Massive language models (LLMs) exhibit exceptional capabilities in generating subsequent phrases or phrases with ease through their advanced autocompletion features. Recent advancements have empowered large language models (LLMs) to intuitively comprehend and adhere to human instructions, execute sophisticated tasks, and engage in meaningful dialogues. Fine-tuned large language models (LLMs) with specialized datasets and reinforced by human feedback (RLHF) drive these advancements. RLHF revolutionizes machine learning by enabling seamless collaboration between artificial intelligence systems and human operators.
What’s RLHF?
Reinforcing Large-scale Human Feedback (RLHF) enables the training of a massive language model to harmonize its outputs with human inclinations and anticipations by leveraging human guidance. The mannequin’s performance is evaluated based on people’s feedback and ranking systems, allowing it to continually refine its outputs. This iterative process enables large language models (LLMs) to refine their comprehension of human instructions and produce more accurate and relevant responses. RLHF has played a crucial role in optimizing the performance of models like Sparrow, Claude, and others, thereby allowing them to surpass the capabilities of traditional large language models (LLMs), such as GPT-3.
Let’s perceive how RLHF works.
RLHF vs Non-RLHF
Significant advancements in natural language processing have led to the development of massive language models, which utilize input known as “prompt” to predict the next word or token that completes a sentence. To finalize a press release, I would suggest leveraging AI-driven tools like GPT-3 to streamline the process and optimize content for maximum impact.
Immediate: Human intuition allows techniques to navigate advanced and nuanced eventualities that may potentially battle with. For instance, in taxation, skilled professionals can provide personalized guidance to individuals and businesses, helping them navigate complex financial regulations and ensure compliance with tax laws.
The mannequin swiftly substantiates its claim by stating:
“Humans’ entry into complex systems enables the development of techniques capable of navigating advanced and nuanced eventualities that may arise.” In the realm of taxation, expert human consultants excel at deciphering complex tax regulations, crafting bespoke guidance for individual clients’ needs, and applying nuanced thoughtfulness to navigate ambiguous legislation.
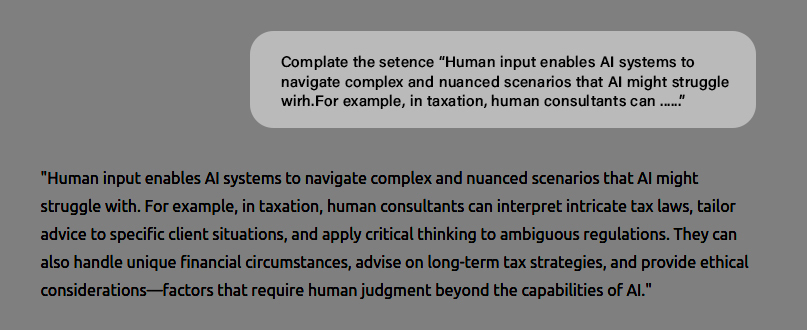
Please provide the text you would like me to improve.
Despite this, massive linguistic trends are poised to exceed expectations. Large Language Models are expected to write stories, compose emails, craft poems, generate code, and accomplish various other tasks.
RLHF vs Non-RLHF Examples
The key differences between non-RLHF and RLHF outputs are illustrated in the following examples, showcasing distinct features of subsequent token predictors and models educated on human feedback.
Non-RLHF Output – Story
As the sun set over the war-torn land, Princess Anastasia’s thoughts drifted to the dashing young soldier she had met earlier that day. His piercing blue eyes and chiseled jawline still lingered in her mind, making her heart flutter with an unfamiliar sensation.
Immediate: As the sun set over the rolling hills of Russia, Princess Anastasia gazed out at the soldiers gathered before her palace. She had called them together to discuss the growing threat from the neighboring kingdom, but her mind wandered as she watched them prepare for battle.
Among them was a young soldier named Sergei, his piercing blue eyes and chiseled features drawing her attention like a magnet. Anastasia felt a flutter in her chest as their gazes met, and she quickly looked away to hide her blush.
Over the next few weeks, Anastasia found herself thinking of Sergei more and more. She would sneak glances at him during council meetings, her heart racing with excitement whenever he caught her eye.
Despite this, enormous linguistic trends are expected to go far beyond merely filling an instant. Large language models are expected to note down stories, emails, poetry, coding scripts, and other forms of writing.
