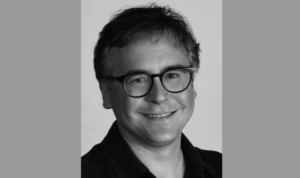
Luis Ceze is a multifaceted individual with numerous accomplishments: he serves as CEO and co-founder of OctoAI, holds the Lazowska Endowed Professorship at the University of Washington, co-founded the Apache TVM challenge, and has been recognized as a 2024 BigDATA Wire Individual to Watch.
Recently, we had the opportunity to reconnect with Ceze and pick his brain about his diverse pursuits. Here’s what he stated:
In January, you underwent a rebranding exercise, renaming your organization from OctoML to OctoAI? What specific aspect of the original text would you like me to clarify?
To better align with the expansion of our offerings, we rebranded our flagship product from OctoML to OctoAI, reflecting our enhanced capabilities in the rapidly evolving generative AI landscape that increasingly demands innovative solutions.
Over the past year, we have significantly enhanced our platform, empowering builders to develop and deploy manufacturing capabilities utilizing cutting-edge generative artificial intelligence models. Firms have the flexibility to select from a wide range of AI models, including off-the-shelf, customised, and open-source options, and deploy them either on-premise within their own environments or in the cloud.
Our latest innovation, OctoStack, is a comprehensive, end-to-end manufacturing platform that enables large-scale enterprises to seamlessly integrate high-performance inference, model customization, and asset management capabilities. This grants firms complete AI autonomy for building and operating generative AI capabilities within their proprietary environments directly.
Several prominent generative AI startups, including Apate.ai, Otherside AI, Latitude Games, and Capitol AI, leverage our platform to effortlessly integrate our robust, customizable, and eco-friendly infrastructure into their own ecosystem, boasting numerous high-growth prospects. These companies are ultimately accountable for their approaches to fashioning and profiting from our low-maintenance server stacks.
As co-founder of the Apache TVM initiative, we pioneered a platform allowing machine learning models to be optimized and compiled for various hardware architectures. GPUs have become incredibly popular lately. Shouldn’t we consider being more receptive to applying machine learning models across various hardware platforms?
Czech: In the past 18 months, we have witnessed an unprecedented surge in AI innovation. As the technology has evolved over time, AI has transitioned from being a theoretical concept in labs to a tangible force driving business success. To enable the widespread adoption of AI, it’s crucial to develop the technology to operate seamlessly across a diverse range of platforms, from cloud-based data centers to edge devices and mobile phones.
As we reach a turning point reminiscent of the early cloud era. Firms require the flexibility to deploy data across various cloud platforms or hybrid models that combine cloud and on-premise infrastructure.
Firms require seamless integration of accessibility and selection when building with AI capabilities. The system should accommodate any model, whether custom-made, proprietary, or open-source. Developing seamless access to run stated fashions freely across any cloud or native endpoint without constraints.
Our original objective with Apache TVM was to achieve this goal, a pursuit that I continued through my affiliation with OctoAI. Developed on the principle of hardware independence and portability, OctoAI SaaS and OctoStack enable seamless deployment in a wide range of customer settings, unaffected by underlying infrastructure.
The transition from experimental stages to widespread deployment of GenAI is anticipated to take place between 2023 and 2024, marking a significant milestone in its development lifecycle. Companies leveraging Large Language Models (LLMs) must prioritize effective integration, strategic deployment, and continuous monitoring to unlock tangible business value.
Ceze: By our estimates, 2024 marks a pivotal year for generative AI, as we anticipate a shift from exponential growth to mainstream adoption and industrial-scale deployment. To successfully achieve their objectives, companies must focus on several crucial aspects.
While the primary cost is indeed a controlling factor, it’s essential to note that the unit economics of large language models (LLMs) can still be influenced by various variables, making it crucial to carefully consider these factors when evaluating their potential value. While mannequin coaching is a predictable expense, unexpected increases in utilization can quickly escalate costs, potentially exceeding initial projections.
Choosing the right mannequin for your specific use case? As the market becomes increasingly saturated with over 80,000 language models and counting, the initial excitement and novelty surrounding large language models (LLMs) start to wear off, culminating in a sense of mannequin fatigue as users struggle to discern meaningful differences between the numerous options available. Finding an approach that harmoniously balances high performance with efficiency and cost-effectiveness is the delicate equilibrium required to achieve optimal results.
Thirdly, fine-tuning is crucial to tailor large language models for specific applications and optimize their performance. As LLMs become increasingly commoditized, their value lies in customization, which caters to specific, high-priority use cases.
Beyond my professional realm, I’m an avid nature photographer, capturing serene landscapes and vibrant wildlife. What might surprise my colleagues is that I’m a passionate beekeeper, with a thriving apiary in my backyard where I harvest honey for local bakeries. When the weather permits, you’ll find me kayaking through tranquil lakes or attempting to break my personal record at the local disc golf course.
Eating is my pleasure; a delightful experience. I enjoy studying meals, preparing dinner, and savoring the experience of eating.
I’m intrigued by meals that seamlessly merge disparate culinary traditions, with a deep dive into their cultural roots and even the chemical reactions that occur during cooking. After approximately 30 minutes of consuming.
Another highlight: I recently contributed to DNA-based data storage research and even had my work travel to the moon, a remarkable achievement.
You’ll have the opportunity to gain insights on the most influential individuals in the 2024 Big Data industry, as featured in the Big DATA Wire’s annual “Folks to Watch” list.