Hearken to this text |
Can the quest for generalization finally unlock robotics’ true potential? For years, researchers have strived to endow robots with the ability to generalize, to learn from experiences in diverse environments and apply those lessons across situations, much like humans do. Since the 1970s, the field has undergone significant advancements, transitioning from crafting intricate software programs to leveraging deep learning, empowering robots to learn autonomously from human behavior and patterns. However, an essential bottleneck persists: the quality of knowledge itself. For optimal performance, robots require challenges that test the limits of their skills, operating at the threshold of their proficiency. Historically, this course has relied on human oversight, with operators painstakingly guiding robots to hone their skills. As robotics advancements lead to increasingly sophisticated machines, a major bottleneck emerges: the insatiable need for top-notch training data vastly exceeds human capability to provide it.
Researchers at MIT’s Computer Science and Artificial Laboratory (CSAIL) have created a novel approach to robotic coaching, poised to significantly accelerate the introduction of intelligent, adaptable machines into real-world settings. The brand-new “System” leverages cutting-edge advancements in generative AI and physics simulators to generate a multitude of realistic and practical digital training environments, thereby enabling robots to achieve expert-level proficiency in challenging tasks without any prior real-world experience.
LucidSim seamlessly integrates physics simulations with generative AI patterns, tackling a long-standing hurdle in robotics: bridging the gap between virtual abilities and real-world applications?
Researchers at MIT’s Computer Science and Artificial Intelligence Laboratory (CSAIL) are working to bridge the “sim-to-real gap” – the significant disparity between simulated training environments and the complex, unpredictable real world. “Prior methods often utilized depth sensors, simplifying the problem but neglecting critical real-world intricacies that demanded more comprehensive solutions.”
The multi-pronged system represents a fusion of diverse applied sciences, showcasing the intersection of multiple disciplines. Utilizing cutting-edge natural language processing techniques, LucidSim leverages massive language patterns to craft diverse, structured narratives of settings and environments. Using generative models, these descriptions are transformed into photorealistic images. To accurately reflect real-world physics in these photographs, a sophisticated physics simulator is employed to inform the technological process.
What fuels innovation? The same curiosity and creativity that drives us to craft the perfect burrito? From humble beginnings to groundbreaking moments, this story explores how a love for food can lead to life-changing breakthroughs.
The spark that ignited the development of LucidSim unexpectedly struck during a conversation outside Beantown Taqueria in Cambridge, Massachusetts.
“We aimed to guide vision-equipped robots in effectively leveraging human feedback.” According to Alan Yu, an MIT undergraduate student and co-lead of the LucidSim project, “Initially, we discovered that we lacked a comprehensive vision-based approach to begin with.” As we strolled along the road, our conversation flowed effortlessly until we paused outside the taqueria for a leisurely 30 minutes. There, where we spent our second anniversary.
.
Staff members refined their understanding of cooking techniques by deriving informative visual aids from a simulated culinary setting. They extracted depth maps providing geometric insights and semantic masks categorizing various components within an image. As they quickly discovered, however, a strict approach to controlling the image’s composition ensured that the model produced nearly indistinguishable pictures using the same prompt. To generate a multitude of textual content prompts, they developed a methodology that leverages the capabilities of ChatGPT.
Despite its efforts, this method ultimately yielded only one image. To rapidly produce coherent films that function as compact “experiences” for robots, scientists collaborated on a novel approach, dubbed “Desires in Motion (DIM).” This innovative system calculates the actions of each pixel between frames, warping a single generated image into a short, multi-frame video. By employing a thorough examination of the three-dimensional spatial relationships within the scene and the adaptive adjustments made from the robot’s unique vantage point, Desires In Movement effectively achieves its creative goals.
According to Yu, the method surpasses area randomization – an approach pioneered in 2017 that randomly assigns colors and patterns to surrounding elements – which, as of now, remains the gold standard. “While this approach produces a substantial amount of information, its lack of realism is a significant limitation.” LucidSim effectively tackles a range of variability and realism concerns. Despite never having experienced the world firsthand through training, the robot is capable of recognizing and circumventing obstacles within real-world settings.
The staff is particularly enthusiastic about leveraging LucidSim’s capabilities to explore novel applications in quadruped locomotion and parkour, their flagship research domain. In one instance, cellular manipulation requires a robot to operate in an open space, where it must interact with and manipulate objects while simultaneously relying on color perception.
