For roughly five years, I’ve closely tracked advancements in computer vision (CV) and image synthesis research on ArXiv and other platforms. As a result, I’ve noticed that breakthroughs become increasingly apparent with the passage of time, and they often take new trajectories each year.
As 2024 approaches, it seems pertinent to explore innovative trends in ArXiv submissions related to PC Vision and Image Recognition. While these observations may be informed by extensive knowledge and experience, they remain anecdotal in nature.
As of 2023, a notable trend emerged in the voice synthesis literature, with an overwhelming majority of publications originating from China and other parts of East Asia. By the end of 2024, anecdotal evidence suggests that this phenomenon has also started to impact the picture and video synthesis analysis landscape.
China and its neighboring countries do not consistently produce flawless results; indeed, there are some instances where this is the case. Moreover, it overlooks the substantial probability in China (similar to the West) that many of the most impressive and effective new growth programs remain proprietary, thereby being excluded from academic literature.
Despite this, east Asia is reportedly outperforming the West in terms of volume, as recommended. The value that something holds ultimately hinges on the degree to which its feasibility is thoroughly evaluated and deemed viable; typically, this process fails to yield meaningful results.
In the realm of generative AI, distinguishing between challenges that can be addressed through refinements to existing architectures versus those that require a complete overhaul from scratch is not a straightforward endeavour?
While research from East Asia may dominate the landscape of PC vision papers, I’ve noticed a surge in ‘Frankenstein’-inspired projects – namely, repurposed amalgamations of existing work, often featuring limited novel architecture and frequently relying on altered data sources.
This year, a vastly expanded array of East Asian collaborations, primarily involving Chinese institutions, appeared to prioritize quotas over merit, significantly increasing the noise-to-signal ratio in an already oversubscribed field.
Meanwhile, I’ve also been impressed by a wider range of East Asian publications that caught my attention in 2024. If that’s all a numbers game, it’s not a failure in itself, yet it doesn’t necessarily equate to a low-cost option either.
The global output of papers has unmistakably surged in 2024, with a noticeable increase across all countries of origin.
The preferred publication date consistently fluctuates throughout the year. As it stands at present, Tuesday sees around 300 to 350 submissions to the Pc Imaginative and prescient and Sample Recognition section, with this number peaking during the May-August and October-December periods, corresponding to convention seasons and annual quota deadlines.
Beyond my own expertise, ArXiv itself undergoes a surge, with over 6,000 entirely new submissions, and the PC Vision component being the second-most submitted part, following closely behind Machine Learning.
Notwithstanding the classification of Machine Learning on ArXiv often serves as a comprehensive category, this trend suggests that Computer Vision and Object Detection may be the most prolific ArXiv submission categories, surpassing Machine Learning in terms of submissions.
Aristotle’s archives decidedly dominate physics and computer science, as the unmistakable leader in submissions.
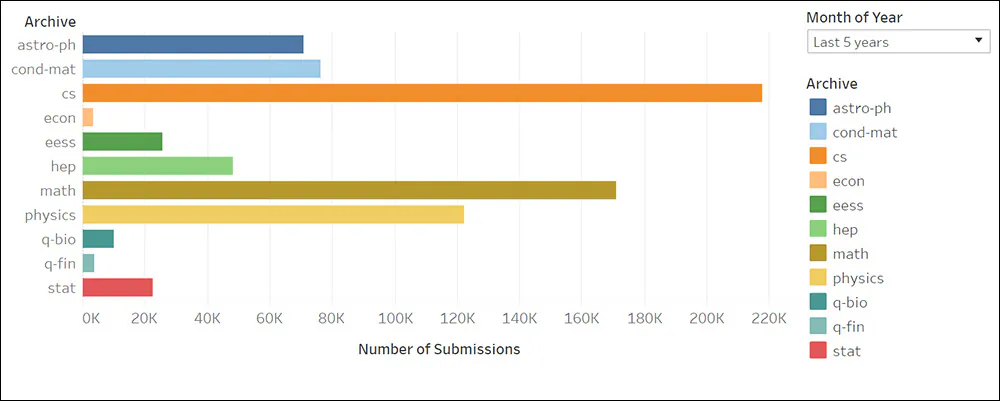
Supply: https://data.arxiv.org/about/experiences/submission_category_by_year.html
Notwithstanding Stanford College’s limitations in reporting the latest statistics, it is noteworthy that there has been a significant surge in the submission of academic papers on machine learning in recent years.
