For the reasons the launch of ChatGPT in November 2022 sparked, the generation of artificial intelligence (GenAI) has taken a significant leap forward.
Panorama has undergone rapid cycles of experimentation, improvement, and refinement.
Adoption occurs across a wide range of application scenarios. Utilized to the software program
Engineering businesses leverage GenAI assistants to expedite software development by having them co-author code with human engineers.
The IDE’s advanced code completion capabilities allow developers to work more efficiently by providing them with a list of suggested code fragments that they can quickly insert into their code.
When the developer begins typing a function or method name, the IDE will offer a list of possible completions based on the relevant code elements within the project.
Original text: What’s the most important thing for you in a relationship? Is it trust, respect, honesty, communication, emotional support, or something else?
Improved text: What do you consider essential in a romantic partnership? Does trust, mutual respect, and open communication take precedence, or is it perhaps emotional validation, shared values, or a sense of adventure that holds the most significance for you? The strategy is employed globally.
producing and testing code. While acknowledging the substantial promise held within.
Utilizing GenAI for forward-looking engineering, we also recognize the myriad benefits of incorporating artificial intelligence into our development processes.
Faced with the daunting challenge of navigating the intricacies of outdated methodologies.
The reality is that developers often devote far more time to grasping the intricacies of programming languages than actually crafting new code.
By leveraging and revitalizing numerous legacy approaches to better serve our customers, we’ve found that adopting an evolutionary approach proves most effective.
Legacy displacement excels in achieving its worthwhile objectives with enhanced safety and simplicity. This methodology not only reduces the
While modernizing key enterprise techniques poses risks, it also enables the creation of value early on and facilitates frequent integration.
Suggestions for progressive development are provided by incrementally releasing new software throughout the process. Despite the encouraging developments that have unfolded recently
From this strategy over a ‘ Huge Bang’ cutover, the price-time-worth equation for modernizing large-scale technologies typically involves
prohibitive. Can GenAI turn this situation around?
Over the past 18 months, our team has been actively exploring and refining.
Large language models to effectively address the complexities and hurdles surrounding their development.
modernization of legacy techniques. Over the course of this period, we have successfully established three
generations of CodeConcise, an inside modernization
accelerator at Thoughtworks . The motivation for
Constructing CodeConcise emerged from a fundamental declaration: the imperative for modernizing.
Challenges faced by our customers share similarities. Our purpose is for this
Key accelerator to transform us into a cohesive unit?
Legacy modernization enables us to enhance the value of our modernized streams.
Enable us to more fully understand the benefits we can offer our customers.
We aim to leverage our expertise in utilizing GenAI for modernization initiatives. Whereas a lot of the
The team at CodeConcise has honed their skills through extensive hands-on experience.
with it. We do not suggest that CodeConcise or its approach is the sole means to leverage GenAI effectively for
modernization. As experimentation unfolds with CodeConcise and diverse instruments, we
We will gladly share our valuable insights and key takeaways with the local community.
Artificial Intelligence Milestones: A Chronology of Notable Events
One main cause for the
The present wave of euphoria and delight surrounding GenAI is the culmination of years of tireless innovation.
The versatility and unwavering efficiency of general-purpose large language models (LLMs), enabling seamless adaptation to diverse applications. Every new era of fashion has consistently evolved.
Established advancements in natural language processing, including improved comprehension, inference, and response capabilities.
high quality. Organizations are increasingly utilizing these remarkably potent
Fashion trends emerge to cater to individuals’ unique preferences and desires. Moreover, the introduction of
Multimodal AIs, akin to text-to-image generative models such as DALL-E, alongside
With AI-powered fashion technologies capable of advanced video and audio comprehension and era-specific styling?
Has significantly expanded the applicability of Genuinely Intelligent Assistants. Furthermore, the
The newest AI fashions are capable of retrieving new information from real-time sources.
Past what’s included in the existing datasets, there exists a vast scope for further expansion and diversification.
their scope and utility.
Since their inception, we’ve observed a proliferation of innovative software products that have revolutionized the way we work and live.
with GenAI at their core. In various cases, contemporary merchandise have evolved
Powered by GenAI, new possibilities are now available through the integration of previously inaccessible features. These
Merchandising often leverages the fundamental capabilities of Large Language Models (LLMs), but these straightforward applications can swiftly reach their limitations as the use case evolves beyond.