The following demonstrates the divergence between non-RLHF (Subsequent token predictor) and RLHF (instructed by human feedback) output:
As the sun set over the war-torn landscape, Princess Anastasia gazed out at the rows of tents and makeshift shelters. Her heart heavy with sorrow, she thought back on the countless nights spent sleeping under starry skies, her only solace the whispered rumors of a dashing soldier rumored to be stationed nearby.
Immediate: As the sun set over the rolling hills of Russia, Princess Anastasia stood at the edge of the palace garden, her mind wandering to the handsome young soldier who had caught her eye.
SKIP
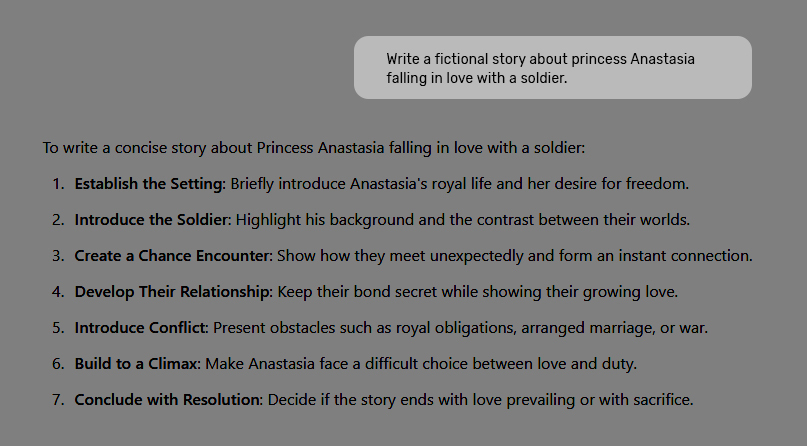
The mannequin is capable of learning how to write stories, but it may not comprehend requests because LLMs are trained on web scraps that are less familiar with instructions like ‘write a story/email,’ followed by the story or email itself? Predicting subsequent phrases is fundamentally distinct from astutely adhering to instructions.
Here’s what you get when identical input is supplied to a highly advanced AI system:
Immediate: As the sun set over the grand ballroom, Princess Anastasia’s eyes met those of Captain Dmitri Petrov. His piercing blue gaze held hers captive, and for a moment, she forgot about her royal duties and the weight of her family’s legacy.
SKIP
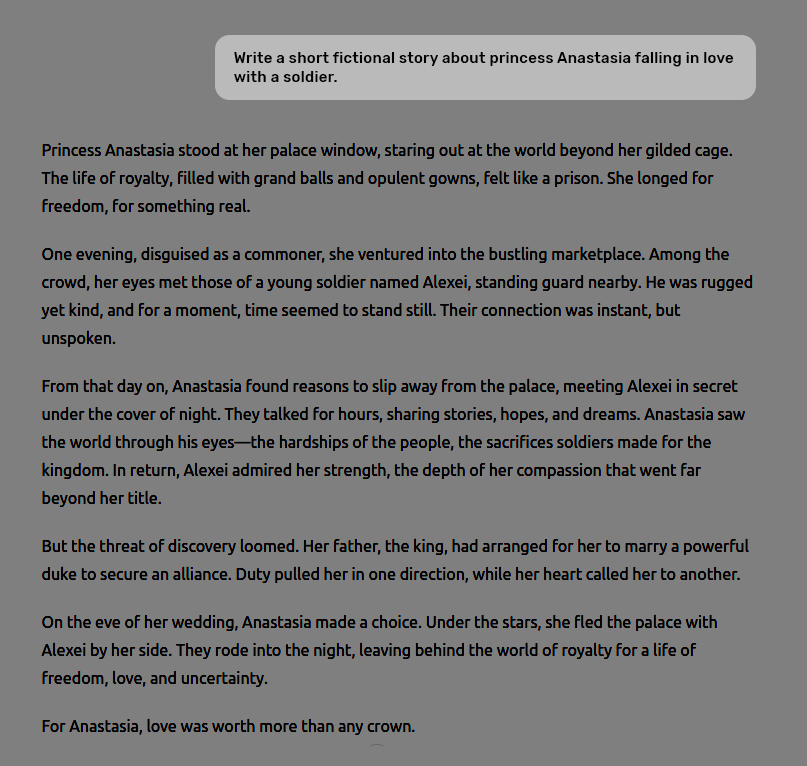
Now, the generated the specified reply.
