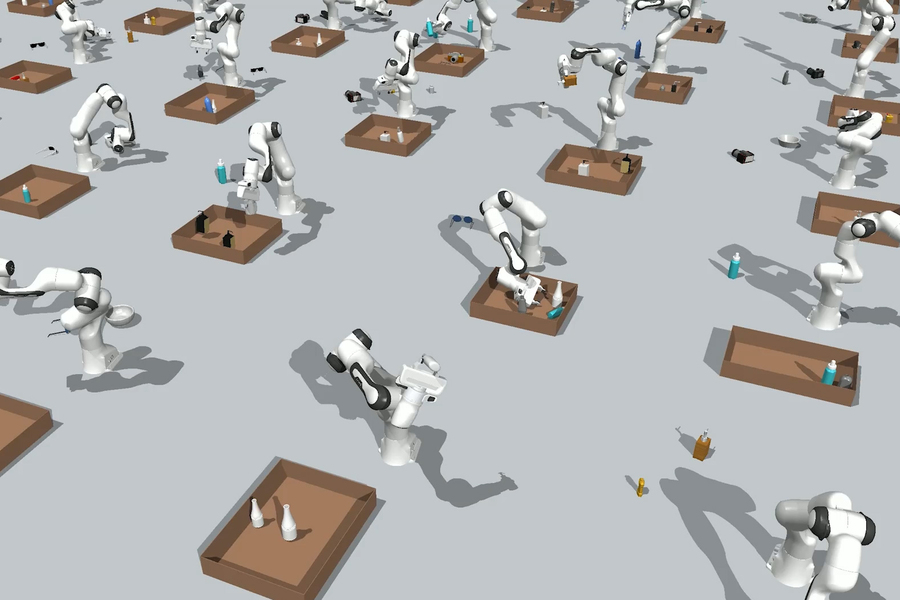
Researchers at MIT are harnessing the power of generative artificial intelligence frameworks to enhance robotic dexterity in tackling complex object manipulation tasks, such as efficiently packing a box with diverse items.
Anyone familiar with the frustration of packing a large amount of luggage into a small car trunk understands that it’s an arduous challenge. Robots also grapple with the complexities of dense packing, a task that requires precision and dexterity.
Ensuring efficient packaging for robots necessitates addressing multiple constraints, much like carefully arranging luggage to prevent suitcases from spilling out of the trunk, ensuring that heavier items don’t rest atop lighter ones, and avoiding collisions between the robotic arm and the vehicle’s bumper.
Conventional approaches address this limitation by employing a step-by-step strategy, proposing a partial solution that satisfies one requirement at a time, and subsequently verifying whether all other constraints have been breached? The potential for an overwhelming task lies in the sheer volume of steps to complete and luggage to prepare, making it a realistically daunting endeavour.
MIT researchers leveraged the capabilities of a generative AI, specifically a diffusion model, to tackle this challenge with greater efficacy. Using a suite of machine-learning models, each trained to embody a distinct type of constraint, they develop their methodology. Fusing diverse fashion elements enables the creation of global packaging solutions that seamlessly accommodate all limitations, ensuring timely resolution.
Their approach yielded efficient options at a pace surpassing alternative methods, simultaneously producing a more extensive portfolio of profitable opportunities within the same timeframe. Notably, their methodology demonstrated the ability to address challenges arising from novel combinations of constraints and increased object counts, which were not encountered during training.
By leveraging their findings’ generalizability, researchers can apply their method to educate robots on recognizing and fulfilling universal packing constraints, like minimizing collisions or ensuring that one item is placed after another in a sequence. Robots trained using this method could potentially be deployed for a broad range of complex tasks across diverse settings, from automating inventory management in a warehouse to tidying up a bookshelf in someone’s home.
“My vision is to challenge robots to tackle increasingly complex tasks with multiple geometric constraints and more nuanced decision-making – a crucial aspect of service robotics, where machines must operate effectively in unpredictable and diverse human environments.” With the highly effective tool of compositional diffusion techniques, we will now tackle these complex challenges and achieve impressive generalization results, remarks Zhutian Yang, an electrical engineering and computer science graduate student and lead author of a study.
His co-authors enthusiastically endorse a talented trio: Jiayuan Mao and Yilun Du, both esteemed MIT graduate college students; Jiajun Wu, a renowned assistant professor of computer science at Stanford University; and the accomplished Joshua B. At MIT’s Division of Mind and Cognitive Sciences, Professor Tenenbaum is a distinguished academician. Alongside Tomás Lozano-Pérez, an esteemed professor of computer science and engineering, they share membership with the renowned Pc Science and Synthetic Intelligence Laboratory (CSAIL). Additionally, Leslie Kaelbling, as the Panasonic Professor of Computer Science and Engineering at MIT, brings her expertise to the fold as a senior creator and member of CSAIL. The proposed analysis of the Convention on Robotic Learning will likely be presented at the upcoming event.
