The concept of shape-shifting robots has been a staple of science fiction since at least 1991, when the T-1000 Terminator model debuted in the popular film. Terminator 2: Judgment Day. Scientists have long envisioned creating a robot capable of transforming its shape to accomplish various tasks since at least that time, if not before.
While the concept of shape-shifting is fascinating, the text could be improved in terms of clarity and coherence. Here’s a revised version:
As we start to see these issues manifest, one notable example is the case of or this remarkable phenomenon where a substance can change its form to evade capture, much like the mythical ability to melt and re-form itself to flee from jail. While each of these solutions requires external magnetic control systems to function effectively? They can not transfer independently.
A team of analysts at MIT is working on developing ones that might. Researchers have designed a groundbreaking machine-learning system that enables a reconfigurable “slime” robot to adapt seamlessly to its surroundings by transforming shape, texture, and form in real-time. Despite initial expectations, reality check: robots are not constructed from liquid steel.
Terminator 2 Judgment Day Clip – Hospital Escape 1991?
“According to Boyuan Chen, a researcher at MIT’s Computer Science and Artificial Intelligence Laboratory, people tend to associate “smooth robots” with devices that can stretch or deform but then return to their original shape.” “Our robotic system has the unique ability to transform its shape, much like slime, which could revolutionize its potential applications.” It’s remarkable that our methodology worked so seamlessly because we were dealing with something entirely novel.
Researchers faced the challenge of developing a control method for an armless, legless slime robot lacking skeletal structure to power its movements, with no fixed location for its muscle actuators. A shapelessness so profound, coupled with a complexity so ceaselessly in flux – this conundrum is akin to a waking nightmare: what feasible means can be devised to design a framework for programming the actions of such a robotic entity?
As the conventional management framework proved inadequate in addressing the complex situation, the workforce sought alternative solutions by embracing AI’s vast capabilities to tackle intricate information and streamline processes. Developing a sophisticated management algorithm, they enabled learning processes for optimal transfer, stretching, and shaping of blob-like robotic entities, often requiring multiple iterations, to successfully complete specific tasks.
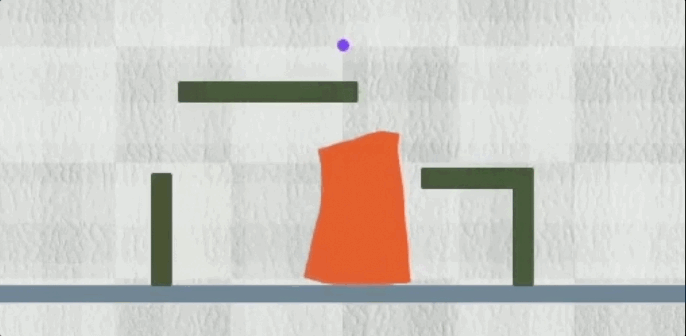
MIT
Reinforcement learning is a machine-learning approach that enables software programs to learn by making decisions through trial and error. Effective reinforcement learning is achieved when coaching robots with clearly defined transferable components, such as grippers with ‘fingers’, which can be incentivized for actions that bring them closer to a target – for example, picking up an egg. Here are two swirling vortex-like structures that are governed by complex interactions between magnetic fields.
“According to Chen, a robot of this complexity could potentially feature hundreds of tiny muscles that require precise regulation.” Studying in a conventional sense proves quite burdensome.
To effect a meaningful transformation in a slime robot, massive portions of its material must be relocated simultaneously to achieve a practical and efficient outcome; attempting to manipulate individual particles would yield no substantial change. Researchers employed an unconventional approach to reinforce learning.
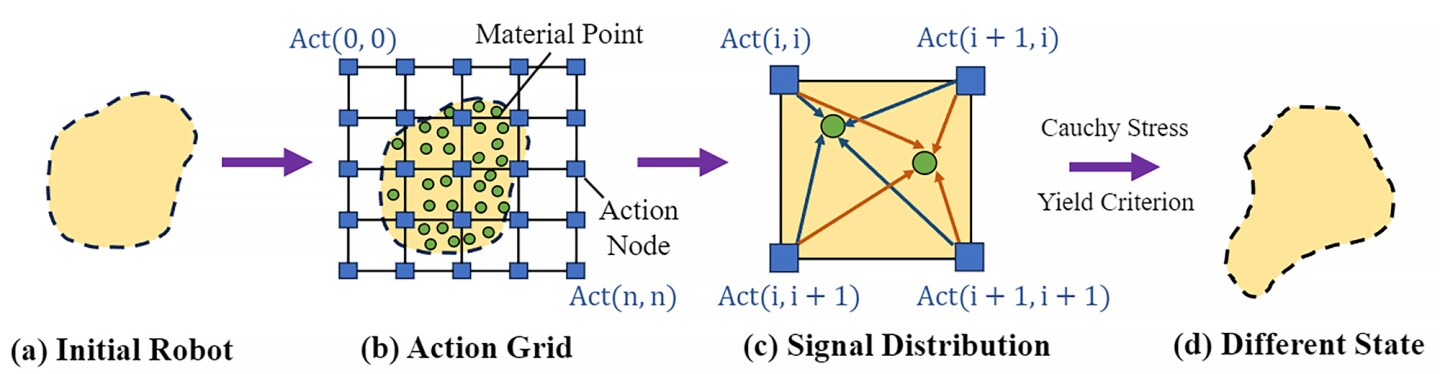
Huang et al.