Please provide the text you’d like me to improve. I’ll return the revised text in a different style as a professional editor.
transformations). As a professional editor, I would revise the original text as follows:
Entry your group’s information; the most economically viable solution typically emerges.
involves the implementation of a Retrieval-Augmented Generation (RAG) approach.
Alternatively, one could consider fine-tuning a general-purpose language model alongside RAG to achieve better results.
particularly when designing a model that needs to address complex rules within a specialized
In certain cases where area-specific regulations demand precise control.
mannequin’s outputs.
The proliferation of AI-generated goods will be driven in part
Attributable to the provision of multiple instruments and expansion?
frameworks. These tools have democratized General Artificial Intelligence (GenAI), providing accessible abstractions.
What AI-driven insights will empower teams to streamline their operations and unlock new efficiencies through seamless integration of large language models?
Quickly conduct innovative tests within simulated scenarios without needing expertise in artificial intelligence?
experience. Despite the progress made, caution is still warranted in these fledgling stages?
Days mustn’t become an excuse to succumb to the comforts of familiar frameworks, lest they constrain our growth.
Thoughtworks’ .
Issues that make modernization costly
Following our decision to leverage “GenAI for Modernization”,
centred on the inevitable challenges that would repeatedly confront us – namely
We had known that those driving modernization were concerned with neither time nor value.
prohibitive.
- What insights can we gain into the underlying architecture by examining the prevailing implementation details of a system?
- What aesthetic appeal does this product hold, allowing users to intuitively grasp its functionality through a harmonious blend of form and function?
- Data on this topic could be collected through various automated methods such as web scraping, social media analytics tools, and natural language processing algorithms that can identify and extract relevant information from unstructured data sources. Additionally, machine learning models can be trained to analyze large datasets and identify patterns and trends without the need for human intervention.
to information us? - Here’s an attempt at rephrasing: Can we leverage idiomatic translation tools to seamlessly migrate code at a large scale to our target technology stack?
stack? How? - As societies adapt to the rapid pace of technological advancements, it’s crucial to strike a balance between progress and preservation. By fostering interdisciplinary collaborations, we can develop innovative solutions that mitigate the risks associated with modernization.
Integrating traditional knowledge with cutting-edge technology enables us to create more resilient and sustainable systems. This holistic approach can help reduce the environmental impact of industrial-scale production, promote eco-friendly infrastructure development, and foster a culture of responsible innovation.
Moreover, inclusive policies and community engagement strategies are vital in addressing the social and economic disparities that often accompany modernization. By empowering marginalized groups and providing accessible education and training programs, we can create a more equitable distribution of resources and opportunities.
Ultimately, embracing a comprehensive and participatory approach to modernization is key to minimizing its dangers and maximizing its benefits.
Automated checks serve as a robust security net. - Here are the extracted domains, subdomains, and IP addresses.
capabilities? - To enhance user protection, we must implement more robust safeguards to accommodate diverse behavioral patterns.
Between outdated techniques and new ones, are clear and intentional distinctions drawn? How will we allow
Can cut-overs be executed with minimal disruption and maximum efficiency?
What kind of relevance do some of these queries have to a comprehensive modernization?
effort. Now we have deliberately focused our attention on addressing the root causes of our problems.
difficult modernization situations: Mainframes. These are among the
Many of the most crucial legacy technologies we come across, measured by their impact on our lives.
complexity. If we resolve these questions surrounding this situation, then there is a possibility that our decisions will have significant implications for the future.
Will undoubtedly yield fruitful results for diverse skill sets.
