Monetary Valuations & Comparative Evaluation
Institutional investors such as hedge funds, market makers, and pension funds have traditionally been at the forefront of embracing cutting-edge analytical techniques and innovative alternative data sources. On the intensely competitive business landscape, those who excel are often able to capitalize on their success by quickly synthesizing and acting upon a broad range of insights to gain a strategic advantage.
As generative AI technology continues to mature, its impact on the financial services industry has become increasingly apparent, bridging the longstanding gap between buy-side and sell-side market dynamics. Leaders have recognized the transformative potential of giant language models (LLMs) and artificial intelligence technologies to revolutionize their financial analysis teams. Without hesitation, numerous entities have already committed resources to pilot projects and proof-of-concepts, often emerging from their respective data science divisions. As we converse, the fight to establish dominance hinges on not only being the first to source accurate information, but also on converting complex technical concepts into practical, reliable solutions that empower businesses to make informed decisions.
Major financial institutions are actively exploring ways to operationalize AI-driven models through bespoke, interactive visualizations specifically designed for financial analysts to facilitate informed decision-making. As forward-thinking financial institutions seek to leverage innovative tools, they must harmonize these advancements with existing analytics platforms, while meeting stringent governance demands. To implement this feature cost-efficiently, they must avoid vendor lock-in and ensure seamless integration with best-of-breed capabilities while accommodating the continuous evolution of AI requirements from the open-source community.
When evaluating options to build or acquire a reliable and trustworthy GenAI system for financial modeling purposes, consider the following key aspects:
- Knowledge Assortment
- RAG Workflow
- Deployment, Monitoring & Consumer Interface
Knowledge Assortment
Searching for alpha? A crucial first step towards informed decision-making is having access to comprehensive, crystal-clear, easily discoverable, and trustworthy information that sets the foundation for sound investment strategies. The Lakehouse Platform provides a catalyst for making this feasible, combined with the flexibility and governance necessary to adapt to the rapidly evolving landscape of Generative Artificial Intelligence (Gen AI).
Capital markets groups consistently rely on and manage a broad spectrum of market analysis and analytics software solutions. While financial analysts may find great value in these tools, they can often seem isolated from the richer insights gathered by their IT colleagues. This case may lead to inefficient duplicated storage and hinder data analysis outside the primary cloud environment?
A crucial methodology for designing such functions is required, yet a mismatched response may impede the Gen AI’s progress within the pilot program if not in sync with the larger community’s goals. The one trillion-dollar pension fund disqualified a proposal, fearing that implementing the innovative solution would necessitate replicating existing infrastructure and data across a secondary cloud architecture. By leveraging an open-source architecture and scalable data storage formats, a centralized repository can efficiently accommodate an extensive range of input documents for seamless processing by the General Artificial Intelligence model. While there may already exist a substantial amount of publicly available, owned, or purchased documentation and data, this existing knowledge can be harnessed to avoid costly duplication and redundant procedures.
As the scope of documentation widens, a model’s capacity for comprehensive security and diverse intelligence is enhanced, yielding richer insights.
Some paperwork to thoroughly consider submitting for a General Artificial Intelligence-based monetary valuation assessment includes:
- 10-Ok and different public studies
- Fairness & analyst studies
- Analyst video transcripts
- Different paid market intelligence studies
- Non-public fairness evaluation
A sample remains the most common approach to feeding these documents into the analytics platform. Knowledge engineers can design and develop automated workflows for processing routine documents and data sets with ease. To facilitate ad-hoc document ingestion, consider providing a user-friendly graphical interface for financial analysts to utilize seamlessly.
