The wait is over – OpenAI o3-mini is lastly right here! OpenAI has simply launched its newest reasoning mannequin, o3-mini, promising quicker and extra correct responses in comparison with its predecessors. The mannequin is now out there on the ChatGPT interface and its API companies. On this article we are going to cowl the important thing options of o3-mini and see the way it performs towards o1-mini, DeepSeek-R1, and different fashions. We will even discover ways to entry the mannequin and check out some hands-on functions. So let’s start!
What’s OpenAI o3-mini?
The o3-mini is a streamlined model of OpenAI’s most superior AI mannequin, o3, which focuses on effectivity and pace. Regardless of its compact design, it provides superior reasoning capabilities, enabling it to interrupt down complicated issues and supply efficient options. This mannequin is especially adept at coding and reasoning duties, outperforming its predecessor, o1-mini.
The mannequin is at the moment out there to all customers of ChatGPT, though free-tier customers have entry with sure limitations. In the meantime, ChatGPT Plus, Group, and Professional customers can use o3-mini for as much as 150 messages per day. Moreover, OpenAI has made the mannequin out there by way of its API companies as effectively. The o3-mini can also be accessible by way of the Microsoft Azure OpenAI Service and GitHub Copilot.
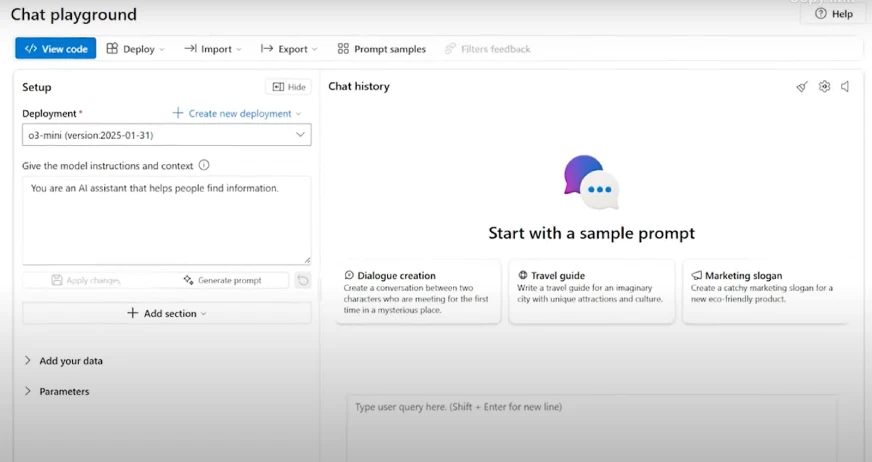
Key Options of OpenAI o3-mini
- Enhanced Reasoning: The mannequin excels in duties requiring logical reasoning and problem-solving, making it appropriate for complicated queries.
- Improved Coding Capabilities: Benchmark checks point out that o3-mini performs admirably in coding duties, providing increased accuracy and effectivity.
- Sooner Response Instances: Customers expertise faster interactions, enhancing the general person expertise.
OpenAI o3-mini BenchMark Comparisons
Now let’s see how OpenAI’s o3-mini performs compared to DeepSeek-R1, o1, o3-mini, and different distinguished fashions.
OpenAI o3-mini vs o1 vs o1-mini
First, let’s see the place o3-mini stands as compared with its predecessors.
1. Graduate-Stage Google-Proof Q&A (GPQA) Benchmark
The o3-mini (excessive) does present some enchancment over its predecessors on the subject of English language question-answering. It at the moment reveals to be one of the best OpenAI mannequin in pure language understanding.
2. American Invitational Arithmetic Examination (AIME) Benchmark
Within the AIME benchmark, the o3-mini (medium) performs virtually nearly as good because the o1 mannequin. In the meantime, the o3-mini (excessive) reveals vital enchancment in comparison with o1. With an accuracy of 87.3%, it stands as one of the best performing in mathematical reasoning as effectively.
