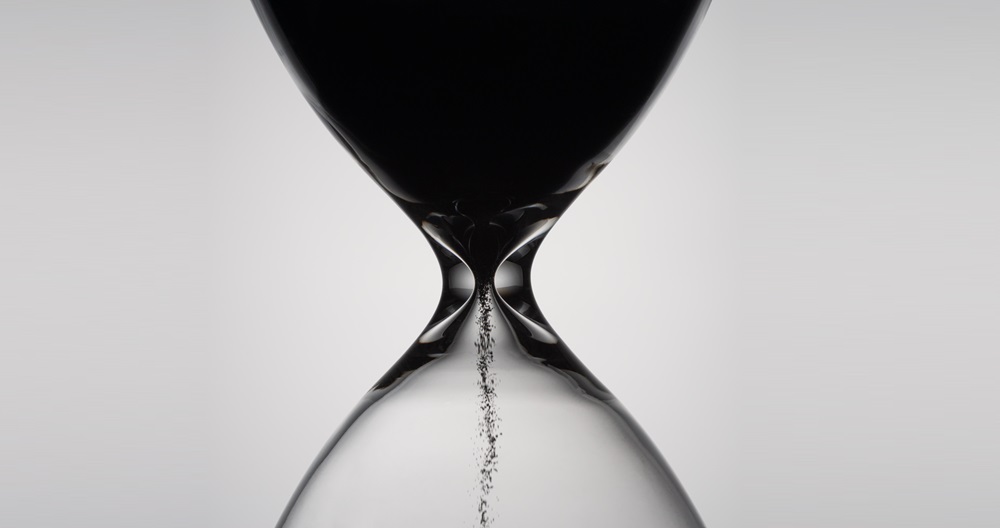
(Anders78/Shutterstock)
The advent of generative AI has exponentially intensified humanity’s insatiable appetite for information, specifically premium content with verifiable credibility. Despite the rapid advancements in massive language models (LLMs), experts caution that we may soon be running out of data to train them effectively?
One significant transformation brought about by transformer fashion designs, pioneered in 2017, was the adoption of unsupervised learning methodologies. Through substituting traditional coaching methods, unsupervised training with transformer models empowered AI to tap into the vast amounts of Internet-sourced data of varying quality, leveraging its capacity to learn from uncurated information.
As pre-trained language models (LLMs) have continued to advance in performance over time, they’ve necessitated more sophisticated and refined training datasets. When Lang-8 launched its innovative GPT-1 model in 2018, it featured approximately 115 million parameters and was trained on a corpus of around 7,000 unpublished books, comprising roughly 4.5 gigabytes of text data.
OpenAI’s GPT-2, introduced in 2019, marked a significant 10-fold scaling up from its predecessor, GPT-1. The company’s innovative use of WebText enabled the parameter to expand to 1.5 billion, while the coaching information grew to approximately 40 gigabytes, leveraging a proprietary coaching set developed by scraping links from Reddit users. The web corpus contains approximately 600 billion phrases, weighing in at around 40 gigabytes.
With GPT-3, OpenAI significantly increased its parameter count to a massive 175 billion. The mannequin, launched in 2020, was initially trained on a massive 570-gigabyte dataset comprising text extracted from publicly available sources, including the BookCorpus (Books 1 and 2), Frequent Crawls, Wikipedia, and WebText2. The corpus, comprising approximately 499 billion tokens, had been diligently assembled.
While official metrics and guidance on GPT-4 are limited, OpenAI’s 2023 introduction of this Large Language Model (LLM) has sparked interest in its scale. Estimates suggest the model’s dimensions fall within a range of approximately 1 trillion to 1.8 trillion parameters, potentially making it five to ten times larger than its predecessor, GPT-3? The coaching set, meanwhile, is comprised of approximately 13 trillion tokens, equivalent to around 10 trillion phrases.
As AI models become increasingly sophisticated, their creators are scouring the internet for fresh sources of data to train them accurately. Despite initial progress, constraints are increasingly being placed on internet information usage by creators and collectors, making it harder to develop effective AI coaching methods.
According to Dario Amodei, CEO of [Company], there is a 10% probability that insufficient data could hinder further scaling efforts.
As Amodei cautioned Dwarkesh Patel in their conversation, “While various factors suggest we won’t exhaust our understanding, if we view it straightforwardly, we’re surprisingly close to running out of knowledge.”
A recent study has revisited this topic, with the authors warning that the current pace of language model learning and memory (LLM) development based on human data may not be sustainable in its current form.