Constraint issues
Robotic systems often struggle with steady constraint satisfaction. Issues appear to arise in multi-step robotic manipulation tasks, such as packing objects into a field or setting a dining table. The robotic arm’s movement is governed by various constraints, encompassing geometric restrictions that prevent collisions with its surroundings; physical limitations that ensure stacked objects remain stable; and qualitative conditions that dictate tasks like placing a spoon beside a knife in the exact manner required.
Constraints exist in varying degrees, influenced by the complex interplay between object geometries and human-defined requirements that ebb and flow across different scenarios and environments.
To address these challenges successfully, the MIT team employed a cutting-edge machine-learning methodology dubbed Researchers employ diffusion-based methods to simulate novel data points that mirror those found in a training set through an iterative refinement process, generating synthetic data that approximates the original distribution.
To achieve this, diffusion fashion studies a process for refining existing solutions by introducing subtle improvements. Initially, they respond with a haphazard, extremely unwholesome solution that gradually gets refined.
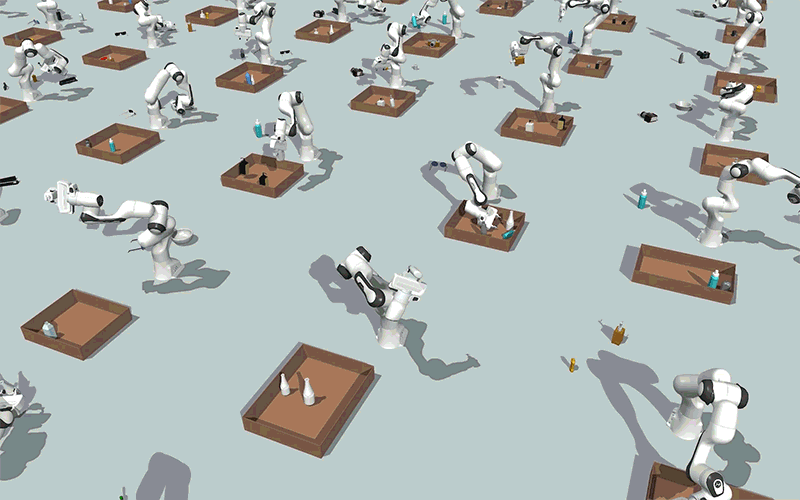
Researchers at MIT leveraged generative AI capabilities to develop a solution enabling robots to efficiently address persistent constraint satisfaction challenges, such as precisely packing objects within a container while minimizing collisions, as demonstrated through this simulation.
Imagine a jumbled assortment of plates, bowls, and utensils scattered across a virtual workspace, allowing the various components to intersect and converge in unexpected ways. Collisions between objects are prevented by the constraint of non-interference, causing them to nudge each other away. In contrast, qualitative constraints exert a subtle influence, drawing the plate to its central position, aligning the salad fork with the dinner fork, and so forth.
Because diffusion fashions can effectively combine the influences of multiple styles to drive the pose of one object towards satisfying all constraints, they are well-suited for this type of steady constraint-satisfaction problem, according to Yang’s explanation. By exploring a diverse range of initial estimates each time, the fashion industry can access a wide array of refined choices.
Working collectively
The researchers sought to capture the intricate relationships between the constraints in their study on Diffusion-CCSP. When preparing luggage, one limitation might necessitate a particular item being placed after another, while a separate restriction could dictate where specific items should be positioned.
The Diffusion-CCSP model learns a diverse portfolio of diffusion patterns, each tailored to address a specific type of constraint. Fashion trends develop through collective learning, facilitating the exchange of knowledge and insights, much like the efficient arrangement of physical objects in packing geometry.
The fashion algorithm collaborates to identify feasible arrangements of objects, taking into account the constraints and finding collective solutions that meet all requirements.
It takes multiple attempts to arrive at a solution. However, when refining an answer and a few violations occur, it is likely to lead you to a more accurate response. One misstep can have far-reaching consequences, she cautions.
By tailoring coaching strategies to individual constraints and then aggregating them to produce predictions, a significantly smaller volume of training data is needed compared to other methods.
Notwithstanding the need for extensive knowledge, coaching fashion entrepreneurs still necessitates access to a significant volume of case studies and resolved problems. Researchers seeking to rectify each shortcoming with traditional slow methods find the cost of compiling such data prohibitively expensive, according to Yang.