3. Codeforces Elo Rating
The o1-mini reveals nice development in coding duties. Within the Codeforces benchmark check, the o3-mini (low) outperformed the o1-mini, whereas giving the o1 mannequin a tricky competitors. In the meantime, its medium and excessive variations carried out significantly better than the earlier fashions, making OpenAI’s o3-mini their greatest coding mannequin but.
4. SWE-bench Verified Benchmark
Even on the SWE benchmark, o3-mini proves to be one of the best OpenAI mannequin in coding, scoring 49.3% accuracy with its excessive model.
5. FrontierMath
As soon as once more, the o3-mini (excessive) mannequin has confirmed it’s dominance in mathematical problem-solving within the FrontierMath benchmark check. The outcomes present that o3-mini (excessive) is nearly twice nearly as good as its predecessors at math.
Move@1 | Move@4 | Move@8 | |
---|---|---|---|
o3-mini (excessive) | 9.2% | 16.6% | 20.0% |
o1-mini | 5.8% | 9.9% | 12.8% |
o1 | 5.5% | 10% | 12.8% |
6. LiveBench Coding
On this coding problem, all of the three variations of o3-mini have confirmed to outperform OpenAI’s o1 mannequin in LCB era. The excessive model carried out higher than o1 in code completion as effectively.
Mannequin | Reasoning Stage | Common | LCB Technology | Code Completion |
---|---|---|---|---|
o3-mini | low | 0.618 | 0.756 | 0.48 |
o3-mini | medium | 0.723 | 0.846 | 0.60 |
o3-mini | excessive | 0.846 | 0.820 | 0.833 |
o1 | excessive | 0.674 | 0.628 | 0.72 |
7. Normal Information
Testing OpenAI fashions throughout varied basic information benchmark check, reveals that o3-mini, particularly its excessive model, is a superior mannequin. Whereas it might solely present a slight enchancment over o1-mini in math, science, and different matters, its primary question-answering and reasoning capabilities are virtually twice nearly as good as o1-mini.
Class | Eval | o1-mini | o3-mini (low) | o3-mini (medium) | o3-mini (excessive) |
---|---|---|---|---|---|
Normal | MMLU(cross@t) | 85.2 | 84.9 | 85.9 | 86.9 |
Math(cross@t) | 90.0 | 95.8 | 97.3 | 97.9 | |
Math | MGSM(cross@t) | 89.9 | 55.1 | 90.8 | 92.0 |
Factuality | SimpleQA | 7.6 | 13.0 | 13.4 | 13.8 |
OpenAI o3-mini vs DeepSeek-R1 and Different Fashions
Now let’s evaluate it with the at the moment main fashions equivalent to Claude 3.5, DeepSeek-R1, and DeepSeek-V3.
1. Graduate-Stage Google-Proof Q&A (GPQA) Benchmark
Each o3-mini (medium) and o3-mini (excessive) show to be higher than DeepSeek-R1 and Claude-3.5 in detailed & factual question-answering duties.
