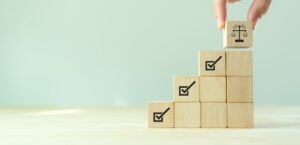
(3rdtimeluckystudio/Shutterstock)
Financial services companies are eager to leverage generative AI to reduce costs, drive revenue growth, and elevate customer satisfaction, mirroring the industry’s broader adoption trend. Despite these concerns, the perils associated with GenAI are certainly not insignificant, especially in financial services. Recently, TCI Media welcomed consultants from EY to help financial services (FinServ) practitioners kick-start their responsible AI initiatives.
Recently, an event took place in New York City in mid-September, which was attended by two AI consultants from EY Americas, along with Rani Bhuva, Principal at EY and Responsible AI Chief for EY Americas Financial Services, as well as Kiranjot Dhillon, Senior Manager at EY and Chief of AI for EY Americas Financial Services.
Monetary services companies seeking to integrate artificial intelligence, or AI, into their operations often encounter significant regulatory hurdles. In “Accountable AI: Regulatory Compliance Developments and Key Concerns for AI Practitioners,” Bhuva and Dhillon identified numerous challenges these firms face when attempting to adopt AI, including GenAI, as well as several steps companies can take to get started.
Financial services firms are no strangers to regulation, and they typically find numerous regulatory developments occurring globally within the FinTech space. The EU’s AI Act has garnered international attention, while in the US, approximately 200 data and AI-related bills are under development at the state level, according to Bhuva.
On the federal level, much of the progress made thus far has been facilitated by actions taken since early 2023 and initiatives spearheaded by President Joe Biden, according to her statement. The Federal Communications Commission (FCC) and the Federal Reserve have directed financial institutions to adopt the risk management and governance guidelines developed over the past decade and apply them to GenAI, said the representative.
While federal regulators are largely in a monitoring posture regarding GenAI, they remain vigilant and prepared to take action as needed. Companies like the US Treasury and Client Financial Protection Bureau (CFPB) have released requests for input to gather industry suggestions, whereas regulators such as FINRA and SEC have clarified certain rules regarding customer data in AI apps.
One key takeaway has been a clear emphasis on aligning with the National Institute of Standards and Technology (NIST). The challenge lies in the fact that NIST’s approach fails to account for the diverse array of regulatory guidelines within financial institutions. Over the past decade, a significant convergence of regulatory requirements has emerged within financial services, encompassing model risk management, third-party risk management (TPRM), and cybersecurity.
What sparks the formation of a Financial Services (FinServ) firm? Firms are advised by EY to revisit their existing instrument portfolio. To ensure regulatory compliance, companies should focus on three key aspects.
-
A comprehensive framework integrating mannequin danger administration, third-party risk management (TPRM), and cybersecurity.
-
Document detailing the entirety of our organization’s artificial intelligence components and properties, inclusive of machine learning models and training data developed thus far.
- A framework for monitoring and tracking the efficacy of AI methodologies, focusing on high-impact applications that require scrutiny.
As GenAI evolves rapidly, so too do the risks associated with it. The National Institute of Standards and Technology (NIST) has recently released guidance on mitigating risks associated with Generative Artificial Intelligence (GenAI). Bhuva, a top expert at EY, sounded an alarm about a critical threat: the propensity of AI models to fabricate information.
The expert emphasized that while everyone might use the term “hallucination,” the National Institute of Standards and Technology (NIST) focuses specifically on the anthropomorphic attribution of human-like qualities to artificial intelligence. While the idea was that hallucinations might make AI seem overly human-like. To account for their arrival at this point, they coined the term confabulation. I’ve rarely come across anyone using the term confabulation in everyday conversation. It appears that many people are misled by misinformation, yet that’s available for purchase.
Developing a comprehensive plan for accountable AI advancement is a complex task that requires the integration of multiple factors, ensuring seamless coordination and rigorous testing to guarantee its efficacy. For a thorough approach, organizations must integrate multiple discrete components, encompassing model risk management, regulatory compliance, data security, and business continuity. Achieving collective cooperation towards a shared goal poses a significant challenge, according to Bhuva.
“To ensure the optimal outcome, you must confirm that relevant events are properly arranged.” As the technology advances, the potential for sophistication will increase significantly due to the diverse range of experiences and complexities inherent in generative AI.
Have you effectively addressed all controls relevant to generative AI, with verification completed for privacy, Total Productive Risk Management (TPRM), information governance, and model risk management fields?
Ethics surrounding artificial intelligence are an entirely distinct consideration. Since moral guidelines for AI are typically unenforceable under the law, individuals must decide for themselves whether to refrain from using large language models that have been trained on copyrighted material, notes Bhuva. Is using a language model trained on copyrighted material morally justifiable even when the supplier indemnifies your organization against potential copyright infringement lawsuits?
