Microsoft is positioned as a Leader in the 2022 Gartner Magic Quadrant for Cloud AI Development Platforms. Azure AI provides a robust, flexible end-to-end platform for accelerating artificial intelligence and machine learning innovation.
Microsoft has been named a Leader in the 2022 Gartner Magic Quadrant for Cloud AI Developer Services. Provides a robust, adaptable end-to-end platform for expediting advancements in data science and machine learning while delivering the corporate governance every organization requires in the era of AI.
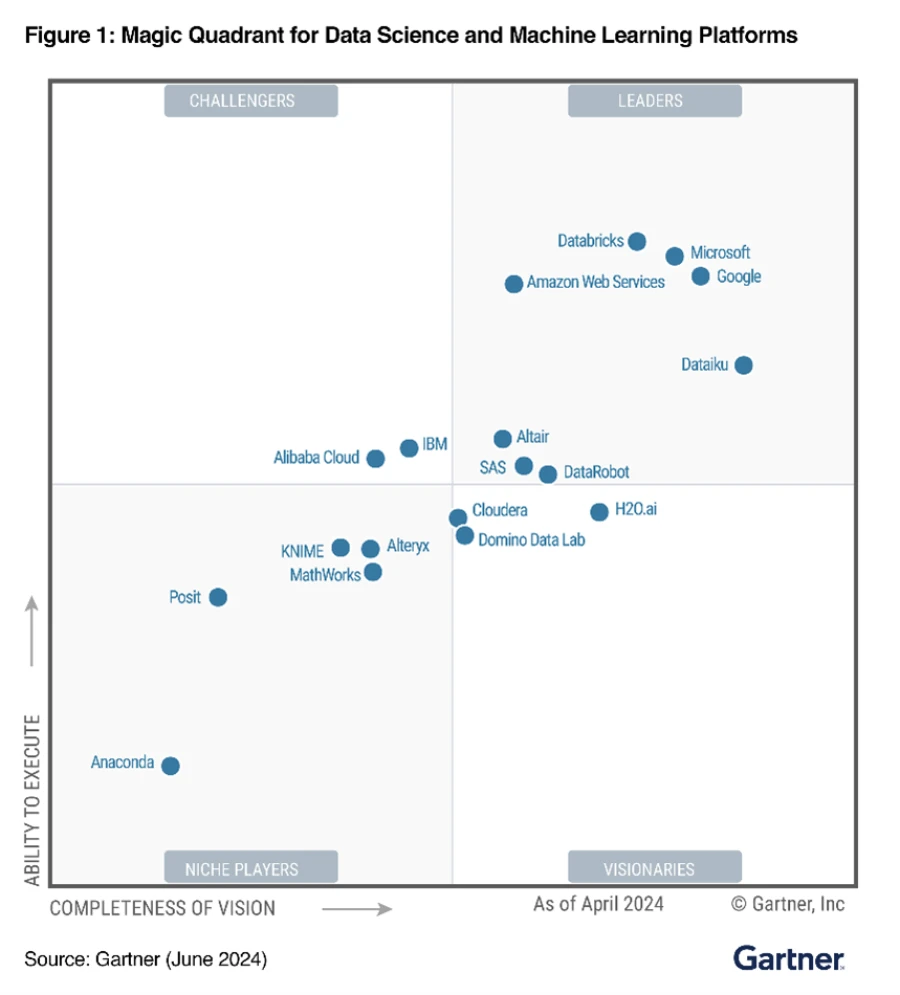
By May 2024, Microsoft had been recognized as a Leader for the fifth consecutive year in a row, according to Gartner, where it ranked highest for its Completeness of Vision. We’re thrilled by these recognitions from Gartner as we continue to serve a diverse range of clients, from large-scale enterprises to innovative startups, helping them successfully integrate and deploy their AI and machine learning models and capabilities within manufacturing environments in a secure and scalable manner.
Azure AI leads the way in purpose-designed AI infrastructure, responsible AI toolkits, and facilitating seamless collaboration among diverse teams through Machine Learning Operations (MLOps) for both generative AI and traditional machine learning projects. Supplies entry to a diverse range of pre-built fashion options within the Azure AI model catalog, including current releases of BERT, DistilBERT, and RoBERTa, as well as tools to fine-tune or build your own machine learning models. Moreover, the platform offers access to a vast, curated library of open-source frameworks, tools, and algorithms, empowering data science and machine learning teams to innovate freely on a trusted foundation.
Accelerate Time-to-Worth with Azure AI Infrastructure?
“.”