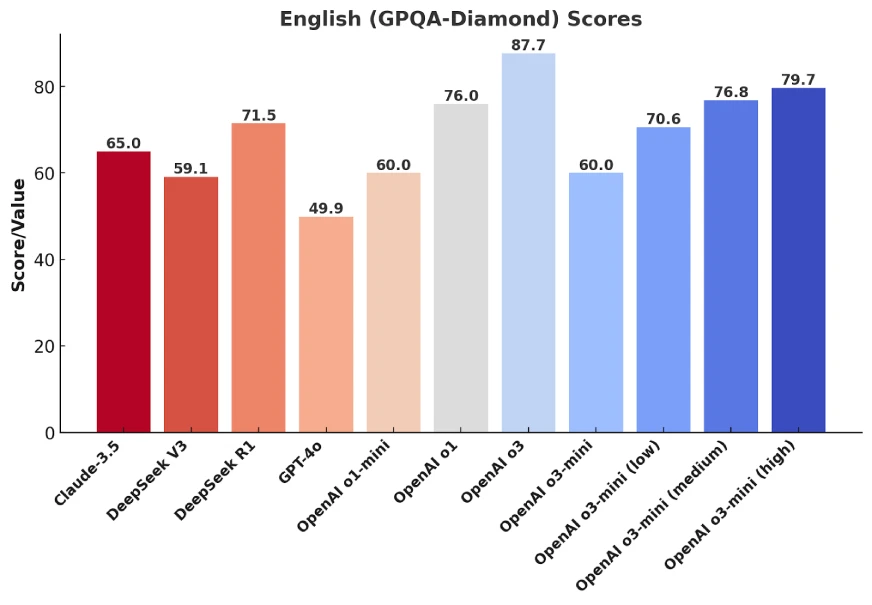
2. American Invitational Arithmetic Examination (AIME) Benchmark
Whereas o3-mini (medium) offers a detailed competitors to DeepSeek-R1 in math, the o3-mini (excessive) outperforms it by over 10%, proving its dominance within the topic.
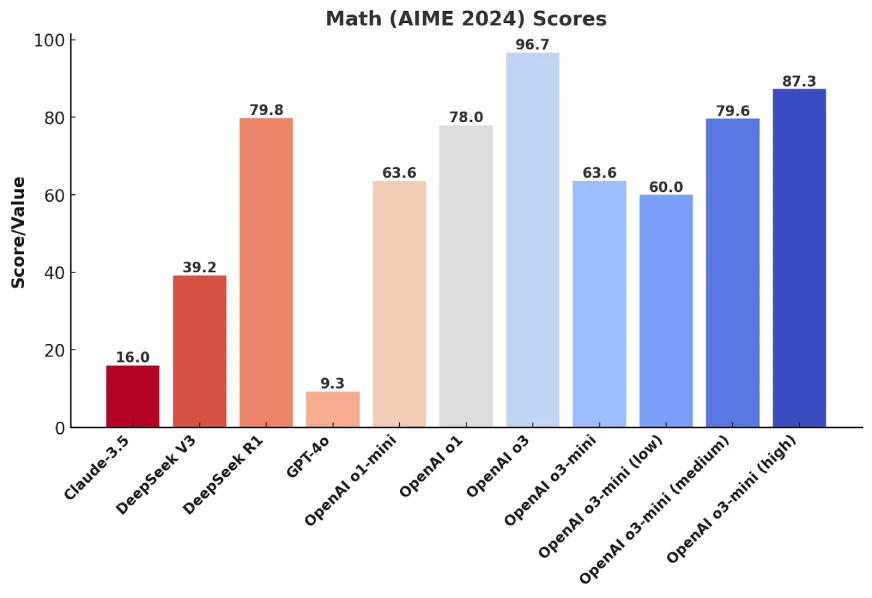
3. Codeforces Elo Rating
In the case of coding, each the medium and excessive variations of o3-mini outperform DeepSeek-R1 and the opposite fashions. The Elo rating of o3-mini (excessive) is the present highest amongst all of the fashions out there right this moment.

4. SWE-bench Verified Benchmark
In the case of dealing with real-world software program engineering issues, Claude 3.5 nonetheless stands as one of the best performing mannequin. Nevertheless, o3-mini (excessive) offers it a detailed competitors, marginally overtaking DeepSeek-R1.