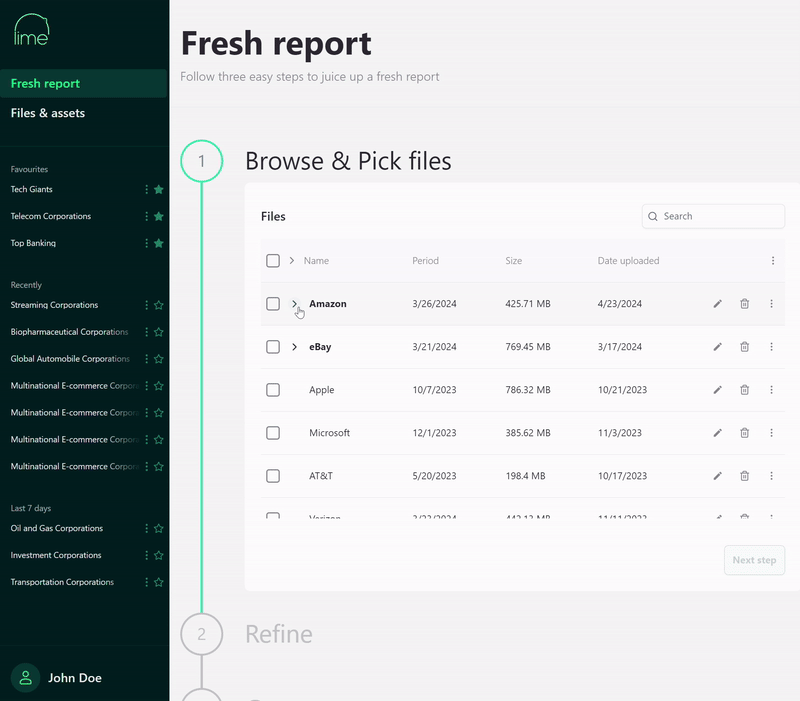
RAG Workflow
At the core of any Generative AI-based response lies the Retrieval-Augmented Generation (RAG) workflow or pipeline. These workflow templates align your unique personal data and organizational specifications with your selected Large Language Models (LLMs). When using the RAG structure, one leverages a pre-trained large language model (LLM) by posing inquiries based on their unique, proprietary information, as opposed to relying solely on the knowledge provided to the LLM during training. This methodology aligns seamlessly with the “” technique, which is adept at grasping the nuanced semantics of your data.
For software developers, the RAG example bears a striking resemblance to working with APIs – augmenting requests by integrating services from disparate pieces of software. For those who are less technically inclined, consider the RAG sample like a trusted friend who can offer valuable advice; provide them with your personal notes and send them off to the library. Before soliciting their responses, we clarify the parameters and prompt the participants to think critically, allowing for a more in-depth analysis of their answers.
The RAG workflow streamlines handoffs by incorporating tailored directions that accommodate unique information sources, custom calculations, and organizational guardrails specific to each enterprise’s distinct context.
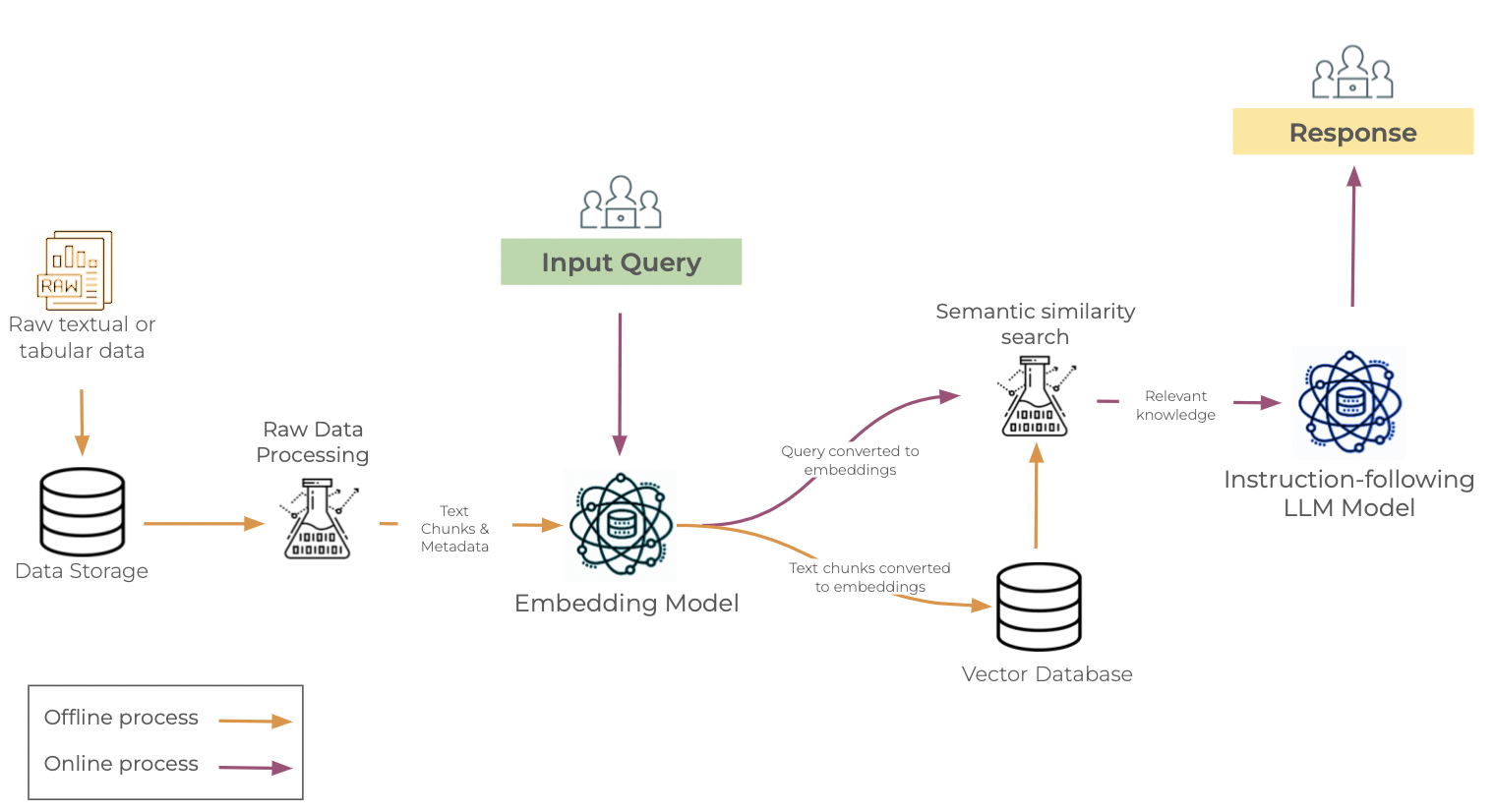
Open structure. Open fashions.
Having trouble securing investment in a bespoke Return on Assets Governance (RAG) framework? Establishing a flexible foundation is crucial for building trust within your team before transitioning to production, allowing for seamless implementation of solutions. Effective visibility and management of your Red Amber Green (RAG) workflow are crucial in enhancing explainability and credibility. A crucial decision for a prominent personal fairness investor was jeopardized when their AI-generated response was rejected due to inconsistent results, as they were unable to reproduce equivalent outcomes using identical inputs week-over-week; the underlying model and/or RAG workflow had undergone changes, with no feasible means of reverting back to an earlier version.