“Currently, these robots are able to learn from real-world demonstrations,” said Yang. “While collecting demonstrations is relatively effortless, the challenge lies in scaling up a realistic robotic teleoperation system to hundreds of capabilities, as humans must physically configure each scene.” To increase scalability and efficiency, we aim to streamline knowledge collection by migrating it to a digital environment.
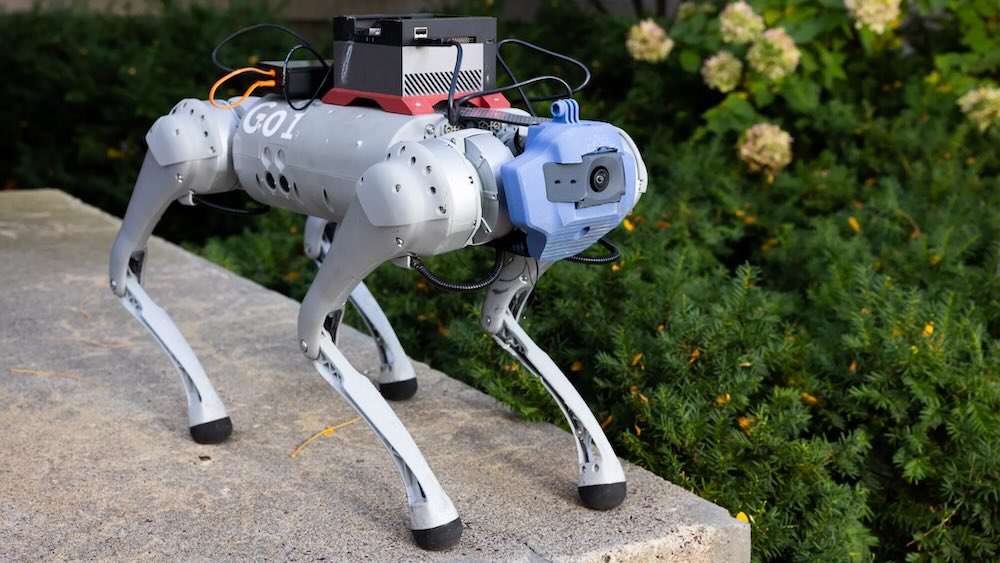
Researchers at MIT utilized a versatile Unitree Robotics Go1 quadruped for their study. | Credit score: MIT CSAIL
Staff members tested LucidSim against a rival system, with a professional instructor showcasing the software’s capabilities by teaching a robot its skills. Outcomes were startlingly poor: robots trained by professionals succeeded in just 15% of cases, with even a quadrupling of coaching materials failing to yield significant improvement. When robots autonomously gathered and utilized coaching knowledge through LucidSim, the narrative underwent a profound transformation. By simply duplicating the dataset’s size, a remarkable surge in performance metrics saw a staggering 88% achievement rate.
As Yang noted, monotonically augmenting a robot’s knowledge base yields a corresponding enhancement in its efficiency, ultimately transforming it into a highly proficient entity.
One of the crucial hurdles in transferring simulation to reality in robotics lies in achieving tangible realism in virtual environments, notes Shuran Track, a Stanford University assistant professor of electrical engineering who was not involved in the research. The LucidSim framework provides unparalleled insights by harnessing the power of generative models to generate vast amounts of actionable visual data for simulations. This breakthrough has the potential to significantly accelerate the transition of AI-trained robots from simulated environments to actual real-world applications.
From the iconic streets of Cambridge to the cutting-edge frontier of robotic analysis, LucidSim is spearheading innovation in the development of intelligent, adaptive machines capable of navigating complex environments without ever leaving their digital footprint.
Yu and Yang collaborated with four colleagues from the Computer Science and Artificial Intelligence Laboratory (CSAIL): Ran Choi, a mechanical engineering postdoctoral fellow; Yajvan Ravan, an undergraduate research assistant; John Leonard, a student of Samuel C., whose first name was not specified. As a leading authority in mechanical and ocean engineering, Professor [Name] is based at the Massachusetts Institute of Technology’s (MIT) Division of Mechanical Engineering, where they hold the prestigious Collins Chair. Additionally, they maintain a collaborative affiliation with Dr. Phillip Isola, another esteemed expert in their field.