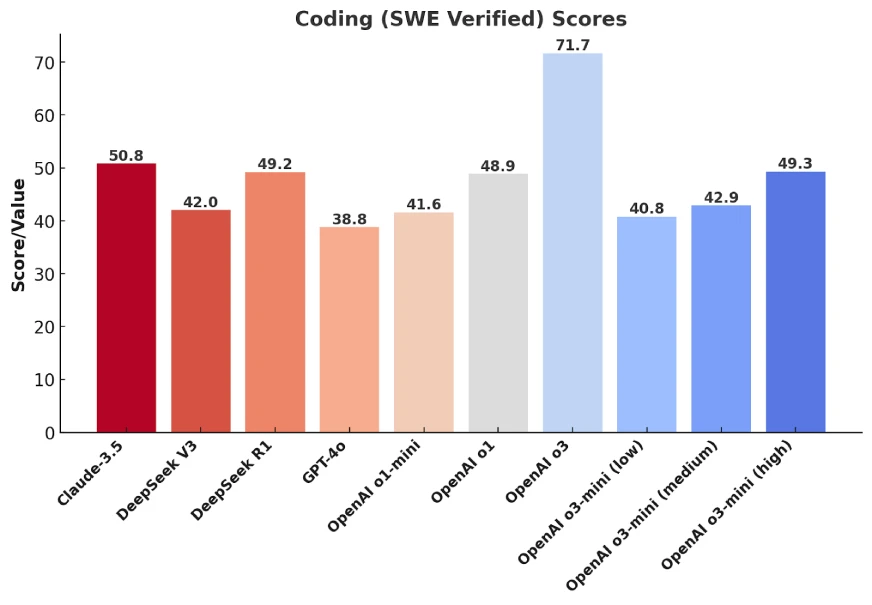
In all of those comparisons, we see the but to return o3 mannequin outperform others.
Sources:
How one can Entry OpenAI’s o3-mini?
There are 2 methods to entry o3-mini. The primary is thru their chatbot interface, utilizing ChatGPT. The second is by way of API. We are going to information you thru each choices within the subsequent part.
Accessing OpenAI o3-mini by way of ChatGPT
Free-tier customers of ChatGPT can expertise the potential of o3-mini by choosing ‘Motive’ beneath the message field.
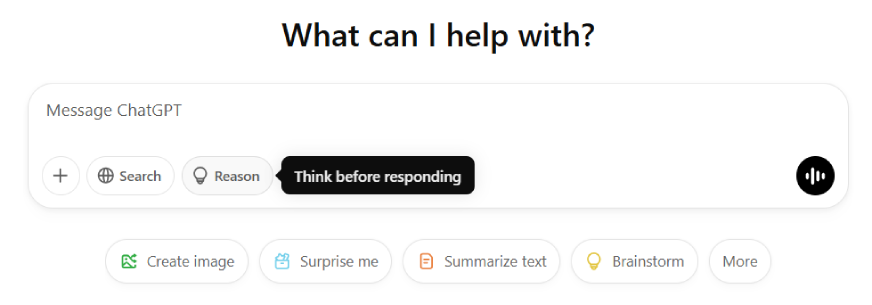
ChatGPT Plus, Group, and Professional customers can instantly choose “o3-mini” or “o3-mini-high” from the mannequin picker drop down record on the prime, and begin utilizing it.
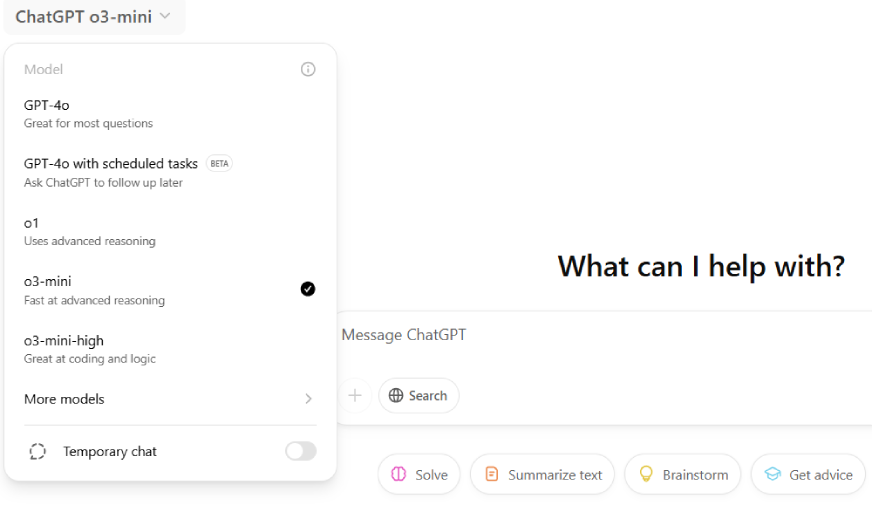
How one can Entry OpenAI’s o3-mini by way of API?