While initial hype surrounded business-generated artificial intelligence fashion trends, open-source alternatives have been gaining momentum and continuing to innovate. In addition to refining processes through tailored RAG workflows, the merits of open-source approaches make a strong argument against commercial alternatives in terms of efficiency and cost-effectiveness.
A flexible response readily adapts to seamlessly integrate within the latest open-source framework. As a result, generative AI models built with adaptable RAG workflows were immediately empowered to capitalize on Databricks’ open-source framework, which surpassed both established open-source and commercial alternatives in terms of performance. As a direct result of the open-source community’s unwavering dedication to innovation, fresh, high-performing designs are continually released on a quarterly basis.
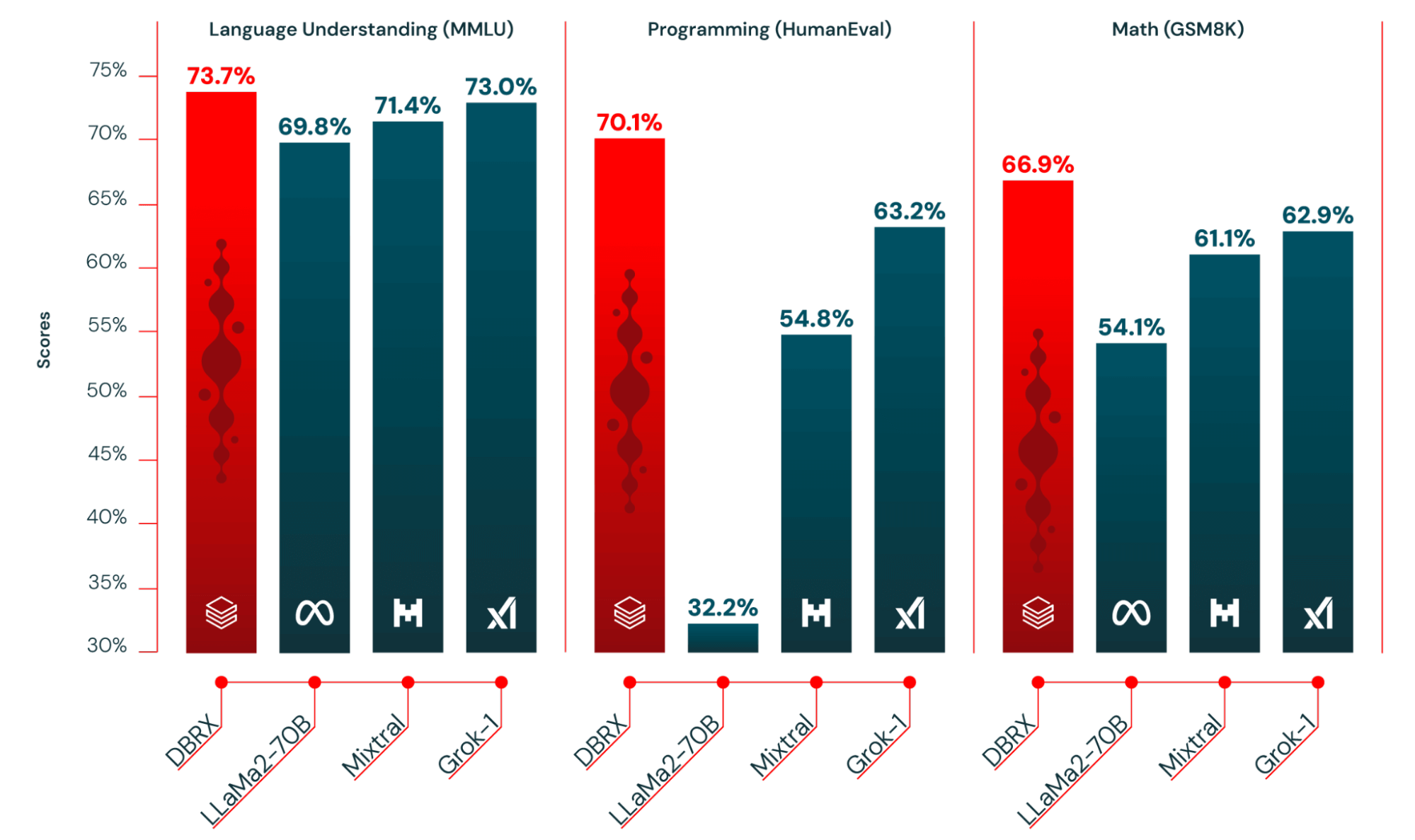
Value & Efficiency
As generative artificial intelligence (AI) software adoption accelerates within financial institutions, the cost of these solutions will increasingly come under scrutiny to justify their value and ROI. A pilot implementation leveraging business-grade generative artificial intelligence may initially incur a manageable cost, with only a select few experts utilising the solution for a limited period. As the volume of personal data, service-level agreement response times, query intricacy, and request diversity escalate, exploring cost-effective alternatives will become increasingly justified.
The costs incurred by a team conducting a monetary evaluation will vary depending on the demands placed upon them by clients. At this prominent financial institution, the tolerance for response times was found to be over two minutes during a controlled pilot test; however, expectations shifted significantly when considering a large-scale production rollout, necessitating a service-level agreement (SLA) that demanded partial output generation within a mere 60 seconds. By offering a diverse range of the latest open-source fashion designs and underlying infrastructure, it is possible to achieve the optimal balance between cost and performance for various use cases, thereby providing a value-efficient scaling solution that is particularly valuable for financial institutions.