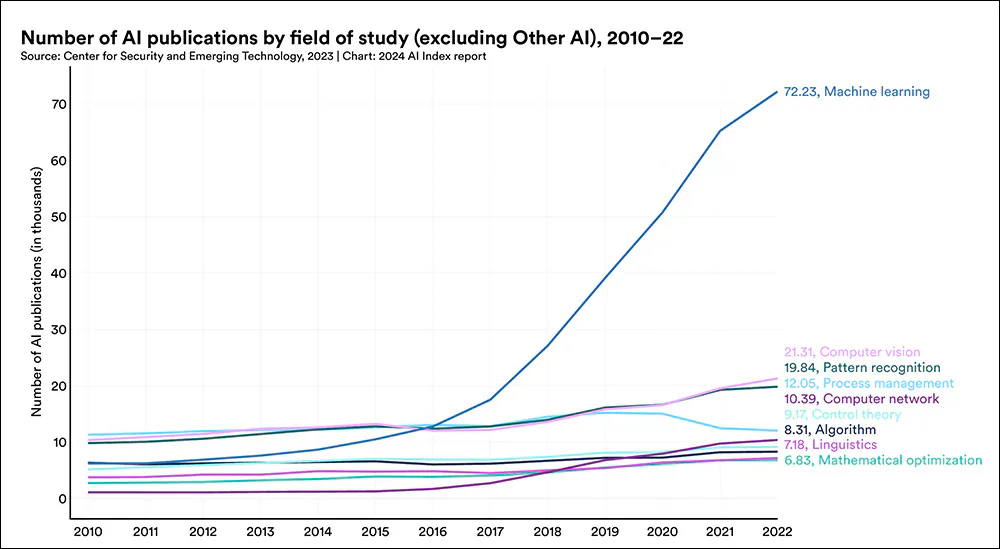
Supply: https://aiindex.stanford.edu/wp-content/uploads/2024/04/HAI_AI-Index-Report-2024_Chapter1.pdf
A notable shift is the proliferation of research papers focused on leveraging data-driven methods (LDMs) to create innovative, mesh-based visuals akin to traditional computer-generated imagery (CGI).
Initiatives like these exemplify Tencent’s commitment to innovation, collaboration, and strategic partnerships, further solidifying its position as a leader in the industry.
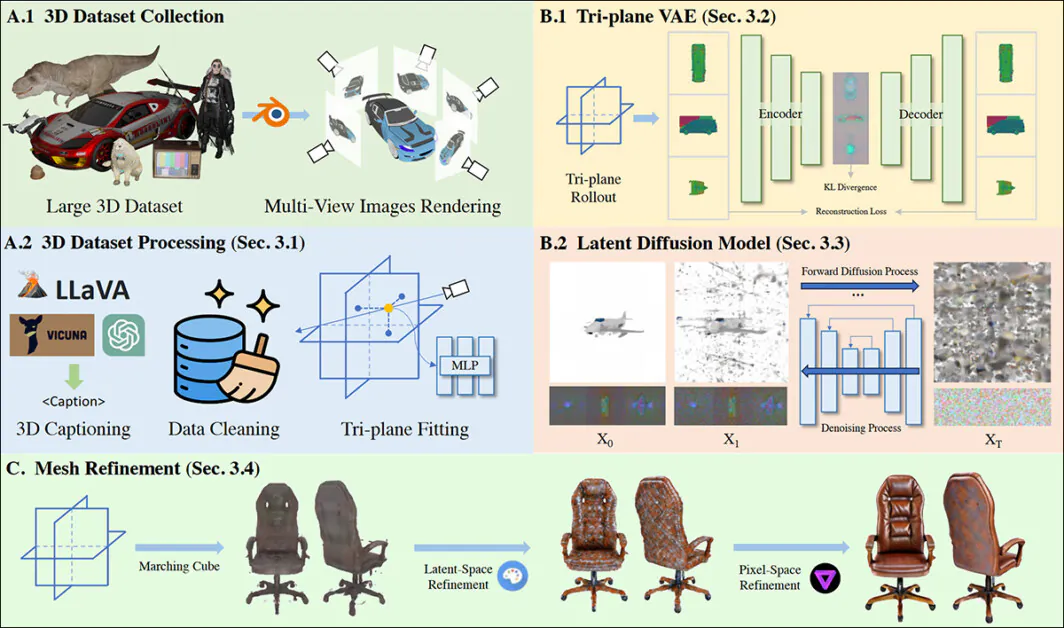
Supply: https://arxiv.org/pdf/2403.02234
This emergent analysis strand may very well be taken as a tacit concession to the continuing intractability of generative programs akin to diffusion fashions, which solely two years had been being touted as a possible substitute for all of the programs that diffusion>mesh fashions at the moment are in search of to populate; relegating diffusion to the position of a instrument in applied sciences and workflows that date again thirty or extra years.