The Structure of CodeConcise
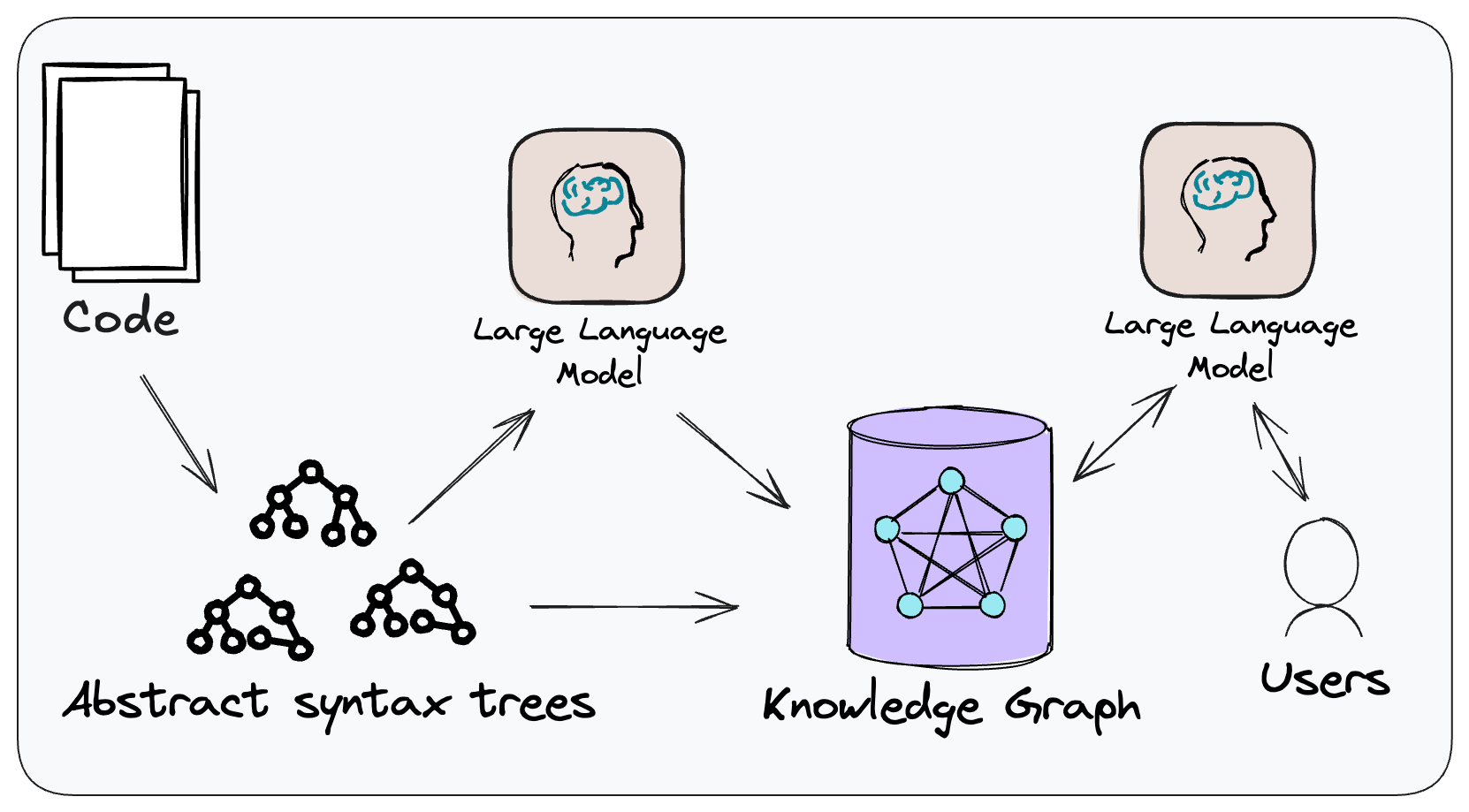
The overarching vision of CodeConcise lies in crafting a seamless synergy between innovative problem-solving and efficiency-driven coding practices.
CodeConcise is impressed by the innovative vibe of this place where coders code.
processed and examined through methodologies traditionally employed for data. This implies
We’re not treating code as just textual content, but rather harnessing the power of language to make it more readable and maintainable.
Particular parsers are examined to identify their inherent structure, and a correlation is drawn.
Code’s entity relationships. That achievement stems from parsing the raw data into a meaningful format.
Code trees within a forest of Abstract Syntax Trees (ASTs), that are then
saved in a graph database.

Determining a Reliable Ingestion Pipeline in CodeConcise?
Nodes are connected by establishing edges that convey specific information. For example, an edge might indicate
The transfer of control occurs from one node’s code to another. This course of
doesn’t allow us to comprehend how a single file in the codebase might connect with others.
To varying degrees, we also extract at a much more granular level, for instance, which specific
Conditional dependencies of specific departments within a single file are transferred seamlessly to the corresponding code sections.
different file. The ability to navigate the codebase with unparalleled precision and nuance.