Right here’s how one can entry OpenAI’s o3-mini utilizing their API.
Step 1: Join API Entry
In case you are not already a part of the OpenAI beta program, you’ll have to request entry by visiting OpenAI’s API web page. When you enroll, you could want to attend for approval to entry the o3-mini fashions.
Step 2: Generate an API Key
Upon getting entry, log in to the OpenAI API platform and generate an API key. This secret’s needed for making API requests. To generate the important thing, go to API Keys and click on on “Create New Secret Key”. As soon as generated, be sure to repeat the important thing and reserve it securely.
Step 3: Set up the OpenAI Python SDK
To work together with the o1 API, you will want to put in the OpenAI Python SDK. You are able to do this utilizing the next command:
!pip set up openai
This bundle means that you can make API requests to OpenAI out of your Python code.
Step 4: Initialize the OpenAI Shopper
After putting in the OpenAI SDK, it’s good to initialize the consumer by organising the API key:
import os os.environ["OPENAI_API_KEY"] = "Enter_api_key"
Step 5: Execute and Confirm the Output
Now that we’ve got arrange the OpenAI consumer, let’s attempt utilizing the o3-mini mannequin to unravel this downside on LeetCode.
completion = consumer.chat.completions.create( mannequin="o3-mini", messages=[ {"role": "developer", "content": "You are a helpful assistant."}, { "role": "user", "content": """ You are given two non-empty linked lists representing two non-negative integers. The digits are stored in reverse order, and each of their nodes contains a single digit. Add the two numbers and return the sum as a linked list. You may assume the two numbers do not contain any leading zero, except the number 0 itself. Example 1: Input: l1 = [2,4,3], l2 = [5,6,4] Output: [7,0,8] Clarification: 342 + 465 = 807. Instance 2: Enter: l1 = [0], l2 = [0] Output: [0] Instance 3: Enter: l1 = [9,9,9,9,9,9,9], l2 = [9,9,9,9] Output: [8,9,9,9,0,0,0,1] Constraints: The variety of nodes in every linked record is within the vary [1, 100]. 0
Output:
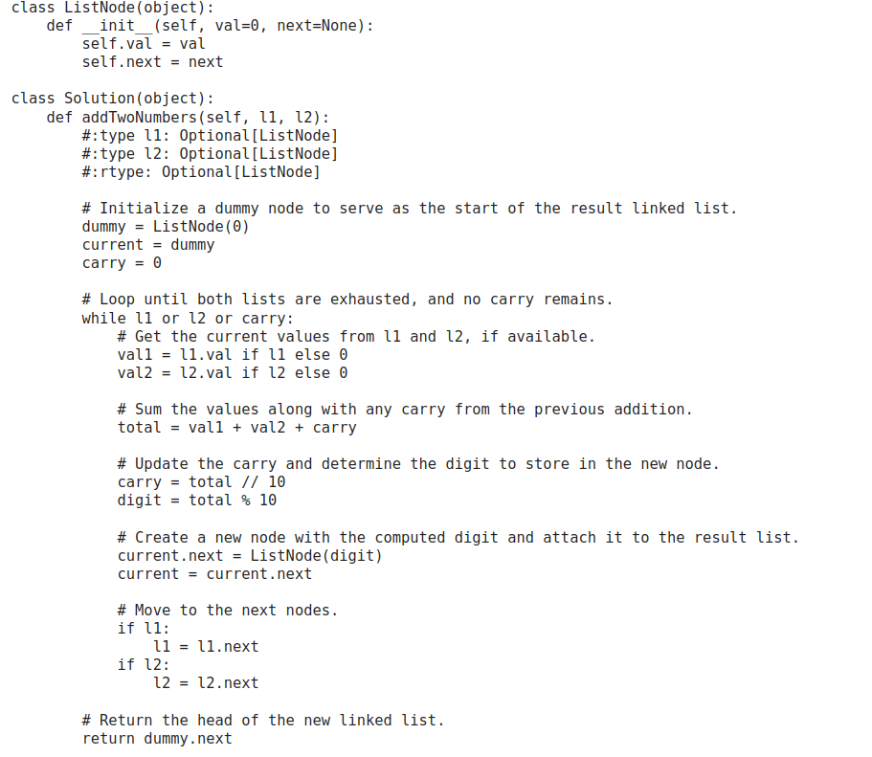
Arms-on Functions of o3-mini
Now let’s check out the o3-mini and check its logical reasoning, mathematical reasoning, and coding abilities.