Stability.ai, pioneers of open-source modeling, have just unveiled a groundbreaking technology that seamlessly integrates an AI-generated image with NeRF interpretation, creating a bridge to produce an express, mesh-based CGI model. This innovative solution can be applied in various platforms requiring explicit 3D coordinates, including Unity, video games, augmented reality, and others.
In the realm of generative AI, distinct lines are drawn between two-dimensional and three-dimensional implementations of image and vision generation, respectively. While three-dimensional objects are typically comprised of faces, these faces do not necessarily involve calculations of addressable 3D coordinates in every situation.
The GANs, widely employed in 2017-era deepfake architectures, among other applications, are capable of accommodating all these strategies.
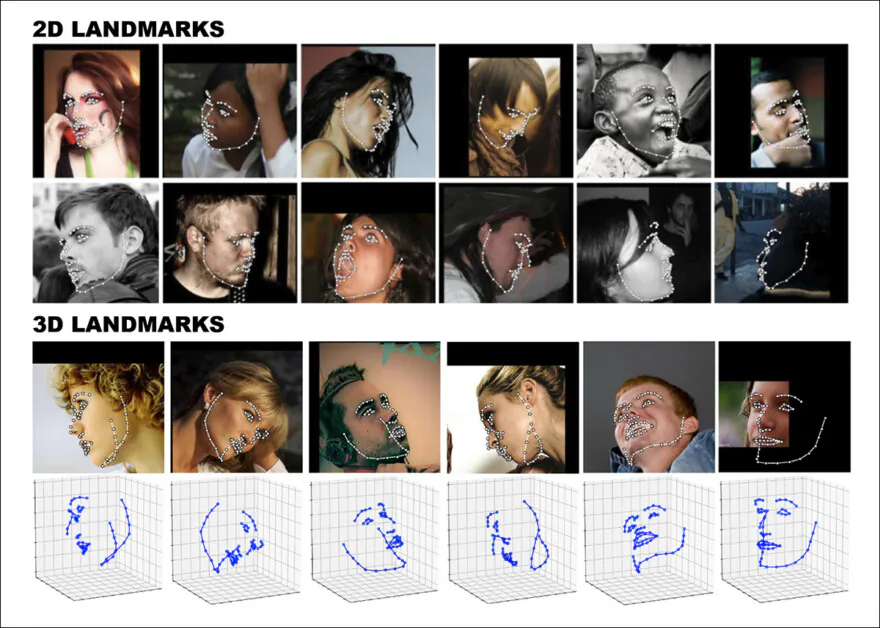
Supply: https://github.com/1adrianb/face-alignment
As the term “deepfake” has evolved into a complex concept in computer vision analysis, so too has “3D” become a multifaceted term in the field of computer vision.
