
Throughout the latter half of the 1960s, I held a ham radio license; it was during this period that I witnessed the significant technological shift from vacuum tube-based systems to transistorized ones. In our reality, the allowance to operate high-power transmitters with 1,500-watt outputs enables vacuum tubes to remain functional for extended periods, significantly longer than in other environments. There’s a compelling reason: tubes prove to be the most effective high-power tools for those who are unaware of their capabilities, or who possess just enough knowledge to cause unintended harm. Can you get them scorching hot enough to sufficiently soften the interior components? While this may seem to imply a significant amount of room for mistakes,
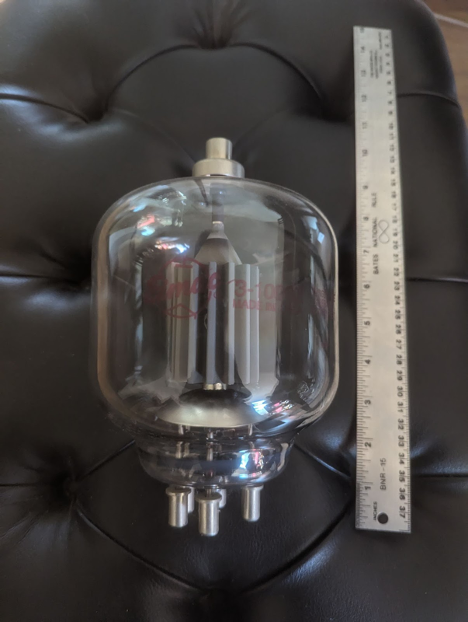
Transistors are the other. For just a millionth of a second, exceeding a transistor’s specifications can prove fatal, often leading to permanent destruction. When comparing components to athletes, tubes resemble skilled soccer players and transistors echo talented dancers – both possess remarkable stability and efficacy, yet if they falter, a severe injury can occur? As a result, a significant disparity exists between high-powered tube tools and their transistor-based counterparts. To effectively cool a vacuum tube, a fan should be placed adjacent to it. To effectively dissipate the heat generated by a tiny transistor producing 500 watts, it’s crucial to employ a robust copper-based heat spreader, a substantial thermal management platform, and multiple fans. The core components of a tube amplifier consist of a high-energy power source, a large vacuum tube, and a carefully designed output circuitry. A transistor amplifier boasts a comprehensive suite of features, including sophisticated computer systems, advanced sensors, and numerous electronic components designed to seamlessly detect and rectify any anomalies or potential flaws, ensuring seamless operation. Many adjustments formerly executed by manual knob-twiddling have now been streamlined through automation. While embracing automation may seem like a luxury, in reality, it’s an imperative. Without manual intervention, excessive usage would reduce the lifespan of the transistors, resulting in an earlier failure to broadcast effectively.
Be taught sooner. Dig deeper. See farther.
The software program has undergone a seamless transformation. In the earliest stages of the internet’s development, creating a simple website was relatively straightforward: basic HTML coding, minimal JavaScript functionality, standard CSS formatting, and server-side processing using CGI scripts. As technology continues to evolve, purposes have become increasingly sophisticated, incorporating complex combinations of backends with databases, middleware, and intricate frontend frameworks that are now an integral part of our digital landscape. Violent attacks, defying the fundamental principles of humanity, have become increasingly prevalent and alarming. Observability lies at the foundation of a “transistor-like” approach to building software, serving as the initial step in this methodology. To effectively anticipate and address potential problems, it is crucial to accumulate a sufficient body of relevant knowledge that enables you to foresee challenges before they escalate into full-blown crises; merely collecting information for the purpose of conducting a retrospective analysis alone is insufficient.
As we chart a new course, the stakes rise exponentially with the advent of AI. This year, we will witness widespread integration of artificial intelligence into various applications. Artificial intelligence presents numerous novel challenges for both builders and IT professionals to address. Here’s a start to our listings:
- Whether intentionally or merely for entertainment purposes, users will inevitably need to manipulate an AI system into producing incorrect results. You can expect racist, misogynistic, and utterly false responses. These seemingly disparate elements will ultimately converge to reveal a cohesive set of key strategic priorities for your organization.
- A critical review of AI systems revealed that they can inadvertently or intentionally compromise customer data, posing significant security risks across various domains.
- Significant advancements in linguistic frameworks enable the automatic generation of software code for computer programs. That code is incessantly insecure. It’s possible that attackers might use an AI-powered tool to generate malicious code at their behest, further complicating cybersecurity efforts.
- Innovation: Fashion trends may eventually lose their appeal, requiring a reboot to stay relevant. There’s no evidence to suggest that giant language models are an exception? Although languages evolve gradually, the topics that require your dummy to be well-versed in will remain constant.
- While initial efforts to develop AI-driven projects may begin to yield results through court proceedings, it is likely that creators of such technology will ultimately face legal accountability for copyright infringement.
- Distinct legal liabilities: The trend towards legislation on privacy and transparency is slowly gaining momentum, with Europe leading the charge in this regard. As global AI adoption accelerates, the United States must reconcile its stance on AI regulations with emerging international standards.
That’s a . While my expertise doesn’t require me to meticulously account for every potential weakness, the intricacy of the situation increasingly renders in-person oversight impractical. For centuries, the monetary system has been a cornerstone of economic activity, with its principles and practices being extensively studied. Algorithmic buying and selling methods require continuous monitoring, prompting swift intervention upon detection of any anomaly. However, a fundamental flaw exists: they should be equipped with computerized “circuit breakers” that automatically shut down the strategy if persistent errors occur; manual override capability must also exist in case these alternative approaches fail. Without the safeguards, the outcome could potentially seem like a company whose algorithmic trading software generated $1 million in profits on its first day.
The AI industry hasn’t yet fully leveraged the expertise of others; instead, it’s rapidly evolving and creating problems as fast as it’s making the transition from relatively simple software – such as a large React-based frontend with an enterprise backend – to complex software that integrates many more processing nodes, whose inner workings are not yet fully understood, and which can cause harm at scale. Like a cutting-edge high-power transistor amplifier, this sophisticated software programme has reached an unprecedented level of complexity and delicacy, necessitating expert guidance to avoid catastrophic failure. Isn’t it unclear how we collectively grasp the skills necessary for building the automation required to tackle AI tasks? With the rapid evolution of automation technologies, developing effective strategies for constructing these methodologies will become a top priority in the coming years.