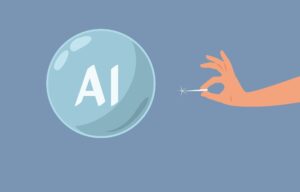
(Nicoleta Ionescu/Shutterstock)
Uncertainty is beginning to permeate conversations surrounding generative artificial intelligence (GenAI), with trade experts increasingly voicing skepticism about whether the substantial investments made in this technology will ultimately yield a return on investment. A lack of a “killer app” alongside coding co-pilots and chatbots poses the most pressing challenge, according to a recent Goldman Sachs analysis letter, which also highlights the hurdles presented by limited knowledge availability, chip shortages, and energy concerns. Despite initial doubts about the short-term viability of GenAI, many remain optimistic about its long-term potential to transform enterprises and society.
The sheer frenzy surrounding GenAI over the past year caught the attention of seasoned tech journalists, particularly those who witnessed the dot-com bubble and subsequent crash in the early 2000s, as well as the rapid growth of cloud computing and smartphones following the introduction of the iPhone in 2006 and 2007.
The unprecedented surge in knowledge during the early 2010s culminated in the widespread adoption of big data technologies, notably the ascendance of Hadoop as a symbol of Silicon Valley’s relentless pursuit of innovation and technological advancement. As the Hadoop ecosystem slowed in its growth, a significant shift ensued with the rise of massive knowledge advertising and marketing efforts fueled by the emergence of AI as the new driving force. Several innovative solutions made strong bids for recognition, with Blockchain taking center stage along the way, poised to make a significant impact. 5G will computing! Self-driving vehicles are almost a reality. Is the dawn of a new era in artificial intelligence – yet, despite the hype, little tangible progress was made, and the vast knowledge landscape gradually refined conventional machine learning approaches while remaining skeptical about the practical value of those novel neural networks.
In late 2022, a groundbreaking new large-language model, dubbed ChatGPT, was unleashed upon the world. The fervor surrounding neural networks’ AI capabilities and transformer networks’ generative AI has unsettlingly echoed earlier pivotal moments in technological history. It’s price noting that a few of these monumental moments proved to be inflection points, such as those in cellular and cloud technology, while others left us wondering “What were we thinking?” regarding innovations like blockchain and 5G. In contrast, it took years for the full implications of other technological breakthroughs to become apparent, as witnessed by the dot-com bubble’s impact.
Which classes do we anticipate GenAI being placed within five years from now? Goldman Sachs joins a chorus of experts cautioning that AI may ultimately suffer the same fate as 5G and blockchain, two technologies that failed to live up to their lofty expectations.
From the latest edition of Goldman Sachs’ Analysis Publication, titled “Will Gen AI’s Excessive Spending Translate into Profit?”, editor Allison Nathan raises a thought-provoking question: can AI deliver on its promises?
“The promise of generative AI expertise holds vast potential for transforming businesses, industries, and societies alike, as major tech giants, various firms, and utilities are expected to invest approximately $1 trillion in capital expenditures over the next few years, with significant allocations dedicated to building knowledge facilities, cutting-edge chips, advanced AI infrastructure, and upgrading the grid.” “Despite this expenditure, there is currently no conclusive evidence that it will yield the desired outcomes, as past experiences have yet to demonstrate a significant impact on building effectiveness.”
According to MIT Professor Daron Acemoglu, Nathan interviewed him and learned that only a quarter of the tasks intended for automation by AI are likely to be successfully automated in an economic sense. According to Acemoglu’s estimate, automation is expected to replace only approximately 5% of total duties within the next decade, resulting in a negligible productivity boost of less than 1% for the US economy over this period?
Generative AI holds the promise of revolutionizing the very fabric of scientific discovery, transforming the way we analyze, innovate, test new products and materials, and more. To further expand opportunities, Acemoglu suggested that Nathan explore the development of fresh product lines and innovative channels. “While the primary focus and architecture of generative AI expertise are currently set, significant transformative changes won’t materialize quickly, with potentially none occurring within the next decade.”
While accelerating GenAI’s progress by intensifying production of its foundational elements—knowledge and graphics processing units (GPUs)—may seem like a viable strategy to some, esteemed economist Daron Acemoglu suggests that it may not be enough to drive meaningful advancements, as the quality of knowledge remains a crucial factor.
“With the incorporation of twice as much knowledge from Reddit into the GPT model, its ability to predict the next phrase in an impromptu conversation may be significantly enhanced,” he said, “but it’s unlikely to directly improve a customer support consultant’s capacity to assist a customer troubleshoot issues with their video service.”
While there may be a shortage of suitable chips for training and deploying generative AI fashion models, this concern might also be seen as a reasonable factor contributing to Goldman’s skepticism towards the technology. Nvidia’s fortunes have skyrocketed as a result, with revenues surging more than 260% to $26 billion in the quarter ending April 28. The company’s market value surged past $3 trillion, earning it membership in an elite group and solidifying its position among the world’s most valuable companies.