For customers, 3D often signifies stereo-enabled media, where viewers need specific glasses; for professionals, it denotes the distinction between 2D paintings (conceptual sketches) and mesh-based models manipulable in software like Maya or Cinema4D.
In PC vision, the concept of “existence” simply implies that an object has been detected and localized within the confines of the model – that is, it can be addressed or directly manipulated by a user; at least, not without employing third-party interpretive CGI-based programs like Blender or Maya.
While the concept of “kind of picture” remains inexact, it’s worth noting that not only can images serve as input to generate a computer-generated imagery (CGI) model, but the term ‘mesh’ is more accurately used to describe this process?
Despite this, diffusion aimed to transform the supply picture into a mesh as part of most burgeoning projects. A more accurate description would be:
However, this is a challenging pitch to present during a board meeting or in a marketing campaign aimed to engage investors.
Despite drawing comparisons to 2023, the latest batch of research suggests an escalating sense of urgency surrounding the mitigation of diffusion-driven technological advancements.
What’s hindering progress is the challenge of maintaining narrative and temporal coherence within videos, ensuring consistency in character and object appearances not only across diverse clips but also within a single, short video sequence.
The final epochal innovation in diffusion-based synthesis was introduced in 2022. While recent advancements, such as Flux, have effectively addressed several key limitations, including Steady Diffusion’s inability to generate textual content within a produced image, and overall picture quality has seen notable improvement; it appears that most papers I reviewed in 2024 primarily focused on rearranging existing concepts rather than introducing meaningful innovation.
Earlier stalemates have arisen with Generative Adversarial Networks (GANs) and Neural Radiance Fields (NeRF), both of which initially showed great promise but failed to live up to expectations. This phenomenon also appears to be happening with diffusion models.
By the end of 2023, it became apparent that the rasterization method (3DGS), which first emerged as a medical imaging approach in the early 1990s, was poised to swiftly surpass human picture synthesis challenges such as facial simulation, recreation, and identity switching.
The 2023 ASH paper delivered on its promise, surpassing the performance of autoencoders and other competing methods, while also achieving impressive cross-validation results.
While this year’s progress in 3D-generated synthetic humans may have been underwhelming, with most research building upon previous achievements rather than pushing boundaries further, the limited scope of recent breakthroughs has yielded few substantial advancements.
To mitigate this issue, significant focus has been placed on enhancing the fundamental buildability of 3DGS, which has led to a proliferation of research studies offering enhanced 3DGS exterior settings. Researchers have received funding to explore the intersection of Simultaneous Localization and Mapping (SLAM) technologies with three-dimensional geographic surveying (3DGS) methodologies, mirroring efforts seen in initiatives such as , , , and numerous other comparable projects.
Several notable efforts have explored the potential of splat-based human synthesis, including, but not limited to, the pioneering work of, the innovative approaches developed by, the ambitious projects undertaken by, and the recent breakthroughs achieved by. While other events took place, none of them came close to the profound impact of the groundbreaking research published in late 2023.
In South East Asia and China, analysis often relies on case studies with potentially controversial or sensitive content, making them unsuitable for republishing in an assessment article.
The proliferation of research papers on generative AI has sparked concerns over the depiction of women and young individuals in mission scenarios; a trend that has persisted for nearly two years, with many studies featuring scantily-clad models or women in their early 20s as default examples. Borderline NSFW examples of this include graphic medical illustrations, risqué advertisements, and even very dry papers akin to the (FVMD), albeit with subtle undertones.
As the latest advancements in Latent Diffusion Models (LDMs) have unfolded, online communities and subreddits have coalesced around these innovations, with the notorious phenomenon of Rule 34 persistently thriving.