Significantly mitigates background interference, thereby allowing for more accurate analysis and detection of meaningful patterns. pointless code) from the
context supplied to LLMs, particularly related for information that don’t comprise
extremely cohesive code. The following two benefits we have observed from this.
noise discount. The large language model (LLM) is particularly susceptible to keeping focused on the present moment.
Within a sustainable framework, we apply the restricted housing concept within the context of a carefully considered windowed environment.
Should consolidate additional details into a concise summary immediately. Successfully, this permits the
Can LLMs search through code without being limited by its organization?
the primary place by builders. We analyze our approach to ensure a seamless integration with the ingestion pipeline.
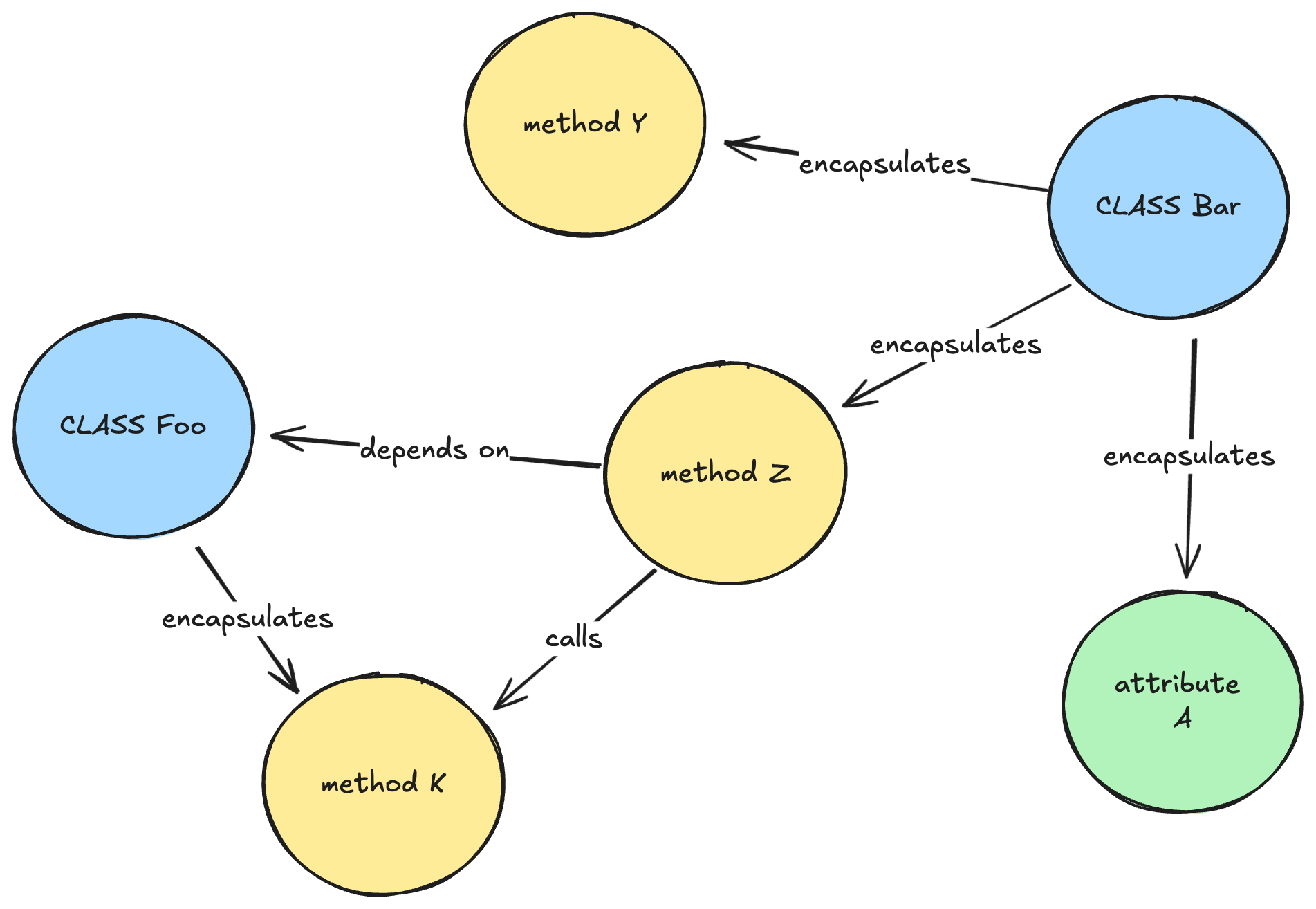
Determining dependencies within a Java codebase? A visual representation might resemble a complex network with nodes and edges connecting various components, illustrating their relationships.