Dr. Nico Wintergerst, an AI Analysis Engineer at
Azure Machine Learning enables organisations to rapidly develop, deploy and manage high-calibre AI solutions, leveraging capabilities that span building large models from the ground up, executing inference on pre-trained models, consuming models as-a-service, and refining models for specific industries. Azure Machine Learning runs on the same infrastructure that powers some of the world’s most popular AI services, including ChatGPT and Bing. Furthermore, the seamless integration of Azure Machine Learning with ONNX Runtime and DeepSpeed enables customers to significantly optimize training and inference times, resulting in greater efficiency, scalability, and energy efficiency.
Regardless of whether your team is training a deep learning model from scratch using open-source frameworks or deploying an existing model in the cloud, Azure Machine Learning enables data science teams to scale out training jobs by leveraging elastic cloud computing resources and seamlessly transitioning from training to deployment. With our solution, clients can seamlessly deploy high-performance workloads on powerful CPU and GPU machines, freeing them from managing the underlying infrastructure complexities. Similarly, clients do not need to provision or manage underlying infrastructure when utilizing a model from the Azure AI model catalog. Clients can seamlessly deploy and manage thousands of models across various manufacturing settings – from on-premise to edge locations – for both batch and real-time predictive analytics.
Automate machine learning workflows efficiently through optimized MLOps and LLMOps strategies.
“.”
Fabon Dzogang, a senior machine learning scientist at
MLOps and LLMOps intersect at the confluence of human capital, workflow efficiency, and technological infrastructure. As knowledge science tasks evolve to incorporate more complex functions and scaling, the need for efficient automation and collaboration tools becomes increasingly crucial for achieving high-quality, repeatable results.
Azure Machine Learning serves as a robust MLOps platform, designed to support machine learning workflows of all sizes. The platform simplifies group collaboration by allowing seamless sharing and governance of machine learning assets, streamlines development through integrated interoperability with Azure DevOps and GitHub Actions, and enables real-time monitoring of model performance in production environments. Innovative information connectors leveraging Microsoft sources akin to those found in Snowflake and Amazon S3, thereby streamlining MLOps workflows.
Additionally, this solution seamlessly enables knowledge scientists to scale their current workloads from native execution to the cloud and edge, while centralizing all MLflow experiments, run metrics, parameters, and model artifacts within a single, easily accessible workspace.
Streamlining the comprehensive enhancement loop for generative AI functions, LLMOps leverages its capabilities to coordinate executable workflows comprising fashions, prompts, APIs, Python code, and tools for vector database lookups and content filtering. Azure AI Immediate Stream enables developers to seamlessly integrate their experimental workflows with standard open-source frameworks such as LangChain and Semantic Kernel, allowing them to scale complex evaluations quickly and efficiently. Developers can collectively troubleshoot, share, and refine functions using in-built testing, tracing, and analytics tools, ensuring seamless integration and continuous evaluation of code quality and security standards. Then, with a single click, builders can deploy functions once prepared, while monitoring key metrics such as latency, token utilization, and technological quality in real-time manufacturing. What reliable outcomes stem from comprehensive monitoring and continuous refinement?
Designing ultra-reliable fashion trends and applications that seamlessly integrate with users’ daily lives.
“.”
—Teague Maxfield, Senior Supervisor at
AI regulations akin to those governing equity, security, and transparency rarely function autonomously. That’s why Azure Machine Learning provides data scientists and engineers with intelligent tools to operationalize responsible AI throughout their workflow, whether they need to assess and debug a conventional machine learning model for bias, protect a base model from instant injection attacks, or monitor model accuracy, quality, and security in production?
The tool enables knowledge scientists to evaluate and troubleshoot traditional machine learning models for fairness, precision, and interpretability throughout the machine learning lifecycle. Customers can create a detailed document to share model efficiency metrics with business stakeholders, enabling more informed decision-making. In Azure Machine Learning, builders can equally assess model cards and benchmarks, performing their own evaluations to select the most suitable foundation model for their specific use case from the array of available options. To proactively mitigate the risks associated with AI, they will employ a multi-layered approach, harnessing the power of built-in safeguards, real-time monitoring capabilities, and swift engineering adjustments. At scale, builders can continuously assess, refine, and document the impact of their mitigation strategies through a seamless integration with built-in and customizable metrics in real-time. Knowledge science teams can confidently deploy solutions while providing transparency to enterprise stakeholders.
Learn extra on .
Enterprise-wide Safety, Privateness, and Compliance?
“.”