While such instances of inappropriate overlap may occur, it is crucial to acknowledge that they coincide with a growing understanding that AI systems should not recklessly exploit famous individuals’ likenesses – particularly in academic research that unthinkingly employs attractive celebrities, often female, in dubious scenarios.
The series features a unique blend of youthful anime-inspired female characters, alongside liberal references to iconic celebrity identities such as Marilyn Monroe’s and contemporary stars like Ann Hathaway – the latter having publicly disavowed this type of usage.
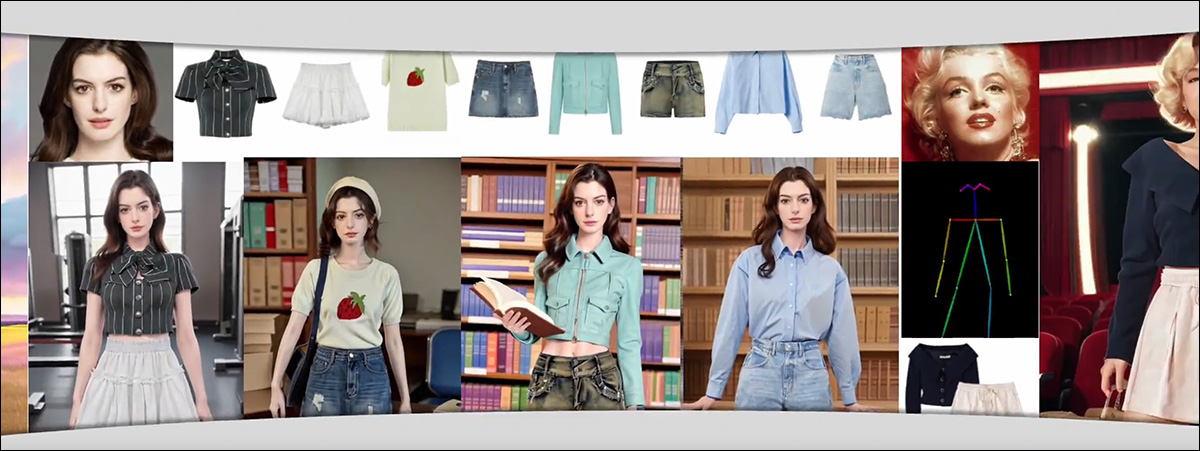
Supply: https://crayon-shinchan.github.io/AnyDressing/
While explicit AI applications have seen a notable decline throughout 2024, this trend is largely attributed to the major breakthroughs from prominent players like FAANG and cutting-edge research institutions such as OpenAI. Cautious of the legal implications that could arise from portraying realistic characters, major gaming companies are increasingly hesitant to depict photorealistic individuals.
Despite the capabilities of their creations being reminiscent of and , Western generative AI initiatives have shifted their focus towards producing “cute”, Disneyfied, and heavily protected images and videos.
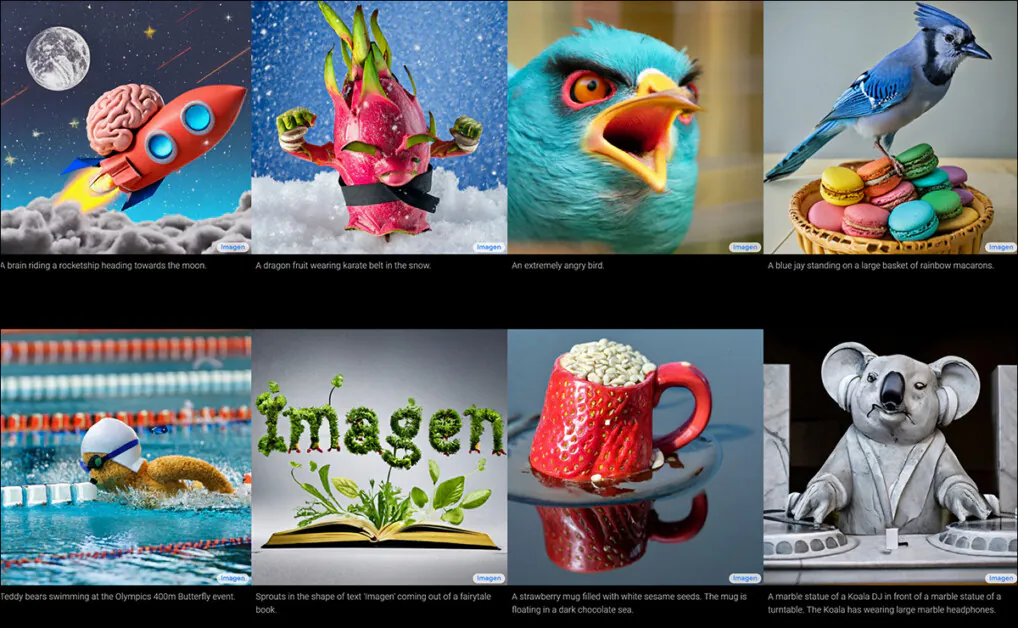
Supply: https://imagen.analysis.google/
In Western computer vision literature, one notable yet deceptive approach stands out: methods that produce consistent representations of a particular individual across multiple exemplars, such as LoRA and its predecessors.