Job 1: Logical Reasoning
Immediate: “You’ve gotten eight balls, one among which is barely heavier than the others. You’ve gotten a steadiness scale however can solely use it twice. How do you establish which ball is the heaviest?”
Output:
“To search out the heaviest ball among the many eight utilizing a steadiness scale solely twice, observe this optimum weighing technique:
Step 1: First Weighing
- Divide the 8 balls into 3 teams:
- Group A: 3 balls
- Group B: 3 balls
- Group C: 2 balls (put aside)
- Place Group A on the left pan and Group B on the correct pan of the steadiness.
Step 2: Analyze the First Weighing Consequence
- If one facet is heavier:
- The heavier facet comprises the heaviest ball.
- Now, you’ve 3 balls left to check within the subsequent step.
- If the dimensions is balanced:
- The heaviest ball is among the many 2 balls in Group C.
- Transfer to Step 3.
Step 3: Second Weighing
- If the heaviest ball is in a set of 3 balls (from Step 2):
- Choose two balls from this set and place one on every pan.
- If one facet is heavier → that’s the heaviest ball.
- If the dimensions is balanced → the third ball (not weighed) is the heaviest.
- If the heaviest ball is in Group C (2 balls):
- Weigh the 2 balls towards one another.
- The heavier one is the reply.
Conclusion
This methodology ensures that you’ll determine the heaviest ball in precisely two weighings.”
Job 2: Mathematical Reasoning
Immediate: “If I make investments Rs 1 lakh in a checking account that gives a 5% annual rate of interest, compounded yearly. How a lot will I’ve within the account after 10 years? Present a step-by-step calculation.”