“One major issue is that when focusing on global AI regulations, all prominent standards emphasize privacy, equity, transparency, accuracy, and security – every guideline,” said Bhuva. Although multiple guidelines may appear to be in conflict, it’s crucial to ensure the accuracy of your model by gathering substantial data. However, this process may inadvertently violate privacy requirements. You are likely sacrificing explainability for accuracy as well. The sheer complexity surrounding the regulation of AI raises significant questions about its ethical implications, prompting widespread interest in navigating these issues effectively.
Implementing Accountable GenAI
At EY, Kiranjot Dhillon, a seasoned AI expert, offered a practitioner’s perspective on responsible AI to the audience.
One of the many significant challenges that GenAI practitioners currently face is determining precisely how GenAI techniques will actually perform in operational settings, according to Dhillon.
“According to her, a critical factor is incorporating accountable AI and risk mitigation strategies throughout the entire solution-building lifecycle.” “It’s crucial to ensure that essential requirements are thoroughly incorporated from the outset, rather than hastily addressing them with additional retrofits later.”
While attempting to integrate readability features into your system, you may find it challenging to retroactively inject this functionality once the data has been collected. She emphasized that starting early produces far superior results.
“Achieving accountable necessities requires careful consideration from the outset,” said Dhillon. As toll gates are identified, they must be navigated through a series of subsequent steps, including operationalization and obtaining approvals at each stage.
Because the foundation of generative AI techniques has been established, it’s crucial for developers and designers to define the key performance indicators and standards they aim to achieve, ensuring that their AI systems meet predetermined goals and expectations. Since the system relies on both accountable AI team members and possibly problematic “purple teams” to intervene and assess whether actual needs are being fulfilled.
Dhillon spearheads the development of transparent GenAI methods by incorporating consumer-oriented safeguards. These techniques, similar to those employed by Nvidia’s Nemo, can operate at both the input and output stages of a Large Language Model (LLM). They will prevent certain requests from being directed at the Large Language Model (LLM), and direct the LLM to refrain from responding in certain manners.
“When discussing sensitive topics, it’s essential to consider setting boundaries or ‘guardrails’ to ensure that the conversation remains focused and relevant,” Dhillon emphasized. While seeking to mitigate hallucinations or confabulations as defined by NIST’s framework, it’s crucial to establish robust security and safety safeguards that anchor our responses firmly on the ground. Stemming answers from external sources and ensuring they do not pose a risk by controlling the supply chain effectively. Recognizing and addressing these potential pitfalls is precisely the purpose that guardrails serve.
The ideal safeguard for Large Language Models (LLMs) is often a secondary LLM designed to monitor and correct primary LLM outputs, ensuring logical coherence, response relevance, and contextual accuracy – key considerations in the Responsible AI Group (RAG) arena, according to Dhillon.
Some of the greatest practices for using instant engineering responsibly include? While traditional machine learning relies on information to train a model, GenAI’s approach often involves providing explicit instructions for the computer to follow, according to Dhillon.
She emphasizes the importance of defining a clear task and objectives when utilizing Large Language Models, underscoring the need for precise instruction to achieve desired outcomes. In reality, simplicity reigns supreme in this situation. Be descriptive. Inasmuch as we endeavor to engage in a most illuminating exchange with the Large Language Model (LLM), I hereby stipulate that its responses shall be forthcoming only when posed with questions of substance, replete with intellectual curiosity and a penchant for erudite inquiry. Any queries phrased in a tone lacking in rigor or sophistication, such as those framed in an overly simplistic or trite manner, shall forthwith elicit no response whatsoever from the LLM, thereby precluding any potential tedium or pedantry that might arise from its indulgence in frivolous or vapid discourse.
The robustness of a generative AI’s outcome varies significantly depending on the type of prompt employed, including zero-shot, few-shot, and chain-of-thought prompting techniques.
To ensure the GenAI system operates safely and effectively, it’s crucial to maintain human oversight throughout its deployment. By leveraging a visualization software that incorporates automated statistical methods to group multiple responses, individuals can swiftly identify anomalous or outlier trends, thereby streamlining the process of anomaly detection and subsequent data analysis.
“When evaluating this concept, a human expert would likely identify similarities between the items within the bean-shaped cluster and those in the database, suggesting potential connections with similar queries.” As you move further away from the core cluster, you’re likely to encounter queries with indirect connections. The most direct and effective route to the lower left corner yields a group of questions that, upon careful review of the instruction manual, are found to contain profanity. The vast disparity lies in the unanticipated realm beyond the confines of expected output, rendering the LLM’s response incongruous with anticipated results. “And thereby, design triggers capable of initiating manual interventions when necessary.”
Watch Bhava and Dhillon’s complete presentation, plus the archived session on HPC.
Artificial Intelligence takes over Wall Street’s trading desks, registering seamlessly at 0.01 nanoseconds.