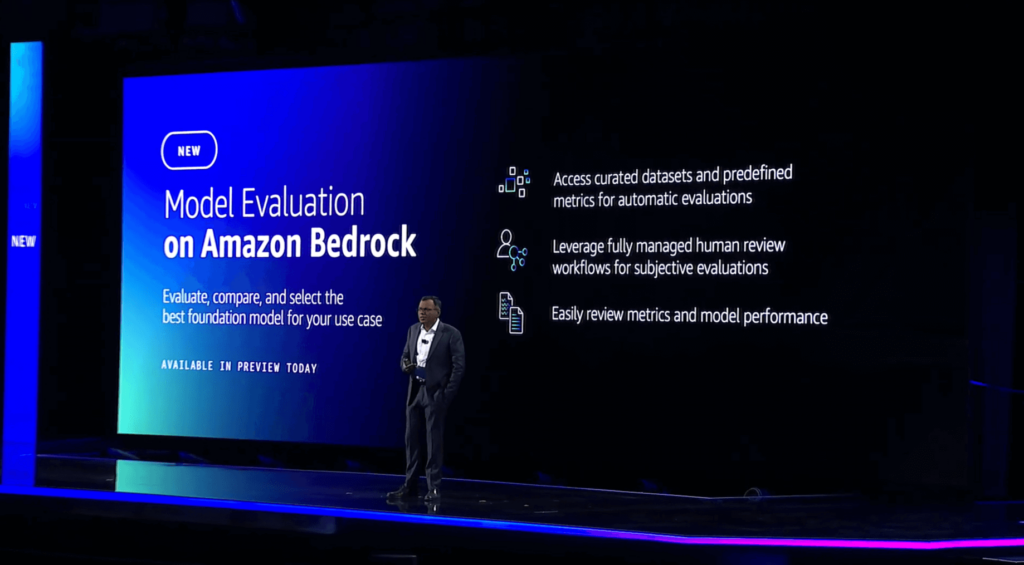
The Swami’s latest declaration reinforced the notion that numerous fashion models exist within Amazon Bedrock, and his previous statement that “no single model will dominate all.” Consequently, model evaluations are a crucial tool that should be conducted regularly by generative AI technology developers to ensure optimal performance. This instant preview launch of Mannequin Analysis on Amazon Bedrock enables you to examine, assess, and select the ideal Feature Model tailored to your specific use case. You may opt to utilize automated assessment based on criteria such as accuracy and toxicity, or human evaluation for matters like model tone and acceptable “model voice.” Upon job completion, Model Analysis will generate a comprehensive report featuring a summary of metrics highlighting the model’s performance.
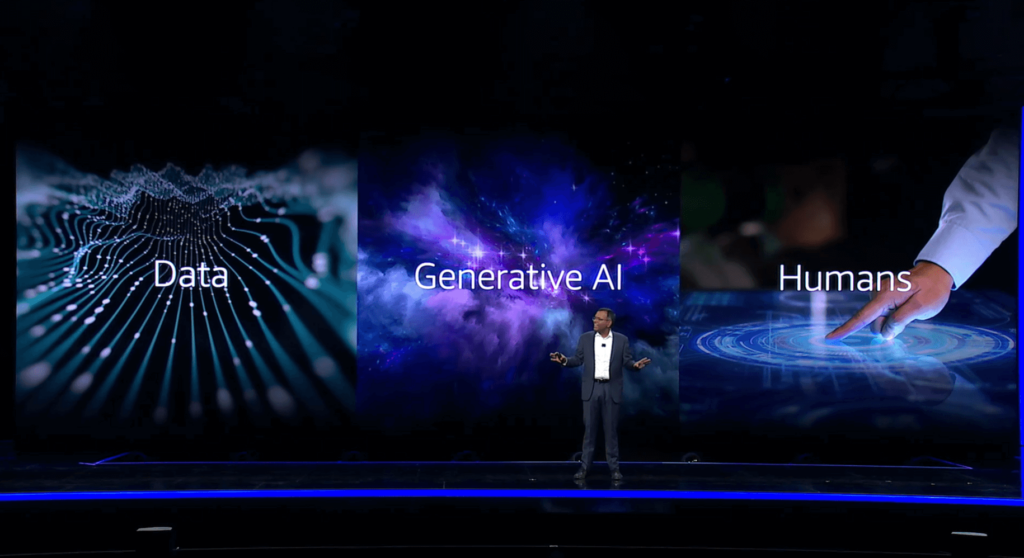
As Swami brought his keynote to a close, he redirected attention to the essential human element underlying generative AI, reiterating his conviction that these systems will significantly accelerate humanity’s productivity. Despite everything, individuals are responsible for providing critical inputs that enable generative AI applications to be informative and relevant. As synergies unfold between information, generative AI, and human interaction, a mutually reinforcing cycle emerges, fostering collective growth and resilience over the long term. As he wrapped up his thoughts, he emphasized that individuals can capitalize on the power of information and generative AI to fuel a self-sustaining cycle of achievement. As the generative AI revolution looms closer, human skills like creativity, ethics, and adaptability will become even more essential for driving success. According to a World Financial Discussion Board survey, nearly three-quarters of companies are expected to adopt generative AI by 2027. While generative AI may eliminate certain roles, it is likely that numerous new positions and opportunities will arise in the years to come.
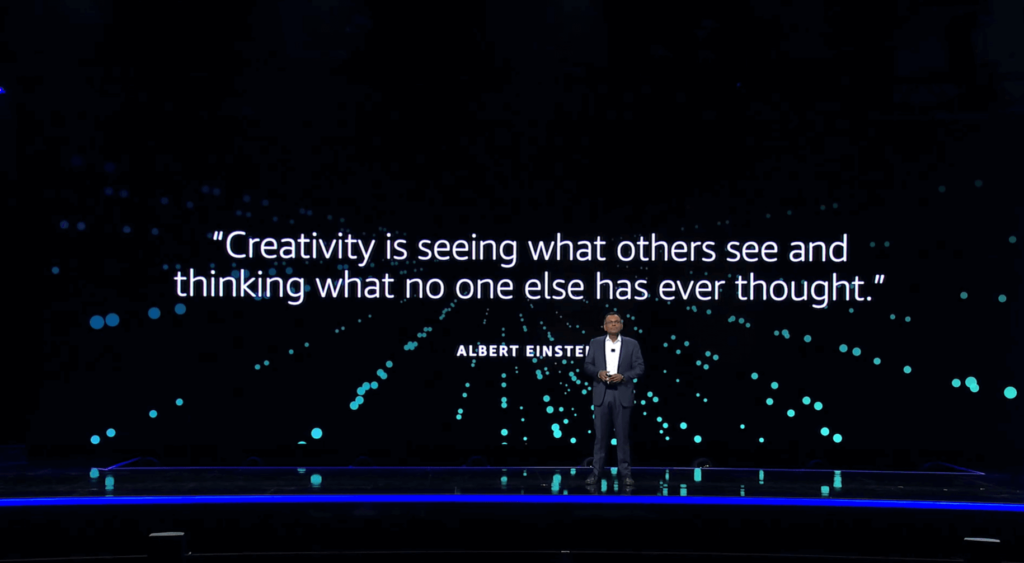
As I eagerly entered Swami’s keynote, brimming with excitement and anticipation, he once again exceeded my expectations by delivering an unforgettable experience. I’ve been thoroughly impressed by the extensive range and profoundness of bulletins and innovative feature rollouts thus far this week, and it’s only hump day! Stay tuned to this blog for more electrifying keynote updates from AWS re:Invent 2023!