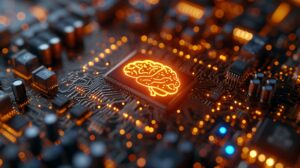
(Treecha/Shutterstock)
In the realm of information observability, Monte Carlo has established a notable presence by harnessing the power of machine learning and statistical techniques to uncover hidden patterns and ensure data quality and reliability within vast datasets. As part of this week’s replacement, made during its IMPACT 2024 event, the company is leveraging generative AI to enhance its data observability capabilities and propel them to new heights.
There is no silver bullet or machine learning model that can effectively detect every possible way information may go awry, as the nuances of observability require a multifaceted approach to ensure IT systems remain transparent and resilient. Engineers must grasp the vast array of potential pitfalls to anticipate and mitigate issues, laying the groundwork for automated information observability by conceptualizing their objectives.
Here’s the improved text:
The brand-new GenAI Monitor Suggestions, introduced just yesterday, have the potential to make a significant difference. The corporation leverages a large language model to scrutinize various uses of data within a customer’s database, subsequently suggesting specific screen designs or data quality standards for optimization.
Here’s how it functions: The Information Profiler module within the Monte Carlo platform ingests pattern data and feeds it into the Large Language Model (LLM) to scrutinize usage patterns in the database, with a focus on identifying intricate connections between column attributes. Utilizing a specific pattern alongside varied metadata, the Large Language Model constructs a nuanced comprehension of precise database usage within its context.
While classical machine learning models perform well in detecting anomalies such as data freshness and quantity metrics, Large Language Models (LLMs) excel at identifying patterns within the data that may be challenging or impossible to uncover using traditional machine learning approaches, notes Lior Gavish, Monte Carlo co-founder and Chief Technology Officer.
GenAI’s true strength resides in its ability to grasp semantics. For illustration, the system could potentially examine SQL query patterns to comprehend how fields are actually employed in manufacturing, thereby establishing logical connections between fields – such as ensuring that a ‘start_date’ is always earlier than an ‘end_date’. This semantic comprehension functionality surpasses traditional machine learning and deep learning capabilities.
The newly introduced functionality simplifies the creation of information quality guidelines, making it accessible to both technical and non-technical personnel. By leveraging an information analyst’s expertise with a professional baseball team, Monte Carlo expedited the development of guidelines for a “pitch_history” table. The data suggests a correlation between pitch type and pitch velocity, with distinct patterns emerging for fastball, curveball, and other pitches. With GenAI integrated, Monte Carlo can automatically propose data quality guidelines that logically follow from the historical context of the relationship between these two columns, thereby ensuring consistency and integrity across the dataset? The corporation claims that a “fastball” should exceed 80mph in terms of pitch speed.
By examining Monte Carlo’s instances, we uncover complex interdependencies hidden within data that traditional machine learning frameworks struggle to unravel. As LLMs leverage their capacity for human-like comprehension, they enable Monte Carlo methods to venture into previously inaccessible information connections, thereby yielding valuable insights into acceptable value ranges, ultimately driving meaningful returns.
Monte Carlo leverages Claude 3.5, a sophisticated sonnet/Haiku model running at a precise level of precision. To minimize hallucinations, the corporation implemented a hybrid approach where large language model (LLM) solutions were rigorously tested against real-world data samples before being rolled out to customers, he explains. The service offers complete configurability, allowing customers to opt-out at their discretion if needed.
Due to its remarkable ability to comprehend semantics and produce accurate responses, GenAI technology holds great promise to revolutionize various information management tasks that heavily rely on human intuition, including information quality administration and observability. Despite this, it has not always been crystal-clear how everything would ultimately come together. The Monte Carlo solution ensures that its information observability software can effectively verify the quality of data feeding into GenAI’s retrieval-augmented era workflows, thereby guaranteeing accurate and reliable insights. This week’s announcement underscores the corporation’s confidence in GenAI’s ability to seamlessly integrate with the information observability process.
“We seized an opportunity to harmoniously merge a genuine customer need with pioneering generative AI technology, enabling rapid construction, deployment, and operationalization of high-quality data guidelines that ultimately enhance the reliability of their most critical data and AI products,” Monte Carlo CEO and Co-founder Barr Moses said.
During the week, Monte Carlo showcased several upgrades to its information observability platform, which it had developed over time. To kick off its efforts, the organization introduced a cutting-edge Information Operations Dashboard, empowering customers to monitor and optimize their data quality projects with ease. The innovative Gavish dashboard presents a unified platform for monitoring diverse data insights, providing a seamless and comprehensive overview at your fingertips.
“The Information Operations Dashboard provides actionable insights to information groups on incidents occurring worldwide, detailing duration, persistence, and performance of incident owners in effectively managing their respective situations,” Gavish explains. By utilizing the dashboard, information leaders can effectively identify incident hotspots, track deviations from intended adoption courses, pinpoint underperforming team segments that require enhanced incident management capabilities, and uncover other opportunities for operational improvement.
Monte Carlo further strengthened its support for major cloud platforms, including AWS, Azure, and Google Cloud. As a result, corporations can identify issues with information pipelines running on various cloud platforms earlier than previously possible, gaining full transparency into pipeline failures, lineage, and efficiency operating on these cloud providers’ infrastructure, according to Gavish.
“As he notes, the intricate connections between these information pipelines can falter, triggering a catastrophic overflow of valuable insights.” “Information engineers struggle to manage alerts across multiple instruments, find it challenging to associate pipelines with the data tables they impact, and lack insight into how pipeline failures lead to data anomalies.” With Monte Carlo’s end-to-end information observability platform, organizations can now gain complete transparency into the interactions between each Azure Information Factory, Informatica, or Databricks workflow job and its downstream assets, including tables, dashboards, and reports.