In reinforcement learning, the agent’s decision-making space is represented by an “action space” – a set of all feasible actions or selections that the agent can take as it interacts with its environment, commonly referred to as the “action space.” The researchers employed a mannequin that utilized photos of the robotic’s atmospheric conditions to create a 2D simulation of a habitable environment, overlaid with a grid featuring various factors.
As researchers’ algorithm recognized, nearby pixels in an image are linked, and similarly, adjacent motion factors exhibit robust correlations. As the robotic arm adjusts its shape, collective motion factors are transferred simultaneously, whereas those on the leg exhibit a distinct pattern of simultaneous movement, differing from the arm’s dynamic.
Researchers also designed an algorithm incorporating “coarse-to-fine” coverage learning. Initially, it’s trained using a low-resolution coarse coverage – essentially processing large segments – to identify the motion space and recognize prominent motion patterns. A high-definition, comprehensive approach dives further to refine the robot’s capabilities and amplify its capacity to execute intricate tasks seamlessly.
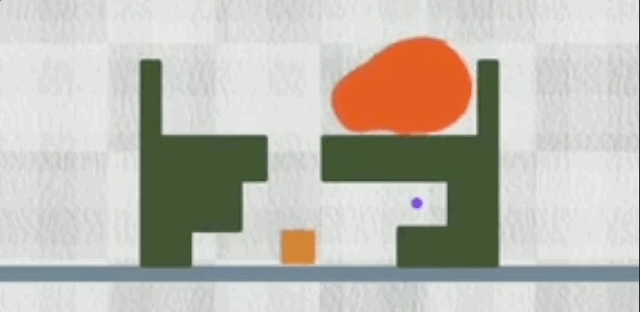
MIT
Vincent Sitzmann, a researcher at CSAIL and co-author of the study, noted: “Coarse-to-fine suggests that even arbitrary motions are more likely to produce discernible results.” “The impact on the outcome is likely to be significant when you simultaneously manage multiple muscle groups.”
The subsequent steps were to check their approach. Developed was DittoGym, a simulated environment featuring eight distinct tasks that challenge the reconfigurability of a robotic system’s ability to adapt its shape. The robot’s capabilities expand as it learns to synchronize its actions, precision-matching letters or images with agility: developing, digging, kicking, catching, and running with calculated ease.
The emergence of a new paradigm in organisational management has been heralded by MIT’s pioneering work on slime-based robotics. This innovative approach seeks to revolutionise traditional command-and-control structures by embracing the unique properties of slimy organisms.
For instance, one potential application is the development of autonomous systems capable of adapting to changing environments through their ability to mimic the self-healing properties of certain slimes.
According to Suning Huang, a visiting researcher at MIT and co-author from Tsinghua University’s Department of Automation in China, DittoGym’s process choice adheres to standard generic reinforcement learning benchmarks and caters to the unique requirements of reconfigurable robots.
“Each process is engineered to embody fundamental characteristics we consider essential, such as the ability to explore over long horizons, investigate atmospheric conditions, and collaborate with external entities,” “We believe that together, these components will provide customers with a comprehensive grasp of the versatility of reconfigurable robots and the efficacy of our reinforcement learning approach.”
DittoGym
Researchers found that their coarse-to-fine algorithm consistently outperformed alternative approaches (such as coarse-only or fine-tuned from scratch methods) across all tasks in terms of effectiveness.
While it may take some time for shape-changing robots to venture out of the laboratory, this research marks an important milestone on the journey. Researchers aim to inspire the creation of reconfigurable robots capable of traversing the human body or being integrated into wearable systems, potentially paving the way for innovative applications in medicine and beyond.
The study was published online in its preprint form. .
Supply: