Up to now, various styles have fulfilled specific purposes in artificial intelligence. These developments have significantly influenced human life, revolutionizing the way we comprehend and generate textual content, particularly with the advent of natural language processing capabilities. Notwithstanding the benchmarks established by these fashion trends, they fall short of incorporating real-world movements and interactions. Does the concept of an autonomous system rely on its ability to make decisions independently, driven by the data and information it has acquired? When that is the case, AI brokers enter the picture? Brokerages employ autonomous techniques that serve a dual purpose, functioning efficiently without human oversight.
By combining with cutting-edge linguistic innovations, AI intermediaries can open up a vast expanse of sagacious decision-making and swift execution possibilities. Historically, fashion trends such as Large Language Models (LLMs) have strived to overcome memory and contextual constraints by increasing input capacity or integrating external data retrieval techniques with technological advancements. While existing methods enhance the dummy’s ability to process large datasets or intricate guidelines, they still rely heavily on stable settings. RAG demonstrates exceptional prowess in enriching the mannequin’s comprehension via seamless integration with external knowledge bases; simultaneously, Long-Context Language Models excel at navigating complex interactions or documentation by masterfully preserving relevant contextual threads. Despite lacking the capacity for self-directed, goal-oriented behavior. The location where Agentic Rescue Group intervenes in a crisis situation. The evolution of Agentic RAG will be a topic for additional discussion in this article.
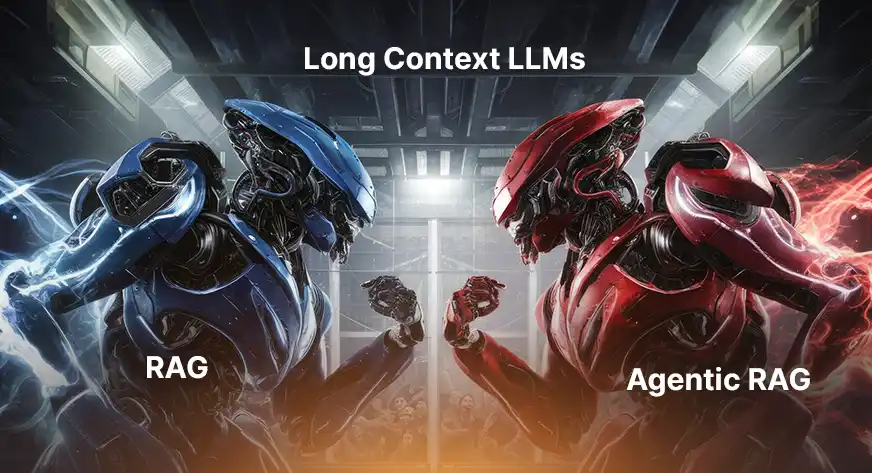
Overview
- Building upon conventional large language models (LLMs), we have successfully transitioned to Reinforced Adversarial Generation (RAG) and Agentive RAG, thereby significantly augmenting our capacities.
- Conventional large language models excel at processing textual information but struggle to undertake independent actions autonomously.
- RAG significantly enhances language learning models (LLMs) by seamlessly incorporating external knowledge to deliver more accurate responses.
- Empowers autonomous decision-making capabilities, facilitating adaptive and flexible task execution.
- Unifies Relevance-Aware Generation (RAG) with Lengthy Context Language Models to achieve a harmonious blend of utility and performance.
- Choices rely on various factors, including cost efficiency, contextual relevance, and the complexity of questions being asked.
The Evolution of Agentic Real-World Gamification: A Snapshot
With the advent of Large Language Models (LLMs), a seismic shift occurred in the way people interacted with data. Despite their reputation for resolving complex problems, relying on them often resulted in factual errors, since they primarily drew upon internal databases.
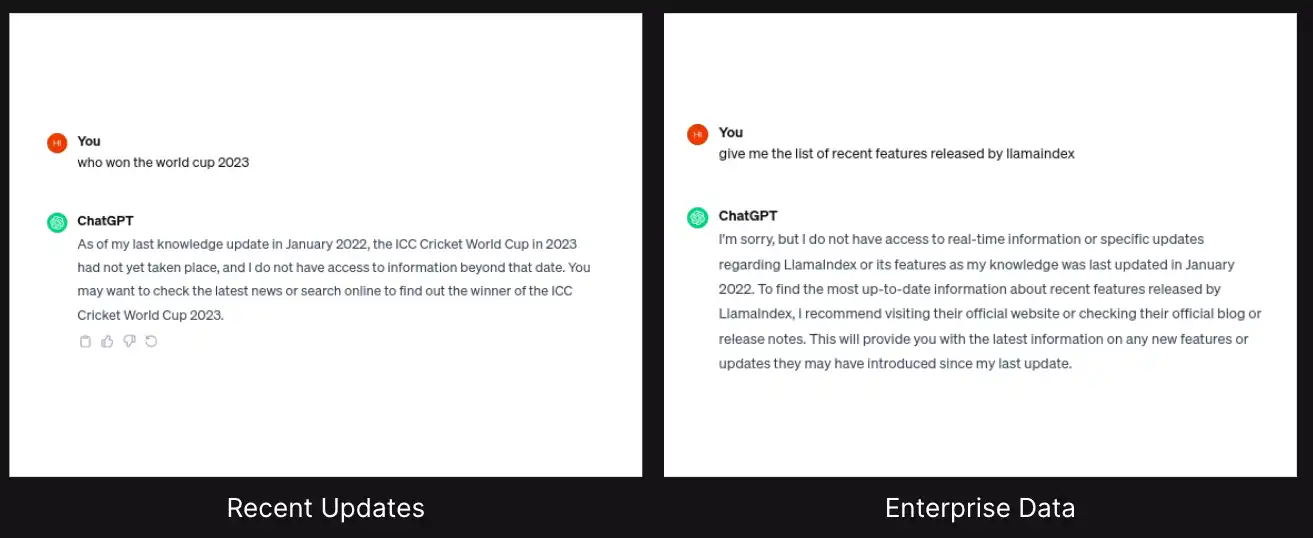
RAG stands for Reinforcement-Augmented Generation, which represents a methodology or strategy to inject external information into Large Language Models (LLMs), thereby enhancing their capabilities.
We will seamlessly integrate with external databases, such as those used by language models like ChatGPT, and immediately prompt these models to retrieve relevant solutions from the connected data sources.
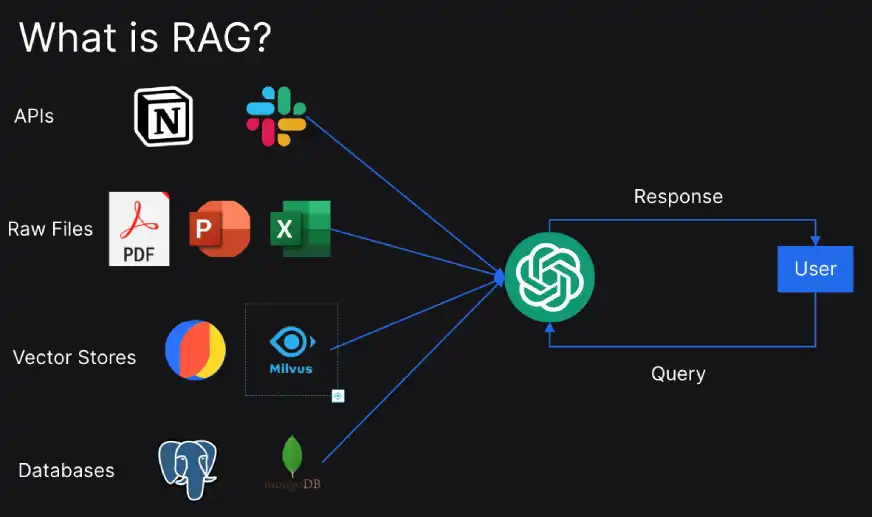
Here’s a rapid perception of how RAG works: RAG stands for Risk Appetite and Governance. It is a framework designed to manage risks effectively in organizations by defining their risk tolerance level, or risk appetite, and establishing governance structures to ensure compliance with that appetite.
- During the preliminary phase, questions are thoroughly analyzed to optimize search performance.
- Within the final stage, the front-end large language model leverages data sourced from an external database to generate precise and accurate responses.
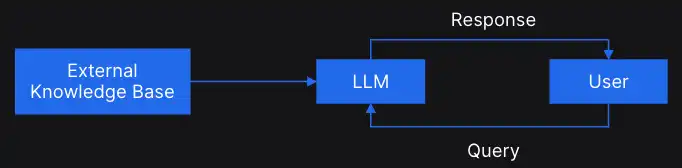
Despite excelling in processing straightforward requests across multiple documents, RAG still falls short of demonstrating sophisticated cognitive abilities. The development of agentic RAG enabled the creation of a self-sufficient decision-making framework, capable of processing initial data and selecting the most effective tools to optimize subsequent responses in a strategic manner.
While and are indeed intently associated with phrases that fall beneath the broader umbrella of Agentic Techniques. Before delving into Agentic RAG in-depth, let’s first review recent breakthroughs in Large Language Models (LLMs) and Reinforcement Agent Generation (RAG).
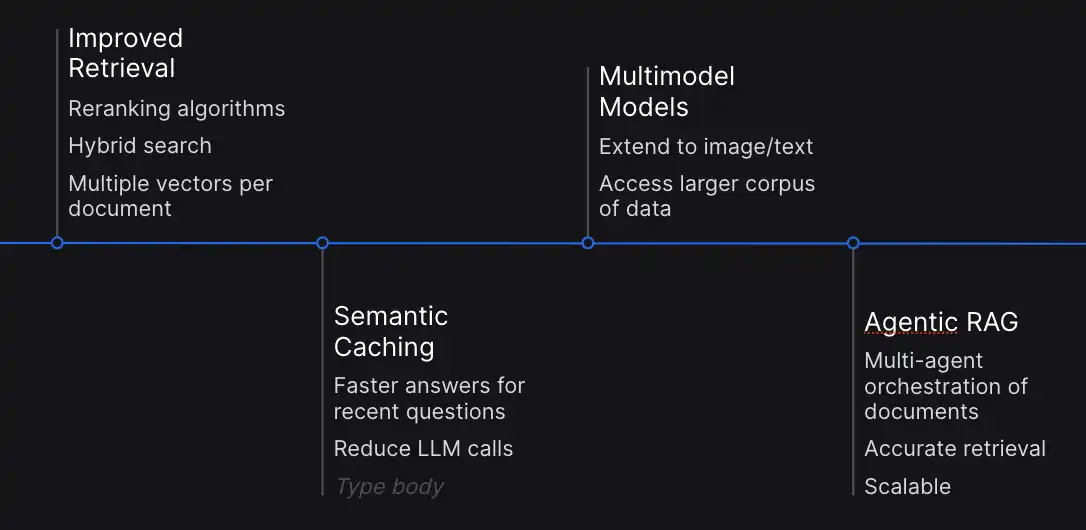
- To achieve consistent performance, you must fine-tune data retrieval for optimal results. Developments are currently focused on refining reranking algorithms and hybrid search approaches, further enhancing relevance recognition through the application of multiple vectors per document.
- Semantic caching has become a crucial strategy for reducing computational complexity issues. It allows for storing solutions to the latest queries, enabling efficient reuse of previously generated responses to identical inquiries without unnecessary duplication.
- This enhancement enables large language models (LLMs) and multimodal architectures to go beyond text-based limitations, seamlessly incorporating photographs and various modalities. This integration enables a harmonious fusion of textual and visual data, streamlining the exchange of information across different mediums.
Traditional brokers often rely on human analysis to provide clients with tailored investment advice. In contrast, artificial intelligence (AI) brokers utilize machine learning algorithms to analyze vast amounts of data, enabling them to deliver highly personalized recommendations. Key variations include the reliance on human expertise versus computational capabilities, as well as the potential for AI-driven systems to process information more quickly and accurately than their human counterparts.
RAG brokers typically rely on their own research and analysis when creating investment strategies for clients, whereas AI brokers leverage vast datasets to identify trends and patterns that may not be immediately apparent. This fundamental difference can significantly impact the quality of advice provided.
Up until now, we’ve grasped the fundamental distinctions between RAG and AI brokers; nonetheless, to truly comprehend these differences intimately, let’s delve deeper into some key defining characteristics.
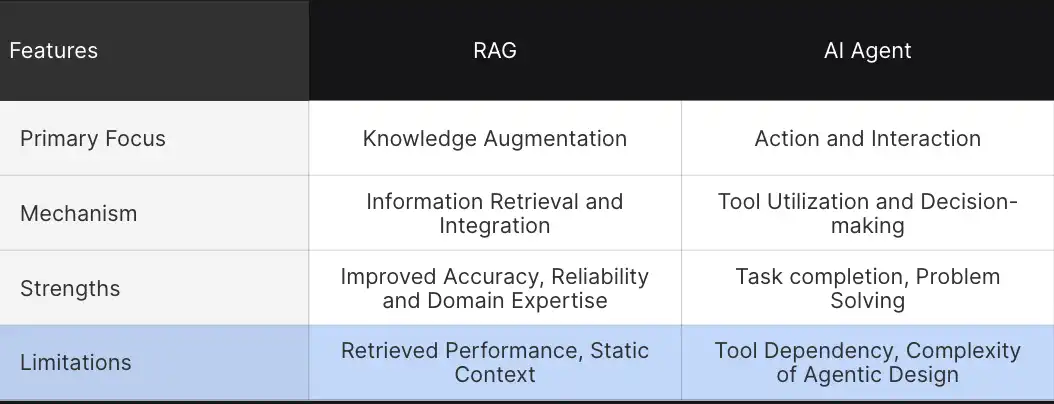
- The primary function of this mechanism is to augment the knowledge base, thereby refining the mannequin’s comprehension through the retrieval and integration of relevant information. This allows for additional decision-making and enhanced contextual comprehension. Unlike other AI models, AI brokers are specifically engineered to facilitate decision-making and seamless interactions with their surroundings. Brokers take a significant leap forward, collaborating seamlessly with various instruments to execute complex tasks.
- Data-driven insights are the foundation of RAG’s operations, fueled by seamless data extraction and integration processes. The technology incorporates data from external sources, seamlessly integrating it into its answers, whereas AI intermediaries function through the execution of instruments and self-directed decision-making processes.
- RAG’s unique strength lies in its ability to generate more informed and thoughtful answers. By integrating Large Language Models (LLMs) with external knowledge, Relevance-Augmented Generation (RAG) enables the provision of more accurate and contextually relevant information. Brokers excel in autonomous trading executions, seamlessly interacting with market conditions.
- RAG techniques encounter difficulties such as retrieval issues, stagnant contextual limitations, and a lack of autonomous intervention when generating responses. While brokers excel in many areas, their primary constraints arise from an overreliance on tools and the intricacy of agent-based system architectures.
Can lengthy context-dependent language models (LLMs), response-adversarial generators (RAGs), and agentive RAGs be distinguished by their architectural configurations?
Traditional LLMs rely on attention mechanisms to process input sequences, leveraging contextualized representations for generation tasks. In contrast, RAGs employ reinforcement learning frameworks to optimize sequence prediction, often incorporating adversarial training schemes.
To date, it has become evident that combining Large Language Models with retrieval mechanisms has yielded significantly enhanced AI capabilities, while Agentic Retrieval-Augmented Generator (ARAG) is innovatively optimizing the synergy between the retrieval system and technological model.
With a solid foundation in place, let’s delve into the diverse architectural manifestations that arise from the interplay of these innovative technologies.
Function | Lengthy Context LLMs | RAG ( Retrieval Augmented Era) | Agentic RAG |
Core Elements | Static data base | LLM+ Exterior information supply | Large-scale Language Models in conjunction with efficient Retrieval modules and integrated Autonomous Agents? |
Info Retrieval | No exterior retrieval | Queries exterior information sources throughout responses | The queries exterior databases seamlessly, selecting suitable instruments with precision. |
Interplay Functionality | Restricted to textual content technology | Retrieves and integrates context | Autonomous selections to take actions |
Use Instances | Textual content summarization, understanding | Augmented responses and contextual technology | Multi-tasking, end-to-end activity technology |
Architectural Variations
- Transformer-based models bearing similarities to GPT-3 often excel on large datasets and rely heavily on a fixed knowledge base. Their structural integrity is well-suited for processing textual content and summarization tasks that do not necessitate external data to produce responses. Despite their limitations, existing resources often fall short in providing access to current or specialized information. Our primary area of concentration is the Long-Form Contextual Large Language Models. These fashion designs are engineered to accommodate and process far longer input sequences than traditional large language models (LLMs).
Fashions echoing those of GPT-3 and its predecessors often impose limitations on the range of input tokens that can be accommodated. Lengthy context models address these constraints by expanding the context window’s spatial dimensions, rendering them more comprehensive at:- Summarizing bigger paperwork
- Sustaining coherence over lengthy dialogues
- Processing paperwork with intensive context
- Rethinking AI’s architecture, RAG has emerged as a promising solution to overcome the limitations of Large Language Models (LLMs). The retrieval component enables Large Language Models (LLMs) to access external information resources, while the augmentation aspect allows Retrieval-Augmented Generative models (RAGs) to provide additional context-specific information beyond that of a standard LLM. Despite its advanced features, RAG remains incapable of making independent decisions autonomously.
- Subsequent to this innovation is an Agentic Risk and Governance (RAG) framework, which incorporates an additional layer of intelligent decision-making capabilities. The system may effectively procure external information and houses a self-governing reasoning module that scrutinizes the gathered data and executes tactical decisions.
