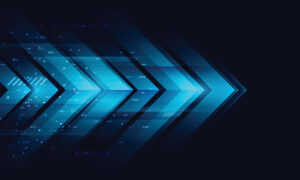
(DongIpix/Shutterstock
As companies delve into building and managing generative AI capabilities, they’re finding that one crucial element is essential for success: a robust vector database. The primary reason driving widespread adoption of this specific type of database is…
While the fervent excitement surrounding General Artificial Intelligence (GenAI) seems unwarranted at first glance, significant interest still surrounds this emerging technology.
According to a recent Boston Consulting Group survey, IT leaders anticipate significant advancements in various machine learning applications over the next 12 months; similarly, KPMG’s March report predicted a surge in investments in Generative Artificial Intelligence (GenAI) within the same timeframe.
The momentum driving GenAI fuels an energized curiosity around vector databases as well. According to database trackers at , vector databases have reigned supreme as the preferred choice for the past 13 months.
The vector database pattern shows no signs of slowing down. Estimated that 30% of companies will leverage vector databases driven by foundational fashion advancements by 2026, a significant increase from the mere 2% adoption rate in 2022.
As demand surges, the database industry is adapting by increasing production of vector capabilities, including standalone vector databases and multimodal databases that seamlessly integrate vectors across diverse data types.
As various trade-offs exist between these two types of vector databases, a promising trend is emerging towards multimodal architectures. By 2026, a significant shift in the database landscape is expected, as a recent study reveals that an astonishing 75% of traditional databases, encompassing both relational and NoSQL varieties, will integrate vector capabilities into their offerings.
“According to Forrester’s report by lead analyst Noel Yuhanna, certain organizations opt for these databases due to their capacity to integrate diverse data types, facilitate hybrid search and build upon existing infrastructure.” As the database landscape continues to evolve, many multimodal solutions now provide vector capabilities at no extra cost, further amplifying their appeal to organizations.
Several factors influence a buyer’s decision to employ either a multi-model database or a local vector database. According to Forrester, if the application demands “exceptional processing power and ultra-low latency for seamless access to vast vector data,” a vector database may indeed be the suitable solution.
Differences in usage scenarios can sway a customer’s decision-making process, favouring one option over another. Conventional databases shine in powering complex functions, generating reports, and driving enterprise intelligence, whereas native vector databases are tailored to support cutting-edge artificial intelligence, advanced search, and seamless data retrieval capabilities in the Age of Augmented Intelligence.
With extensive access to high-dimensional, cutting-edge data, a buyer can leverage a local vector database to unlock even greater capabilities. Native vector databases excel at handling unstructured data types, including text documents, images, videos, and audio files, by efficiently indexing complex information and seamlessly integrating with machine learning tools.
Despite some limitations, a standard database still offers several key benefits to its users? In line with Forrester’s principles, these solutions aim to facilitate transactional processing, diverging from the local vector database concept. Typically, they boast even stronger support for integration with third-party tools. To efficiently store and retrieve data, consider leveraging a traditional relational database, which offers a reliable foundation for querying information; in contrast, native vector databases typically interact with applications via application programming interfaces (APIs). Multimodel databases occupy a middle ground regarding their benefits and drawbacks.
“Not unlike traditional databases, which prioritize exact matches on formatted data, vector databases truly shine when tasked with conducting exceptional similarity searches on complex, multidimensional data.” For illustration, a vector database can swiftly identify all images within its repository that resemble a specified photograph with remarkable speed, accomplishing this feat through the instant evaluation of vectors in mere seconds. The unique advantage of vector databases stems from their ability to support customised vector indexes, enabling rapid query processing and delivering the high performance necessary for complex information retrieval.
Native vector databases enable clients to efficiently store, index, and search vector embeddings, as Forrester emphasizes. Native vector databases leverage advanced indexing and hashing techniques, such as Ok-dimensional trees, hierarchical navigable small-world graphs, locality-sensitive hashing, Facebook AI’s similarity search algorithm, and graph-based indexes, to achieve superior performance.
Among the most prevalent applications of vector databases are RAG, picture similarity searches, optimizing suggestion engines, personalizing customer experiences, detecting anomalies, powering search engines, and identifying fraudulent activities. Forrester recommends tailoring solutions to individual client needs by leveraging either local vector databases or multi-model databases, depending on the unique requirements of each use case.
When seeking rapid access to enormous datasets of vectors – tens of terabytes in scale – consider leveraging a localized vector database, the company advises. “Notwithstanding, when your applications require combining vector and non-vector data, consider partnering with a multi-model database that offers vector data capabilities.”
While scalability and efficiency dominate discussions around native versus multi-model architectures, lingering doubts persist regarding the performance of vector databases at scale.
“Forrester’s research reveals that most vector databases have not yet demonstrated high-end scalability and efficiency, particularly when handling billions of vectors or vast amounts of terabytes of data.” “To maximize performance, ensure that vector processing employs optimized indexing, precision-crafted search mechanisms, and strategic utilization of Graphics Processing Units (GPUs) and scalable architecture where applicable.”