At present, NVIDIA stands alone in its capacity to manufacture GPUs that power AI capabilities, according to Jim Covello, Goldman’s head of global fairness research, who noted this insight in a recent newsletter. While some speculate that internal adversaries in the semiconductor industry or even hyperscalers like Amazon and Microsoft may arise as opponents to Nvidia, a scenario that’s certainly plausible. While it’s a significant step forward considering chip companies haven’t been able to topple Nvidia from its supreme GPU position in the past decade.
The hefty costs associated with implementing and utilizing GenAI pose significant obstacles to achieving productivity or efficiency gains that this technology could ultimately deliver, according to Covello.
“AI has demonstrated significant potential in streamlining existing processes, such as coding, by enhancing efficiency, although estimated gains have diminished; however, the cost of utilizing AI to tackle tasks remains significantly higher than current methods.”
As Covello worked as a semiconductor analyst during the initial launch of smartphones, he gained profound insights into the intricacies required to grasp the fiscal benefits derived from technological advancements. The smartphone manufacturers pledged to integrate global positioning systems (GPS) into their devices, and indeed, they had a clear vision in place that ultimately proved insightful.
“No equivalent road map currently exists for the development of AI,” he noted. AI bulls seem to firmly believe that the proliferation of use cases will accelerate as expertise grows and evolves. Despite the excitement surrounding generative AI’s debut, a full year and a half later, no groundbreaking software has emerged that can boast both significant impact and cost-effectiveness.
Ultimately, the amount of energy necessary to train large language models and other generative AI architectures must be taken into consideration in our calculations. Estimated to account for merely 0.5 percent of global energy consumption currently, AI’s appetite for power is poised to increase significantly over time.
“According to Brian Janous, co-founder of Cloverleaf Infrastructure and former vice president of energy at Microsoft, utilities are inundated with requests for significant amounts of energy as the AI sector expands, but ultimately only a small percentage of that demand will materialize.”
According to Janous, the capacity of energy projects prepared to integrate with the grid increased by nearly 30 percent over the past year, while wait times currently range from 40 to 70 months. As energy demands surge and knowledge facilities seek additional power to fuel AI training, simple goals become a reality.
The US faces a pressing need to upgrade its grid infrastructure in response to growing energy demands, but implementing such changes will not come cheaply or easily, according to experts. “The United States has unfortunately lost its ability to undertake massive infrastructure projects—a task better suited to 1930s America than 2030s America,” said Janous. That leaves me with a sense of cautious uncertainty.
While some may predict a bleak outlook for AI’s prospects, not all share this gloomy forecast. Joseph Briggs, a senior world economist at Goldman, is an enthusiastic proponent of generative artificial intelligence (GenAI). According to his article refuting Acemoglu’s views, Briggs projects that GenAI will ultimately automate approximately one-quarter of all job tasks, thereby boosting U.S. productivity by 9 percent and cumulative GDP growth by 6.1 percent over the ensuing decade. As AI’s capabilities expand, GenAI won’t simply displace existing jobs but rather catalyze the emergence of novel responsibilities, according to its leader.
“The potential for full AI-driven automation to uncover duties that can occur over an extended period may yield significant cost savings, potentially amounting to several hundred dollars per employee annually,” he stated. As technological advancements accelerate, the cost of newly applied sciences typically declines rapidly over time. While cost-saving applications of generative AI may initially follow a similar pattern, the marginal value of deployment is likely to dwindle once purposes are established, leading us to expect AI adoption and automation costs to surpass the estimated 4.6% suggested by Acemoglu in the end.
Kash Rangan, another prominent advocate for General Artificial Intelligence (GenAI), shares the vision of a future where AI surpasses human intelligence in all aspects. During his interview with Goldman editor Nathan, the senior fair value analysis analyst expressed astonishment at the pace of innovation at GenAI and admiration for the rapid build-out of cloud infrastructure by major players. While acknowledging GenAI’s lack of a defining application, he noted that it may eventually achieve widespread adoption similar to how ERP systems prevailed in the 1990s, followed by search and e-commerce in the 2000s and cloud computing in the 2010s.
“Not surprisingly, though, this approach shouldn’t come as a shock, considering the typical sequence of development in computing: infrastructure is prioritized initially, followed by platforms, with applications ultimately taking center stage.” “While significant progress is being made in infrastructure development, the AI cycle still lingers in this phase. While it may require additional time to find the breakthrough software, I’m confident that we’ll ultimately arrive at a solution.”
Eric Sheridan, his colleague, aligned with him in a confident and optimistic outlook.
The expertise still has a long way to go in its development. At industry gatherings or developer conferences, witnessing firsthand the astounding feats of generative AI’s capabilities is a guarantee to leave attendees energized and optimistic about its boundless possibilities for the future.
“While I wouldn’t deny my concerns about the possibility of no return on investment, I’m not overly worried at present, though that could change if we don’t see significant client growth within the next 12 to 18 months,” Sheridan said.
Will the promise of GenAI remain extravagant at the end of the day? Will GenAI’s returns surge before time runs out? The clock is ticking.