Following this, a comprehension pipeline navigates the graph through multiple steps.
algorithms corresponding precisely to this post-order traversal.
Traversal to concurrently counterpoint graphs with Large Language Model (LLM)-generated explanations at diverse depths.
(e.g. strategies, lessons, packages). While certain methods employed at this juncture are
Frequently encountered in legacy technology infrastructures, we have further developed innovative prompts within our
Comprehensive comprehension pipelines tailored specifically for unique languages and frameworks. As we started
Utilizing concise coding practices with real-world customer code, our team recognized the need to
maintain the comprehension pipeline extensible. That streamlined data effectively
data most valuable to our customers, taking into account their unique regional perspectives.
For example, at one shop, we discovered that a query to a specific database
Desk-embedded AI code can potentially facilitate enhanced comprehension for Enterprise Analysts when implemented correctly.
defined using our customers’ industry-specific vocabulary. That is notably related
When there isn’t a common understanding between people?
technical and enterprise groups. While the enriched data graph serves as the cornerstone
The product of the comprehension pipeline isn’t a single, treasured outcome. Some
Enrichments generated throughout the pipeline, mirroring those created by robots.
Documentation concerning the system are invaluable in their own right. When supplied
On customers, these enrichments can provide targeted enhancements that either complement existing knowledge or fill gaps in their understanding.
techniques documentation, if one exists.
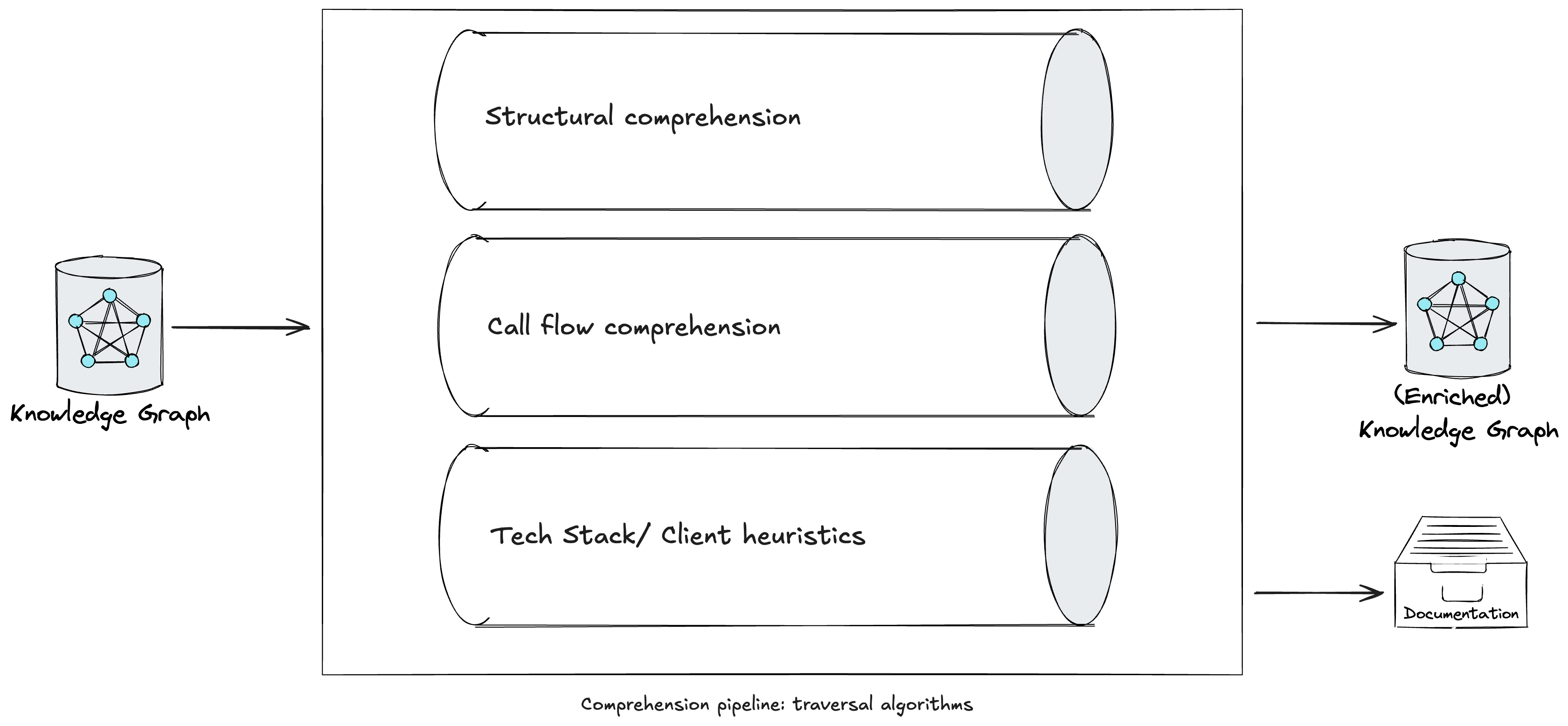
The concise comprehension of code pipeline determines whether a certain level of clarity is achieved by condensing the code into a more streamlined and efficient format.
Neo4j, a leading graph database, stores its enriched Data Graph.