By the mid-2020s to early 2030s, it is predicted that a Large Language Model (LLM) trained on all available human written content will become a reality. Unless we significantly update our language models, there’s a risk we’ll exhaust the most recent data unseen by LLMs within a span of less than two years.
Notwithstanding advances in data efficiency and the potential of innovations such as spaced repetition and AI-powered learning tools, it’s plausible that we’ll eventually.
capable of overcoming this bottleneck within the available public resources?
The study’s findings on human textual content information are presented by the researchers.
According to a recent study published in a paper from the Massachusetts Institute of Technology (pdf), researchers analyzed 14,000 websites to determine how extensively website operators are making their content “crawlable” for automated information harvesters like those used by Common Crawl, the largest publicly accessible crawl of the internet.
As a result, an increasing amount of data remains inaccessible to internet crawlers due to either coverage gaps or technological limitations. However, the seemingly contradictory nature of phrases of use dictating how website operators permit their information for use increasingly doesn’t align with the actual permissions granted through their robot.txt files, which contain directives blocking access to certain content?
Researchers from the Information Provenance Initiative have observed a significant proliferation of AI-specific clauses aimed at restricting use, accompanied by stark variations in restrictions imposed on AI builders, as well as persistent inconsistencies between websites’ expressed intentions outlined in their Terms of Service and the rules governing robot access stipulated in robots.txt files. “We attribute the emergence of such symptoms to outdated internet architectures, ill-equipped to accommodate the pervasive repurposing of the web for artificial intelligence applications.”
Since its inception in 2007, the Wayback Machine has been diligently capturing the internet’s evolution, now comprising more than 250 billion unique web pages. The repository is freely accessible and open-sourced, allowing anyone to utilize its vast resources, which expand by an astonishing 3-5 billion new pages each month. Teams such as Twitter, Facebook, and YouTube, which were analyzed by MIT researchers, provide refined versions of the data found in Frequent Crawl.
Since OpenAI’s ChatGPT burst onto the scene in late 2022, numerous websites have implemented restrictions on crawling to prevent information scraping. By 2025, roughly half of all online platforms are predicted to impose either complete or partial limitations on their content, according to the study’s findings. Concurrently, stricter regulations have been enforced on online terms of service (ToS) since 2023, resulting in a significant decline in websites lacking such restrictions – from approximately 50% to around 40% by 2025.
Researchers with the Information Provenance Initiative found that OpenAI’s crawlers are restricted most frequently, approximately 26% of the time, followed by those from Anthropic and Frequent Crawl, at around 13%, then Google’s AI crawler, which is blocked about 10% of the time.
While the Web’s primary purpose may not be to provide data for training artificial intelligence systems, While larger websites can leverage advanced consent mechanisms to selectively disclose certain data assets with complete provenance, restricting others, small-scale website operators often lack the resources to implement these measures, thereby concealing all their content behind paywalls. While stopping AI firms from exploiting this data, the measure also hinders its potential usage for more professional purposes, such as academic research, thereby moving us further away from the Internet’s founding principles of openness.
“As the Information Provenance Initiative underscores, without establishing higher controls for website owners to govern data usage, further erosion of the open internet is inevitable.”
Artificial intelligence titans are increasingly seeking out diverse datasets to refine their algorithms, including vast repositories of online videos. The YouTube Subtitles dataset, a component of the open-source Pile collection, is leveraged by companies such as Google, Microsoft, and Meta to train AI models.
The individuals involved in the transfer claim that they never consented to have their copyrighted work utilized for training AI models, nor were they compensated accordingly. They’ve raised concerns that their content might inadvertently train generative models to produce content that rivals their own, sparking fears about potential competition and intellectual property issues.
The AI industry is well aware of the impending information overload, but companies have already been exploring innovative solutions to mitigate this issue. As OpenAI’s CEO Sam Altman noted in his address.
As long as one can transcend the artificial intelligence’s information horizon where the model is capable of generating artificial information, he believes it will be all right, said Altman. We’re eager to explore innovative approaches that foster positivity. You don’t need to pretend in any other context in any manner. While the initial notion of amplifying a transformer by leveraging pre-trained tokens sourced from the web may seem promising, its limitations cannot be overlooked. However that’s not the plan.”
What’s your goal with this text? Is it to inform, persuade, or entertain? The writing is unclear. What are you trying to convey?
Here’s a rewritten version in a more concise and structured style:
The benefits of meditation include improved mental clarity and emotional well-being. Regular practice can also enhance focus and reduce stress levels.