Flexibility
Whether to choose open-source Large Language Models (LLMs) or OpenAI ultimately hinges on your specific needs, resources, and limitations. For those prioritizing customization, cost-effectiveness, and information privacy, open-source large language models (LLMs) might prove a more suitable choice. If your organisation demands cutting-edge textual content technology and is willing to invest in premium solutions, business options may prove a wise decision. When choosing a platform, it is essential to consider one that provides a comprehensive range of options and ensures future-proofing by being adaptable to the rapid changes in technology. The Databricks Intelligence Platform uniquely provides seamless management, accommodating even the most intricate customizations and complexities, as outlined below:
| Pre-training | Developing Coaching and Leadership Mastery from Scratch: A Comprehensive Approach |
High-quality-tuning | Comparing fine-tuned language models with diverse datasets and domains requires nuanced evaluations akin to monetary appraisals. | |
Retrieval Augmented Era (RAG) | Combining Large Language Models (LLMs) with enterprise information akin to private and non-private financial studies, transcripts, and other financial data? | |
Immediate Engineering | Developing customized prompts for Large Language Models (LLMs) to generate specialized insights, whether static studies or integrated into a visual exploration tool for financial analysts. |
Deployment, Monitoring & Consumer Interface
Once all financial documents, both private and public, are integrated and a Risk Assessment and Governance (RAG) workflow is established in alignment with your organization’s specific context, you will be empowered to explore model deployment options, while also granting financial analysts access to the model.
