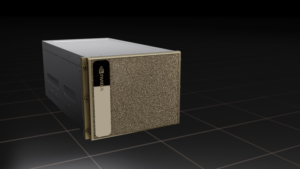
Nvidia’s latest DGX series: a powerhouse for AI and data science.
Clients enjoy a wide range of options when building their generative AI architectures, allowing them to train, refine, and deploy AI models with flexibility and precision. At times, an abundance of options can indeed be overwhelming. Nvidia has introduced DGX Cloud, a cloud-based solution designed to streamline decision-making and accelerate the training of AI models by providing access to powerful computing resources through Amazon Web Services (AWS).
The NVIDIA DGX methods are widely regarded as the industry standard for GenAI workloads, encompassing training massive language models, fine-tuning them, and running inference workloads at scale. The DGX methods leverage the latest generation of NVIDIA GPUs, including the H100 and H200 series, as well as the company’s comprehensive AI software stack, featuring Nvidia Inference Microservices (NIMs), Riva, NeMo, and RAPIDS frameworks, alongside other tools.
Nvidia is empowering users with access to its DGX Cloud, offering a range of GenAI development and manufacturing capabilities, including DGX methods, but now delivered via the cloud. NVIDIA previously offered DGX Cloud on Microsoft Azure, Google Cloud, and Oracle Cloud Infrastructure; last week, it unveiled the availability of DGX Cloud on Amazon Web Services (AWS) at re:Invent 2022.
“Nvidia’s VP of DGX Cloud, Alexis Bjorlin, emphasizes the value proposition of their managed service: ‘When you consider DGX Cloud, we’re offering a premium solution that delivers unparalleled performance’.” “It’s a decidedly opinionated approach to optimizing AI efficiency and streamlining pipelines.”
Constructing a comprehensive GenAI system requires far more than merely procuring high-performance computing infrastructure, such as Nvidia GPUs, or readily available AI models like LLaMA-3, followed by a cursory injection of knowledge. There are occasionally additional steps, such as knowledge curation, meticulous fine-tuning of the model, and artificial intelligence technologies, that a customer should integrate into an end-to-end AI workflow, securing it with guardrails, Bjorlin noted. I’d say 95% accuracy. Is downsizing the fashion industry a viable solution to sustainability concerns?
()
NVIDIA boasts extensive experience in building AI pipelines across various infrastructure types, leveraging this expertise to offer its DGX Cloud service, which enables customers to tap into its knowledge base. By simplifying the process, customers are spared from navigating excessive intricacies, allowing for expedited GenAI development and deployment, according to Bjorlin.
At the recent re:Invent conference, Bjorlin underscored the significance of achieving rapid deployment capabilities, emphasizing that getting up and running with time-to-first-train is a critical performance indicator. How long would it typically take for someone to create a personalized, custom-made model from scratch, allowing them to dictate its features and uses? One key metric that enables us to hold ourselves accountable is our developer velocity.
Despite the initial accomplishment of establishing a seamless operations model, the journey doesn’t stop there. Nvidia’s DGX Cloud enables expert assistance in the nuances of model development, including optimizing training routines, as noted by Bjorlin.
Typically, our interactions involve guiding environmentally conscious individuals who require tailored advice to optimize their sustainable practices. In order for them to operate efficiently, calculations often require a reduction in precision from 16-bit formats like FP16 or BF16 to the more economical 8-bit floating-point format, FP8. It’s possible that the quantization of the information might be a factor. What are the key considerations when adopting a distributed strategy to scale a model’s processing power across an infrastructure, leveraging four types of parallelism: data parallelism, model parallelism, knowledge parallelism, and pipe-line parallelism?
We examine the mannequin and work with the architect to ensure seamless integration onto existing infrastructure. That’s a complex concept, indeed, given the intricacies involved in synchronizing computational, communication, and memory timelines. To maximize your effectiveness in your endeavors? That’s why we’re delivering measurable results through outcome-based capabilities.
Nvidia’s DGX Cloud now supports H100 graphics processing units (GPUs) on Amazon Web Services’ (AWS) Elastic Compute Cloud (EC2) P5 instances, with future support for the newly launched EC2 P6 instances. This cutting-edge technology empowers businesses of every scale to harness the power of massive language models, enabling them to mentor, refine, and execute complex AI processes alongside industry-leading giants.
AWS offers numerous types of customers leveraging DGX Cloud capabilities. The technology has gained widespread adoption among both large companies, which leverage it to train fundamental fashion styles, as well as smaller firms that refine pre-trained models with their proprietary data, according to Bjorlin. Nvidia seeks to maintain flexibility to cater to a wide range of requirements.
“Increasingly, people are turning to cloud computing as a convenient and accessible way to access computer resources.” “And as experts in recognising the importance of continuous improvement, we must excel in refining our silicon, methodologies, intellectual property, design expertise, and software ecosystem.”
With DGX Cloud, clients can leverage significant advantages, including accelerated time-to-first practice, by gaining access to a DGX system with a commitment of just one month. For AI startups like those participating in Nvidia’s Inception program, having a cloud-based platform is particularly useful as they continue to test and refine their AI concepts before considering mass production.
NVIDIA boasts a significant partnership network of approximately 9,000 Inception collaborators, with the availability of its DGX Cloud on Amazon Web Services (AWS) poised to amplify their success, according to Bjorlin. “This facility serves as a proving ground,” she declared. Builders within the organization say: “We’re evaluating multiple instances of Amazon’s DGX cloud infrastructure on AWS.”
“Nvidia is a company that has always prioritized close relationships with developers,” “Across the globe, builders are leveraging Nvidia’s technologies. As such, it’s a seamless approach for us to integrate their expertise and have them develop an AI-powered solution, which can then be deployed on Amazon Web Services.”