Immediate: What’s 4-2 and 3-1?
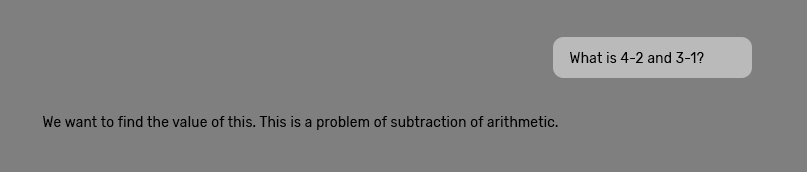
The non-RLHF mannequin does not respond to queries and instead incorporates them into a narrative dialogue seamlessly.
Immediate: What’s 4-2 and 3-1?
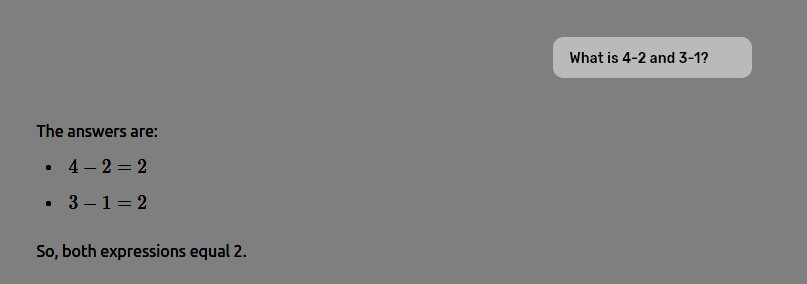
The Reinforcement Learning-based Human-like Fellow (RLHF) mannequin effectively comprehends contextual demands and formulates a suitable response promptly.
Let’s examine how a large language model is trained on human input to respond appropriately.
The RLHF methodology commences by leveraging a pre-trained language model or next-token predictor as its foundation.
The following tasks require completion by the mannequin: In different phrases, a coaching dataset consisting of <immediate, corresponding ideally suited output> pairs is created to fine-tune the pre-trained mannequin to generate comparable high-quality responses.
This step involves creating a benchmark model to assess the extent to which the output meets elevated standards of excellence. Like a trained model, a reward mannequin is initially taught on a comprehensive dataset of human-rated responses, serving as the benchmark for evaluating the quality of generated replies. With unnecessary layers pruned to optimize performance over production, it becomes a leaner version of the original. The reward model processes the input and generated response, assigning a numerical rating – a scalar reward – to the output.
Human evaluators assess the generated content primarily based on its relevance, accuracy, and readability, considering it to be of high quality if it meets these criteria.
The culmination of the RLHF process lies in training a reinforcement learning policy, specifically a coverage model that determines which phrase or token to generate next within a text sequence, effectively learning to produce text that aligns with the reward model’s predictions of what people would find appealing.
Through diverse phrasing, the RL algorithm develops the capacity to reason like a human by optimally leveraging insights gleaned from a reward model.
That’s how a cutting-edge AI model, such as the one powering this conversational powerhouse, is designed and refined through meticulous training and tuning.
Significant advancements in language technologies have been made in recent years, continuing to shape the future. Strategies such as Reinforced Learning-based Human Feedback (RLHF) have led to the development of AI models like ChatGenesis (ChaGPT) and Gemini, which have revolutionized response quality across various tasks and responsibilities. By leveraging human input during the fine-tuning process, large language models (LLMs) not only excel at following instructions but also demonstrate increased alignment with human values and preferences, ultimately enhancing their ability to understand the scope and intended use cases for which they are designed?
Researchers are revamping enormous language models (ELMs) by refining their predictive capabilities and adaptability to follow human instructions accurately. Unlike traditional language models, which were primarily designed to predict forthcoming phrases or tokens, RLHF-trained models utilize human guidance to refine responses, harmonizing them with user preferences.
Researchers are revamping enormous language models by refining their capacity for accurate responses and increasing their ability to adapt to human prompts. Unlike traditional large language models, which were originally developed to predict the next phrase or token, RLHF-trained models leverage human feedback to refine their responses, ensuring that they align with individual users’ preferences.
The article was published for the first time on.