Examples embody complexities, Google’s search algorithms, and a technical mess.
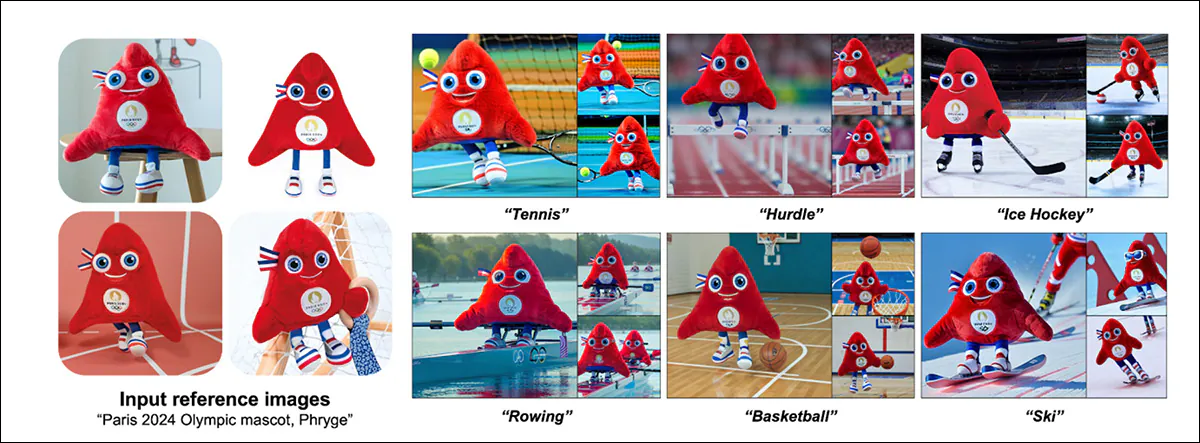
Supply: https://websites.google.com/view/instructbooth
Notwithstanding the emergence of the “cute instance” phenomenon, disparate CV and synthesis analysis strands across various initiatives, such as those exemplified by,, and, among numerous others, have conceded to more realistic consumer expectations in their offerings.
The proliferation of user-generated content platforms, akin to those that enable residents with relatively modest hardware to create programs, has precipitated a surge in the availability of free, downloadable movie-style fashion models and neighborhoods. While illicit use persists, its accessibility stems from open-sourced architectures like and.
While it’s generally feasible to bypass content restrictions in generative text-to-image and video programs by exploiting their protection options, a significant gap remains between the limited capabilities of top-tier models like RunwayML and Sora, and the virtually unlimited potential of underperforming algorithms and native deployments.
While proprietary and open-source programs differ, they share a common threat: becoming equally ineffective. T2V programs risk being hamstrung by concerns about litigation, while lack of licensing infrastructure and dataset oversight in open-source programs may bar them from the market altogether as regulations tighten.