As Michael Calvin, Chief Technical Officer at
As we navigate today’s data-driven landscape, effective knowledge security, governance, and privacy demands that every team possesses a comprehensive grasp of their data and AI/machine learning methodologies. Effective governance of AI necessitates seamless collaboration among diverse stakeholders, including IT directors, AI and machine learning engineers, data scientists, and risk and compliance professionals. With Azure Machine Learning, organizations can ensure knowledge and models are safeguarded and compliant with the highest standards of security and privacy through enabling enterprise observability by MLOps and LLMOps.
With Azure Machine Learning, IT directors can restrict access to resources and operations via consumer accounts or teams, monitor incoming and outgoing network communications, encrypt data both in transit and at rest, detect vulnerabilities through continuous scanning, and centrally manage and audit configuration policies across. Information governance groups can leverage Azure Machine Learning to automatically register metadata on AI assets – including models, datasets, and jobs – directly to the Microsoft Purview Information Map. Knowledge scientists and knowledge engineers can thereby scrutinize the sharing and reusability of elements, as well as the lineage and transformations of coaching knowledge, to comprehend the intricacies of dependencies and their far-reaching implications. Similarly, threat and compliance experts can leverage observations of coaching methodologies, fine-tuning or expanding best practices, and their applications across various manufacturing processes to inform compliance reports and audit findings.
With Azure Arc now enabled, organisations can effortlessly deploy AI and machine learning workloads across all their Kubernetes clusters, ensuring seamless data residency, security, and compliance for hybrid cloud and on-premises scenarios. Here is the rewritten text:
This feature enables organisations to track where data resides, meeting stringent regulatory requirements while maintaining flexibility and control over their MLOps. Clients leveraging the combination of Azure Machine Learning and other tools can develop highly effective models on diverse data sources without requiring manual data transfer or replication from secure locations.
Can you imagine harnessing the power of AI to revolutionize your business? With Azure Machine Learning (AML), you can unlock new insights and opportunities by training machine learning models on a massive scale.
Here’s a step-by-step guide to get started:
1\. Create an AML workspace: Begin by setting up your Azure account, then create an AML workspace where you’ll store and manage all your machine learning projects.
2\. Choose the right data source: Identify the dataset that best represents your business problem or opportunity. You can import data from various sources, such as Excel files, CSVs, or even databases.
3\. Prepare your data: Ensure your data is clean, processed, and ready for training. You might need to perform tasks like data augmentation, feature engineering, or handling missing values.
4\. Build and train a model: Use AML’s intuitive drag-and-drop interface, Jupyter Notebooks, or Python libraries (like TensorFlow or PyTorch) to build and train your machine learning models.
5\. Deploy and manage your model: Once you’ve trained your model, deploy it as a RESTful API, a web service, or integrate with popular platforms like Power Apps or Stream Analytics. Monitor its performance and make updates as needed.
6\. Integrate with other Azure services: Leverage AML’s seamless integration with other Azure services, such as Azure Databricks for data processing or Azure Functions for real-time predictions.
7\. Continuously improve your model: Refine your model by iterating on the training process, experimenting with different architectures, and incorporating new data.
As the digital landscape evolves, machine learning is revolutionizing how businesses operate and compete – whether optimizing internal processes, enhancing customer interactions, or pioneering new technologies; a robust, flexible machine learning and data science platform empowers responsible AI innovation.
Magic Quadrant for Information Science and Machine Learning Platforms, Gartner, June 17, 2024, Authors: Afraz Jaffri, Aura Popa, Peter Krensky, Jim Hare, Raghvender Bhati, Maryam Hassanlou, Tong Zhang.
Gartner has released the Magic Quadrant for Cloud AI Developer Providers, a report authored by Jim Scheibmeir, Arun Batchu, and Mike Fang, available as of April 29th, 2024.
The GARTNER name and logo are registered trademarks and service marks of Gartner, Inc. and/or their affiliates within the U.S. The Magic Quadrant is a registered trademark of Gartner, Inc., globally recognized. This information is owned by XYZ Inc. and/or its associates and is used herein with permission of XYZ Inc. All rights reserved.
Gartner neither endorses nor recommends any vendors, services, or products featured in its research reports, nor advises clients to select only those providers with top ratings or designations. Gartner analysis publications encompass the opinions of Gartner’s Analysis & Advisory group and shouldn’t be construed as statements of reality. Gartner disclaims all warranties, express and implied, including those related to merchantability and fitness for a particular purpose, with respect to this analysis.
The graphic, published by Gartner, Inc., was… What is the main goal or objective of this larger document? The Gartner document is available upon request.