To train a robot in basic instrument usage, start by teaching it simple techniques for handling tools like hammers, wrenches, and screwdrivers. This will enable the robot to quickly learn how to perform minor repairs around the house. To successfully utilize a device, one would require an unrestricted abundance of knowledge showcasing its functionality.
Presented robotic datasets exhibit significant variations in modality, comprising diverse formats such as image arrays and tactile patterns. Knowledge is also gathered from various domains, including simulations and human demonstrations. Every dataset may seize upon a unique process and environment.
To develop a robust machine-learning model, it’s challenging to integrate information from multiple sources seamlessly, thereby leading many approaches to utilize a solitary type of data for training a robot. Although robots proficiently tackle tasks utilizing a relatively limited amount of domain-specific expertise, they can occasionally struggle to adapt and complete novel responsibilities in uncharted settings.
Researchers at MIT have designed a methodology for integrating diverse sources of knowledge across domains, modalities, and tasks, leveraging the power of generative AI diffusion models in an effort to coach higher multipurpose robots.
They employ a distinct diffusion model to learn a specific technique or coverage for completing a task using a unique dataset. Companies merge the insurance policies generated by fashion models into a comprehensive coverage, enabling robots to perform multiple tasks across diverse environments.
Through simulations and real-world experiments, this innovative coaching approach allowed a robot to successfully execute various tool-based tasks and seamlessly transition to novel responsibilities unseen during training. The implementation of the Coverage Composition (PoCo) strategy yielded a notable 20% enhancement in process efficiency relative to baseline methods.
Addressing heterogeneity in robotic datasets poses an inherent dilemma? To effectively implement comprehensive robotic insurance frameworks, we initially require deployable robots that can efficiently absorb and utilize vast amounts of knowledge? According to Lirui Wang, an EECS graduate student and lead developer, “Integrating diverse knowledge pools, much like researchers have accomplished with ChatGPT, is a pivotal step forward for the robotics field.”
Wang’s co-authors consist of Jialiang Zhao, a mechanical engineering graduate student; Yilun Du, an electrical engineering and computer science (EECS) graduate student; Edward Adelson, the John and Dorothy Wilson Professor of Brain and Cognitive Sciences at MIT; and senior author Russ Tedrake, the Toyota Professor of Electrical Engineering and Computer Science, Aeronautics and Astronautics, and Mechanical Engineering. The robotics industry will be showcased at the forthcoming Robotics: Science and Techniques Convention, with experts offering insights and analysis throughout the event.
A robotic agent is a machine learning model that utilizes inputs to perform a task or action. Considered as a technique to measure software testing coverage. In the context of a robotic arm, this technique can manifest as a precise trajectory – a calculated sequence of poses that enables the arm to accurately pick up a hammer and employ it to drive a nail into place.
Traditional datasets for teaching robotic insurance policies were often limited and focused on a single, specific task and environment, such as packaging devices into containers within a warehouse setting?
While individual robotic warehouses produce massive amounts of data, this information remains isolated and proprietary to each specific setup handling those packages alone? “It’s hardly impressive,” Wang says, “if you want to apply all that expertise to train a simple AI.”
Researchers at MIT have created a methodology capable of training separate insurance policies for individual sequences of smaller datasets – such as those collected from multiple robotic warehouses – and combining them in a way that enables robots to generalise effectively across various tasks.
By employing a type of generative AI model known as a diffusion model, they represent all coverage. Techniques from the field of diffusion models are typically employed in image generation tasks, where they are trained to produce novel data examples that closely resemble those present within a provided training dataset through iterative refinement of their predictions.
Rather than instructing a diffusion model to generate images, the researchers trained it to produce a motion plan for a robotic system. To augment their coaching dataset, they introduce random noise to the trajectories. The diffusion model’s manifold gradually eliminates noise and refines its predictions into a coherent trajectory.
This system, dubbed by its creators, was previously launched by researchers at Massachusetts Institute of Technology, Columbia University, and the Toyota Research Institute. Building upon the foundational research of Diffusion Coverage, PoCo advances our understanding…
Staff trains each diffusion model with datasets resembling those featuring human video demonstrations and another derived from the teleoperation of a robotic arm.
The researchers combine the individual insurance policy outcomes from each diffusion model, iteratively refining the results to ensure the blended coverage meets the objectives of each unique policy.
“One notable benefit of this approach is that it enables us to combine different insurance policies to reap the benefits of each, thereby creating a comprehensive coverage solution.” Wang notes that a coverage expertise trained on real-world data can acquire additional dexterity, whereas one trained on simulations can gain further generalization capabilities.
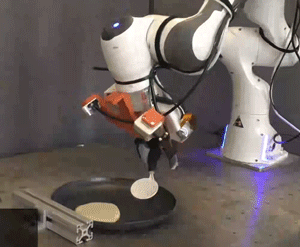
Picture: Courtesy of the researchers
Due to their unique design, individual insurance policies can be combined and tailored to achieve better results for a specific process. Consumers can further augment their expertise by training an additional diffusion model on a novel dataset, rather than initiating the entire process anew.
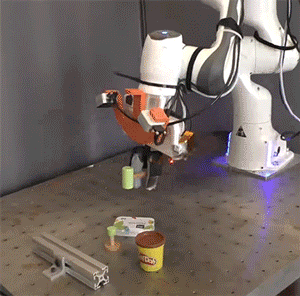
Picture: Courtesy of the researchers
Researchers simulated and tested the Pick-and-Place Operation (PoCo) capability on various robotic arms, mimicking tasks such as driving a virtual hammer to strike a digital nail or deftly flipping objects using a simulated spatula. Process Cycle Optimization (PoCo) resulted in a 20% enhancement in process efficiency compared to baseline methods.
“When asked about their experience, Wang notes, ‘After fine-tuning and visualizing our results, we were able to see a marked improvement in the composite trajectory, which far outperformed its individual components’.”
Researchers will eventually need to adapt this approach to tackle complex tasks where a robot selects and utilizes multiple tools in sequence. To further boost their performance, they must also leverage larger robotics datasets to optimize their operational effectiveness.
To achieve successful robotics development, we require a trifecta of expertise: leveraging web-based information, honing simulation skills, and acquiring hands-on experience with actual robots. What’s the secret formula to blending these ingredients? “NVIDIA’s AI Brokers Initiative Chief Praises PoCo as ‘Stable Step Heading in Right Direction'”, notes Jim Fan, Senior Analysis Scientist.
This research is supported in part by Amazon, the Singapore Ministry of Defence’s Protector Research Office, and the United States Department of Defense. The collaboration between Nationwide Science Foundation and the Toyota Research Institute.