Databricks provides a comprehensive suite of current and future deployment options, enabling effective initial rollouts and ongoing monitoring, governance, validation, and scaling capabilities. Key deployment associated capabilities embrace:
- Provisioned and on-demand optimized computing infrastructure for large language model serving.
- Professional editor’s revised version:
Evaluation of MLFlow Large Language Model (LLM) for Verifying Mannequin Accuracy and Excellence
- Databricks Vector Search
- What potential do Large Language Models (LLMs) hold in facilitating the automation of various processes?
By leveraging their vast knowledge and capabilities, we can potentially streamline tasks such as sentiment analysis, language translation, and text summarization.
Moreover, the ability to analyze large volumes of data quickly and accurately enables LLMs to identify patterns and trends, allowing for more informed decision-making.
As a result, it is crucial that we continue to develop and refine these models to unlock their full potential in various industries.
SKIP
- What’s a game-changer in RAG workflow optimization? Previewing the RAG Studio to streamline operations and amplify productivity.
- Improved Text: LakeHouse monitoring streamlines automated scanning and alerting for hallucinations or inaccuracies.
By combining these choices and tools, data scientists are empowered to quickly respond to insights from financial analysts. As expertise with mannequin high quality advances, the mannequin’s utility, pertinence, and precision enhance concurrently, yielding faster and more influential financial intelligence ultimately.
Monetary analysts’ traditional reliance on manual data analysis and forecast modeling will evolve as they collaborate with generative artificial intelligence (Gen AI).
Financial analysts necessitate a harmonious collaboration with generative artificial intelligence models that resonates with the demands of their daily responsibilities. Valuations and comparative evaluations are complex, iterative processes that necessitate a systematic approach to maintaining momentum. The interactive character of the collaboration between financial analysts and models involves requests to expound specific sections of a produced financial summary, or to structure bibliographic entries and citations.
T1A, a Databricks collaborator, has developed Lime to fulfill this purpose. The Lime platform provides a user-friendly interface tailored specifically for financial analysts, leveraging Databricks’ capabilities and aligning with the Gen AI concepts discussed in this piece.
The report showcases the capabilities of Large Language Models (LLMs), along with the ability for analysts to expand upon key points through an intuitive, point-and-click interface.
Analysts are adept at producing summaries for individual stocks and mixed studies for comparative analysis. By leveraging a chat-based and dynamic reporting interface, users can initiate targeted follow-ups that delve into specifics like “What drove the variation in EBITDA across the most recent period?” or “Which key factors could potentially impact business value over the next 12 months?”
The interface offers analysts options to provide ratings for paragraphs, charts, and embellishments as they collaborate. With its added layer of premium governance, this cycle provides valuable recommendations that can foster a form of self-reinforcing learning, ultimately leading to adaptations in the RAG Workflow and model calibration. The more detailed financial analyses are used to support conclusions, the clearer they display a company’s unique circumstances and the greater the strategic implications?
Conclusion
The path to discovering alpha is well-laid by a robust Gen AI foundation. Utilizing a standardized framework for ingest, our system seamlessly integrates with existing open storage solutions, ensuring seamless collaboration and eliminating redundant financial documentation. Through sustained investment in RAG Workflows, enterprises can foster development and strategic differentiation by creating a contextual understanding of their business environment that is both transparent and replicable. To ensure subsequent deployments are executed efficiently, leveraging the latest open-source frameworks is crucial, while continuously monitoring for quality and accuracy to guarantee seamless outcomes. Finally, incorporate a user-friendly interface to ensure ongoing engagement and adoption among financial analysts.
About T1A
T1A is a specialized consulting agency that helps enterprises unlock the full potential of Databricks, as well as being the developer of Lime – Gen AI for financial valuations. T1A, SAS-to-Databricks migration experts, have created GetAlchemist.io, a prominent profiler and automated code transformation solution.
To gain in-depth insights on how financial analysts leverage a custom-built Gen AI-powered interface designed specifically for financial valuations and comparative analysis, visit. Explore our interactive video showcase or schedule a tailored demo to experience firsthand how our Gen AI solution empowers demand generation and fosters seamless collaboration among internal stakeholders.