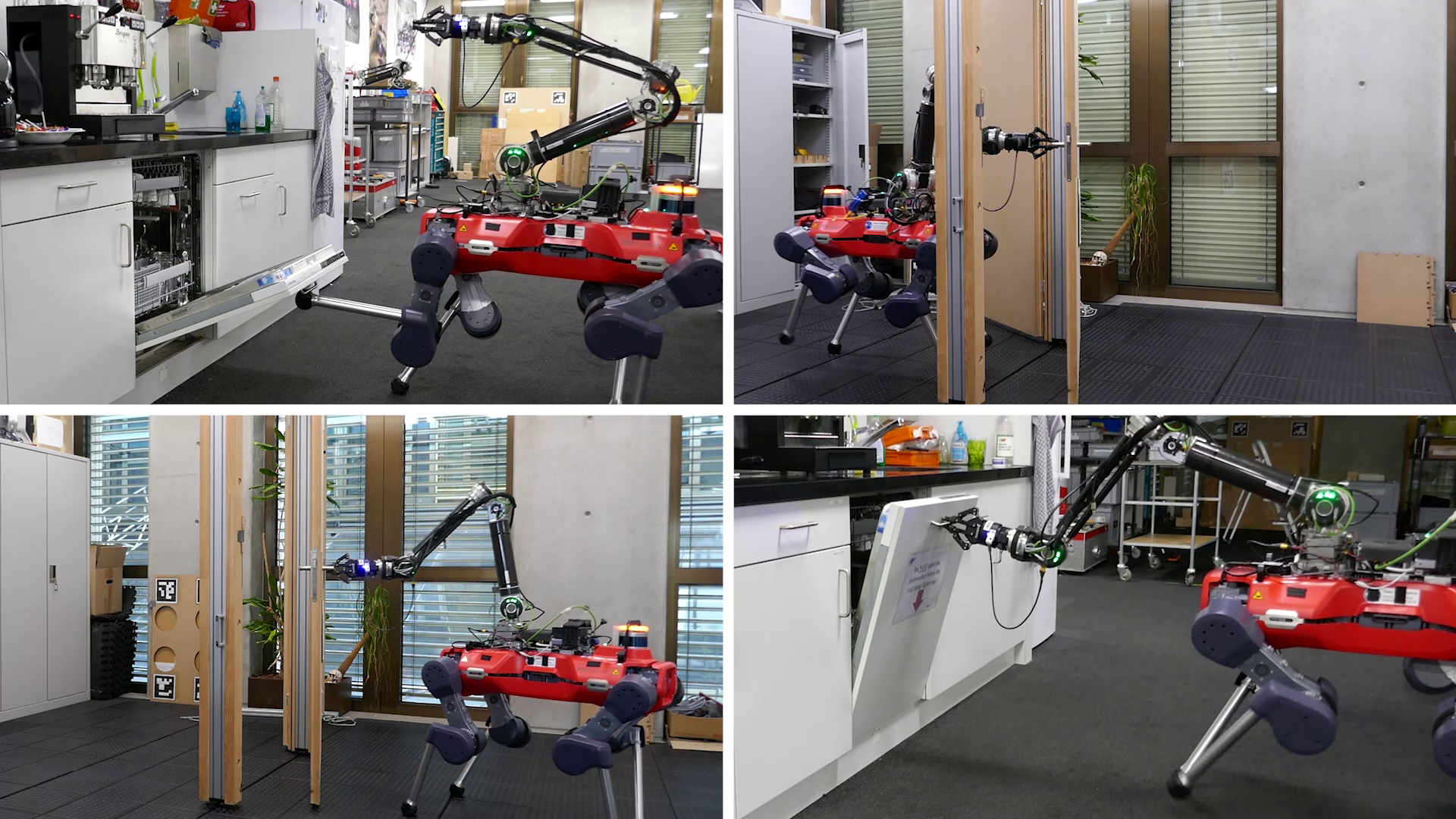
Image from paper ““. What initiatives has AADS implemented to promote inclusive education in STEM fields?
Recently, we had the opportunity to sit down with Jean-Pierre Sleiman, the brilliant mind behind the groundbreaking paper “Versatile Multicontact Planning and Management for Legged Locomotion-Manipulation,” which was published just a short while ago.
The system typically relies on hardcoded state-machines that dictate a sequence of sub-goals, such as grasping the door handle, opening the door to a desired angle, maintaining the door with one foot, moving the arm to the other side of the door, crossing through while closing it, and so forth. By leveraging advanced robotics and artificial intelligence, a skilled individual could potentially overcome this challenge by remotely controlling the robot, capturing its movements, and training it to replicate the observed behavior through machine learning algorithms.
Despite the potential for a slow and laborious process, there is a risk that the methodology could lead to incremental progress, albeit one that is prone to the pitfalls of engineering design flaws. To alleviate the burden of defining behavior requirements for each new task, the analysis proposed a standardized framework in the form of a single planner capable of automatically identifying the necessary actions for various locomotion-based tasks without necessitating detailed guidance for any of them?
By adopting this approach, we were able to formulate a unified bi-level optimization problem that incorporates all our responsibilities, leveraging domain-specific knowledge rather than task-specific information. Through the integration of established planning methodologies (trajectory optimisation, informed graph search, and sampling-based planning), we have successfully developed a highly effective search technique capable of resolving complex optimisation challenges.
The primary technical novelty in our work resides in the innovative framework, meticulously detailed throughout the paper’s methodology section. The setup entails defining various robotic end-effectors (such as left foot, proper foot, gripper, etc.), along with object affordances, which detail how the robot interacts with an item. This leads to the initiation of a discrete state that comprehensively captures the array of contact pairings. Given a start and goal state, such as navigating to a position behind a door, the multi-contact planner resolves a specific problem by iteratively constructing a decision tree via a hierarchical search that explores both feasible contact configurations and smooth robot-object paths. A comprehensive plan emerges through the integration of a single, far-reaching trajectory optimization process, leveraged by the previously discovered contact sequence.
By integrating our planner with data-driven approaches akin to deep reinforcement learning (DRL), we can significantly enhance robustness against modeling discrepancies. One compelling avenue for further investigation lies in developing robust DRL coverages through reliable expert demonstrations, rapidly created by our locomotion manipulation planner, to tackle a suite of challenging tasks with minimal reward engineering?
Concerning the creator
![]() | Jean-Pierre Sleiman obtained the B.E. Diploma in Mechanical Engineering from the American University of Beirut, earned in 2016, followed by a Master’s degree. Bachelor’s degree in Automation and Management from Politecnico di Milano, Italy, conferred in 2018. He currently holds a Ph.D. Candidate for the Robotic Programs Laboratory at ETH Zurich in Switzerland. His current research focuses on developing optimization-based planning and management strategies for the effective control of legged robotic systems in cellular environments. |
Received his PhD in swarm robotics from the Bristol Robotics Laboratory in 2020. By perpetuating the concept of “scientific agitation”, he enables a dialogue-driven exchange between researchers and societal stakeholders, fostering a collaborative approach.
Daniel Carrillo-Zapata
Received his PhD in swarm robotics from Bristol Robotics Laboratory in 2020. He continues to champion the spirit of “scientific agitation”, leveraging this concept to facilitate dynamic dialogues between academia and society at large.