Researchers developed a novel approach that started by creating multiple options, then refined the method to suit users’ needs. Utilizing expedient algorithms, the team created compartmentalized bins that seamlessly accommodated a diverse range of 3D objects, ensuring efficient packing, stable configurations, and collision-avoidant arrangements within each section.
“With this course of study, information technology is almost instantly accessible through simulation.” “We’re poised to create tens of thousands of environments where we’re well-equipped to tackle long-standing challenges,” she remarks.
Utilizing these informations, the diffusion models collaborate seamlessly to determine optimal object placement areas for the robotic gripper, effectively accomplishing the packing task while respecting all constraints.
They conducted feasibility research, subsequently showcasing the capabilities of Diffusion-CCSP by utilizing an actual robotic system to tackle various complex challenges, including transforming 2D triangles into a field, packing 2D shapes with spatial relationship constraints, stacking 3D objects while ensuring stability, and packing 3D objects using a robotic arm.
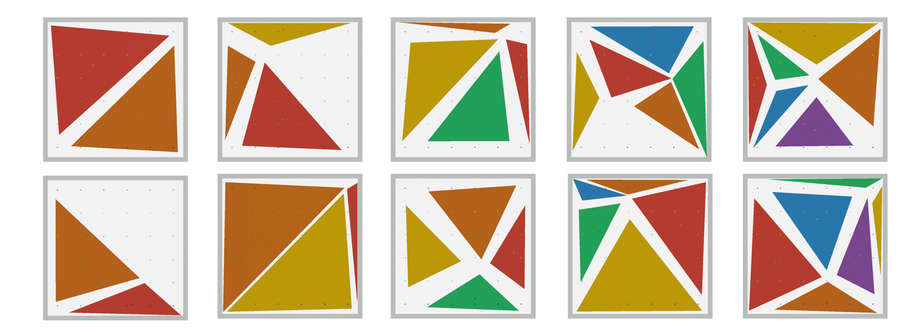
This example illustrates instances of two-dimensional triangle packing. These are collision-free configurations.
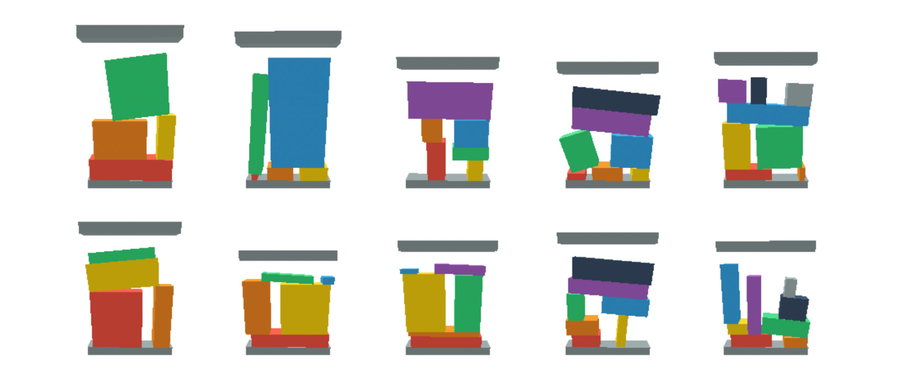
This algorithm determines 3D object stacking configurations that meet stability constraints. Studies suggest that at least one entity is sustained by multiple entities.
Their methodology excelled across numerous trials, yielding a diverse array of reliable and collision-avoiding solutions.
As their research evolves, Yang and her team will likely seek to validate the efficacy of Diffusion-CCSP under more complex scenarios, such as those involving robots navigating a room? Additionally, they aim to empower Diffusion-CCSP to address challenges across multiple domains without requiring retraining on fresh data.
“Danfei Xu, an assistant professor in the College of Interactive Computing at the Georgia Institute of Technology and Research Scientist at NVIDIA AI, notes that Diffusion-CCSP is a machine-learning approach that leverages current highly effective generative models.” As the system rapidly generates options, it simultaneously satisfies multiple constraints by combining predefined individual constraint patterns. While still in its nascent stages of refinement, ongoing advancements in this technology retain the potential to facilitate more sustainable, secure, and reliable autonomous systems across diverse applications.
The analysis was funded by a diverse coalition of organizations, including the Nationwide Science Foundation, Air Force Office of Scientific Research, Office of Naval Research, MIT-IBM Watson AI Lab, MIT Quest for Intelligence, Center for Brains, Minds, and Machines, Boston Dynamics Artificial Intelligence Institute, Stanford Institute for Human-Centered Artificial Intelligence, Analog Devices, JPMorgan Chase & Co., and Salesforce.
MIT Information