The landscape of natural language processing is undergoing a transformative shift with the advent of deep learning. Researchers at Meta have developed a pioneering structural framework that revolutionizes the traditional reliance on tokenization in massive language models (LLMs), thereby clearing the path for more efficient and robust language processing. As the clock strikes dawn, a new era dawns on the prospect of token change, necessitating a comprehensive understanding of the BLT’s core features, advantages, and far-reaching consequences for shaping its future trajectory.
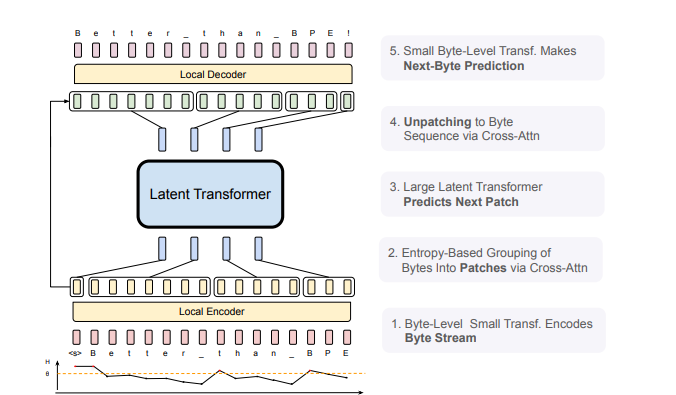
The Tokenization Downside
Tokenization has been a fundamental component in preparing text data for language model training, transforming raw text into a structured set of tokens. Despite its potential benefits, this technique inherently poses several significant constraints.
- Tokenization can exacerbate disparities across various languages, often disproportionately benefiting those with more robust token structures.
- Fastened tokens fiercely contend with noisy or variant inputs, risking a degradation in model efficacy that can compromise their overall performance.
- Conventional tokenization often neglects subtle linguistic nuances that are crucial for comprehensive understanding.
Introducing the Byte Latent Transformer
The BLT tackles these obstacles by performing linguistic analysis at the byte level, thereby obviating the need for a predefined lexicon. Utilizing an unconventional approach, the system employs a unique mechanism that categorizes team members’ bytes based on their intricacy and forecastability, gauged through proprietary metrics. By doing so, the mannequin optimizes its computational resources and concentrates on regions where in-depth comprehension is essential.
Key Technical Improvements
- The Bit-Level Transformer (BLT) breaks down bytes into customised patches according to the intricate nuances of their data composition, thereby optimising computational efficiency.
- :
- Converts byte streams into patch representations for seamless data manipulation.
- : Processes these patch-level representations.
- Reconstructs patch representations into corresponding byte sequences.
Key Benefits of the BLT
- The BLT architecture significantly minimizes computational costs during training and inference by adaptively adjusting patch sizes, thereby yielding up to a 50% reduction in floating-point operations (FLOPs) compared to traditional models like LLaMA-3.
- By operating at the byte level from the outset, the BLT showcases its robustness in the face of entropy, ensuring seamless performance across a broad range of tasks.
- The byte-level approach allows for the meticulous capture of linguistic subtleties, rendering it particularly valuable for tasks demanding a profound comprehension of phonology and orthography.
- The scalable architecture is engineered to thrive, effortlessly handling increasing fashion and dataset sizes without sacrificing performance.
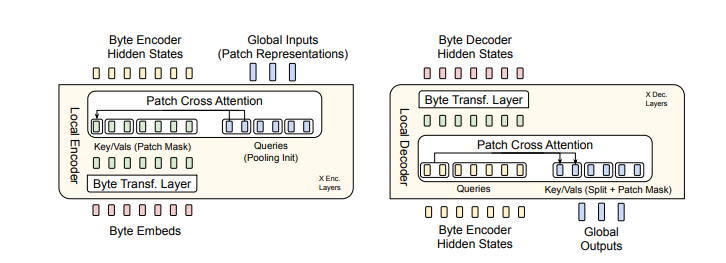
Experimental Outcomes
Significant experiments confirm that the BLT outperforms traditional tokenization methods in terms of efficiency, utilizing fewer resources. As an illustration:
- According to the HellaSwag noisy benchmark results, Llama 3 demonstrated an accuracy of 56.9%, while the BLT model achieved a significantly higher accuracy rate of 64.3%.
- In character-level understanding tasks such as spelling and semantic similarity benchmarks, the system demonstrated near-perfect accuracy rates.
These outcomes highlight the BLT’s potential as a compelling alternative for various purposes.
Actual-World Implications
The introduction of the BLT sandwich presents a tantalizing array of possibilities for culinary innovation.
- Enhancing eco-friendly coaching and inferencing capabilities.
- Effective processing of linguistically complex tongues.
- Achieving optimal performance even in the presence of noisy or varying input data.
- Enhancing Equity in Multimodal Language Understanding.
Limitations and Future Work
Researchers recognise that despite its pioneering significance, there are numerous avenues for further investigation.
- Emergence of comprehensive patchwork trends.
- Optimizing Byte-Level Processing Strategies for Enhanced Efficiency and Scalability.
- Scaling legal guidelines for byte-level transformers: A comprehensive investigation.
Conclusion
The Byte-Literal Transformer signifies a significant breakthrough in natural language processing, rendering traditional segmentation methods obsolete. Its progressive structure not only boosts effectivity and robustness but also revolutionizes the way we comprehend and create human language. As researchers continue to uncover its potential, we expect exciting breakthroughs that will lead to the creation of even more innovative and agile methods. The BLT concept has the potential to revolutionize natural language processing, fundamentally transforming AI’s capacity for comprehending and generating human language with unprecedented accuracy.
The submission appeared initially on.