This DBMS’s advanced vector search capabilities enable us to seamlessly integrate the
Implementing a data graph in the frontend software to visualize Red-Amber-Green (RAG) indicators in real-time, allowing users to seamlessly track and analyze key performance metrics. This strategy
leveraging the graph’s construction, offers the LLM a significantly richer contextual understanding.
Enabling the model to explore adjacent nodes and generate elucidations through Language Model-driven insights.
at varied ranges of abstraction. Data acquisition capabilities are optimised through the utilisation of diverse phrases in the Retrieval Application Gateway (RAG), thereby enhancing the overall efficacy of the system.
pulls nodes related to the consumer’s immediate needs, while the large language model (LLM) additionally traverses the entire network.
graphs to aggregate additional information from their adjacent nodes. As an illustration,
How does authorization work in API design?
Does the card information display correctly when viewing card details? The index should consistently show relevant results only.
Ensure thorough validation of consumer roles explicitly, implementing robust code to safeguard against potential issues?
Notwithstanding the presence of behavioral and structural edges within the graph, we will further examine
Embracing related information within established strategies, the encircling framework of code.
Within the data structures
The context to this Large Language Model (LLM), thus potentially disrupting a more comprehensive response. The next is an instance
of a richly detailed data visualization framework?
the enriched data’s output nodes, where inexperienced blue nodes signify the results of various enrichment processes.
comprehension pipeline.
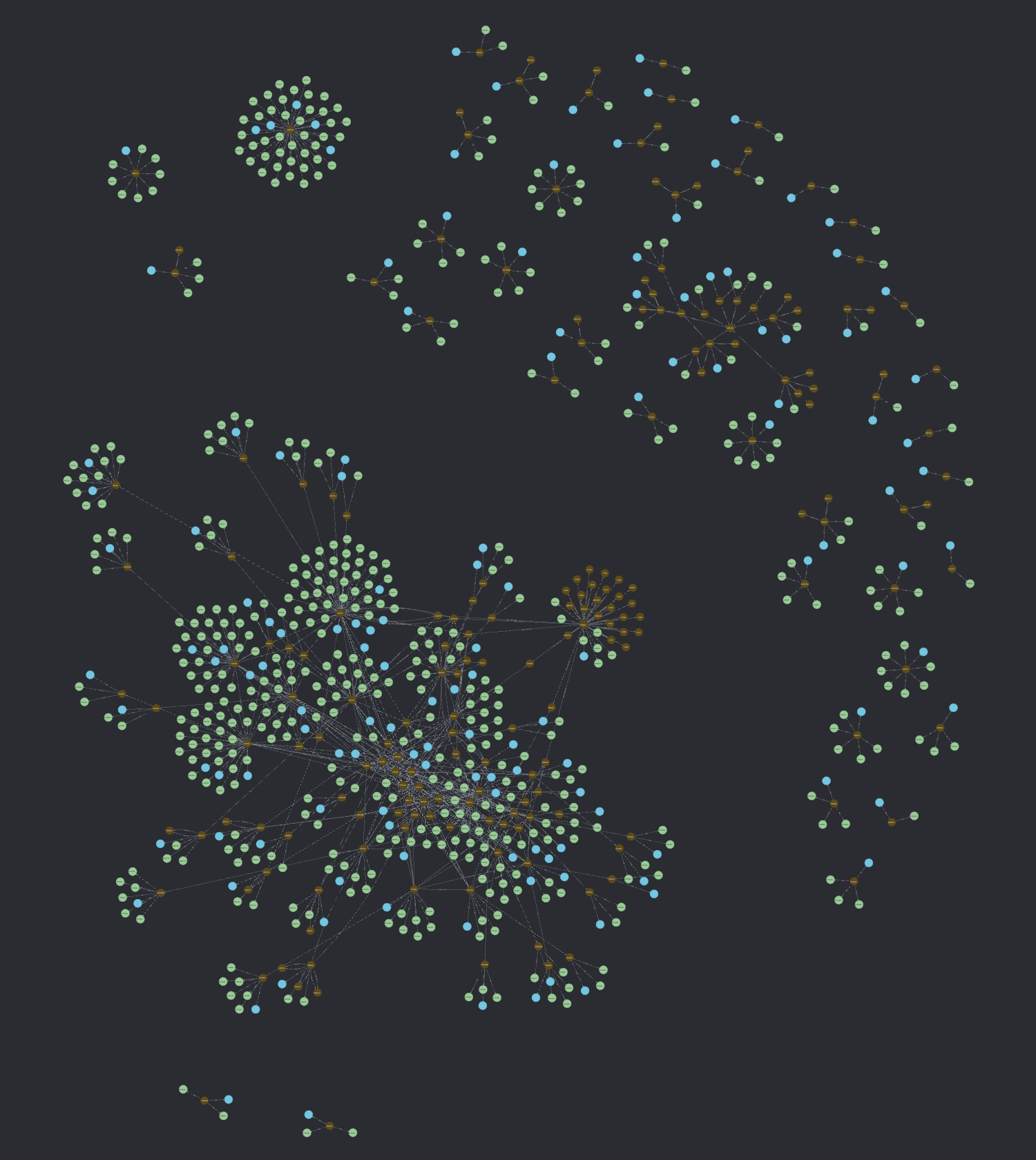
Determine 05: Data Visualization – Enriching Insights with AWS Card Demo
What remains crucially linked to this analysis is the capacity for supplementary traversal of the graph, whose significance lies in its potential to reveal novel patterns and correlations hitherto unnoticed?