Output:
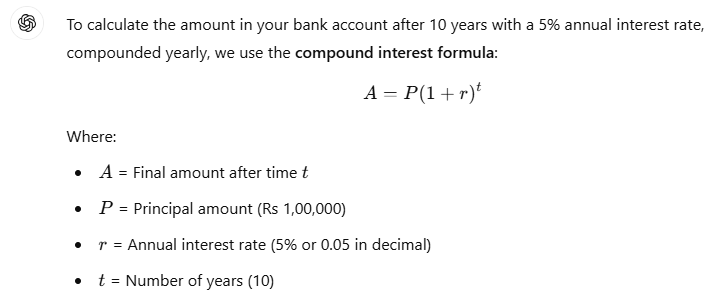

Job 3: Coding
Immediate: “You might be given a dataset with 1 million rows and wish to scrub and preprocess it effectively. Write a Python script utilizing Dask or multiprocessing to deal with lacking values, normalize numerical options, and apply one-hot encoding to categorical columns. Clarify why your method is extra environment friendly than utilizing pandas alone”
import dask.dataframe as dd from dask_ml.preprocessing import StandardScaler import pandas as pd import dask.multiprocessing def preprocess_data(file_path): """ Effectively preprocesses a big dataset utilizing Dask. Steps: - Load knowledge in parallel - Deal with lacking values - Normalize numerical options - One-hot encode categorical columns """ # Load dataset utilizing Dask df = dd.read_csv(file_path) # Fill lacking values df = df.fillna(df.imply()) # Establish numerical and categorical columns numerical_cols = df.select_dtypes(embrace=['float64', 'int64']).columns categorical_cols = df.select_dtypes(embrace=['object']).columns # Normalize numerical options scaler = StandardScaler() df[numerical_cols] = scaler.fit_transform(df[numerical_cols]) # One-hot encode categorical columns df = dd.get_dummies(df, columns=categorical_cols) # Compute the ultimate end in parallel df = df.compute(scheduler="multiprocessing") return df # Instance utilization # processed_df = preprocess_data("large_dataset.csv") # processed_df.to_csv("cleaned_data.csv", index=False) """ Why this method is environment friendly: 1. **Dask for Parallel Processing:** In contrast to pandas, which masses knowledge into reminiscence, Dask processes massive datasets in parallel utilizing activity scheduling, making it reminiscence environment friendly. 2. **Lazy Analysis:** Dask masses solely the required chunks of knowledge, stopping reminiscence overload. 3. **Multiprocessing Scheduler:** Makes use of a number of cores to hurry up computation, outperforming pandas in large-scale knowledge operations. """
Additionally Learn: DeepSeek R1 vs OpenAI o1 vs Sonnet 3.5: Battle of the Greatest LLMs
Conclusion
Breaking all benchmarks, OpenAI’s o3-mini certainly represents a major development in AI mannequin growth. It appears to supply a great steadiness between efficiency and effectivity. Its enhanced reasoning and coding capabilities, coupled with quicker response instances, make it the most effective and best fashions we at the moment have. The broad accessibility of o3-mini to all customers and in addition by way of the API ensures that a variety of customers can leverage its capabilities.
So go forward, attempt it out, and tell us within the feedback how good you suppose it’s!
Incessantly Requested Questions
A. A. OpenAI o3-mini is a streamlined model of OpenAI’s newest reasoning mannequin, o3. It’s designed for quicker and extra environment friendly efficiency, significantly in logical reasoning and coding duties. In comparison with o1-mini, o3-mini provides improved accuracy, higher problem-solving capabilities, and better benchmark scores.
A. Sure, free-tier customers of ChatGPT can entry o3-mini below the “Motive” mode, however with limitations. For prolonged utilization, ChatGPT Plus, Group, and Professional customers get entry to as much as 150 messages per day.
A. o3-mini (excessive) achieves 87.3% accuracy on the AIME benchmark, making it OpenAI’s best-performing mannequin in mathematical reasoning. It additionally outperforms DeepSeek-R1 in logical reasoning checks, exhibiting vital enhancements over earlier fashions.
A. The mannequin has prime scores in coding benchmarks like Codeforces Elo and SWE-bench. The excessive model of o3-mini is OpenAI’s greatest coding mannequin but, surpassing o1 and o1-mini in real-world programming challenges.
A. OpenAI’s o3-mini outperforms DeepSeek-R1 in a number of areas, significantly in reasoning, coding, and mathematical problem-solving. Whereas each fashions are optimized for effectivity, o3-mini achieves increased scores on all key benchmarks.
A. Builders can entry o3-mini by way of OpenAI’s API and Microsoft Azure OpenAI Service. To make use of it, it’s good to generate an API key, set up the OpenAI Python SDK, and make API calls utilizing the “o3-mini” mannequin identifier.
A. OpenAI is predicted to launch o3, a extra highly effective model with additional enhancements in reasoning, coding, and real-world software dealing with. Based mostly on present benchmarks, o3-mini’s sturdy efficiency hints at an much more succesful upcoming mannequin.