Ultimately depends on the factors employed to construct and refine the network within
first place. There is no one-size-fits-all solution to this challenge; its success hinges on
The precise context, the insights one seeks to extract from their code, and, in turn,
The teams’ ultimate strategies were shaped by the guidelines and methodologies they chose to employ.
When establishing the foundation of your solution’s infrastructure. As an illustration, heavy use of multimedia elements, such as videos, images, and interactive diagrams, can effectively convey complex information to learners, while also fostering engagement and motivation.
inheriting constructors that would potentially necessitate additional attention to INHERITS_FROM
edges vs
COMPOSED_OF
Edges of a codebase that prioritizes composability.
What are the key features of the CodeConcise model that enable developers to accelerate their coding workflow?
Through iterative development, our team experienced a transformative journey.
Will soon publish another article: Code comprehension experiments with machine learning algorithms to improve software development.
LLMs.
We examine specific aspects of modernization in greater detail.
Challenges that, should they be resolved through the application of GenAI, could potentially have a substantial impact on pricing.
Worthwhile investments and a willingness to adapt are essential factors in determining whether to embark on a digital transformation, as they typically precede any reluctance to modernize – components that often deter us from making significant changes.
the choice to modernize now. As we venture further inward, we are now commencing internal explorations.
How GenAI will potentially tackle obstacles moving forward as we’ve merely begun to explore its capabilities.
experiment with alongside our purchasers. The places where our writing truly shines?
The extra speculative analyses have been meticulously highlighted to facilitate easy identification.