These architectural distinctions help clarify how each system enables data, augmentation, and integration to function seamlessly together.
Can Longitudinal Memory-augmented Generative (LLMs), Reference-based Adversarial Generation (RAG), and Agentive RAG effectively leverage context?
- There have long been efforts to equip large language models (LLMs) with the ability to effectively process lengthy contexts. While recent large language models such as Gemini 1.5, GPT-4, and Claude 3 have significantly increased their contextual understanding capacities, the perceived value of long-context prompts has remained stagnant.
- Augmented large language models (LLMs) using Response-based Adaptive Generation (RAG) yielded suboptimal efficiency when compared to Language Chains (LC). Notwithstanding its significantly decreased computational value, this makes it a viable option. The graph indicates a substantial fee disparity of approximately 83% between LLMs and RAG for the reference fashions. Therefore, RAGs remain timeless and unaffected by passage of time. There is a need for a method that leverages the synergy between these two approaches to create a fast and affordable prototype simultaneously.
Before delving into the novel fusion methodology, it’s essential to examine the outcome that has emerged from its application thus far.
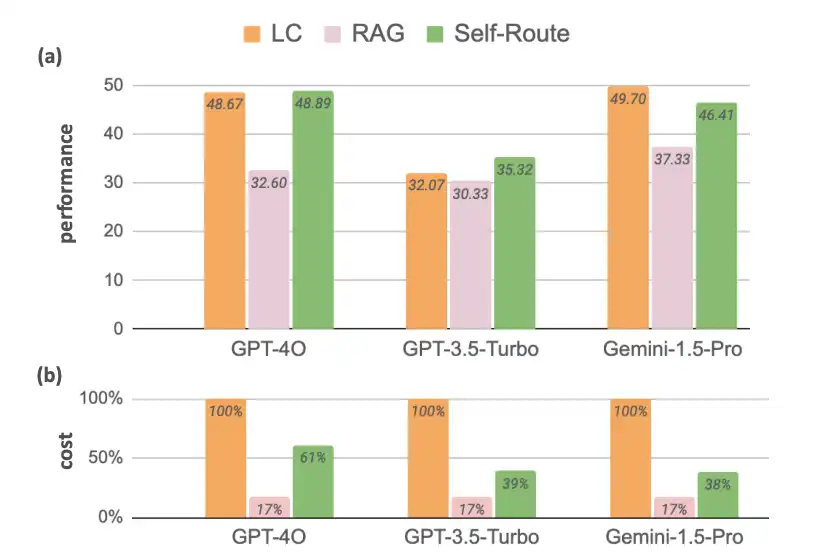
Self-Route is a novel Agent-based Retrieval-Augmented framework, engineered to strike a harmonious balance between economic value and operational efficacy. When dealing with complex queries that don’t require routing, the approach uses fewer tokens and instead leverages local connectivity (LC) for more intricate inquiries.
Let’s grasp self-route now.
What if we merged two prominent models – Recurrent Attentional Graph (RAG) and Agent-based RAG (Agented RAG)? This novel approach would enable neural networks to not only attend to relevant information within a graph but also simulate the strategic actions of multiple agents operating on that graph. By fusing these two paradigms, we could develop more sophisticated models for complex decision-making tasks.
The Self-Route design sample leverages Large Language Models (LLMs) to route queries through self-reflection, predicated on the assumption that LLMs are well-calibrated in predicting whether a question is answerable based on provided context.
- Retrieved chunks are fed into the Large Language Model (LLM), prompting it to predict the likelihood of the query being resolvable, and if so, fabricating a response. What is your task?
- When confronted with seemingly intractable queries, a necessary next step is to provide comprehensive contextual information to large language models (LLMs) to elicit their most informed predictions.
While Self-Route may prove efficient in balancing efficiency and value, it is essential to note that this approach is particularly effective when seeking a harmonious balance between the two considerations. This ensures seamless integration into complex systems requiring handling diverse query sets.
Key Takeaways
- ?
- There is a desire to reduce computational costs.
- Questionnaires often exceed the mannequin’s contextual boundaries, thereby rendering the results of the Ragin’ Most Effective survey inconsequential.
- ?
- Processing complex information efficiently is essential.
- Substantial resources are readily available to support complex calculations of greater magnitude.
-
- A well-rounded response is essential – certain inquiries will be addressed using the Red-Amber-Green (RAG) system, while more complex ones will be handled by our specialized team, LC.
Conclusion
We’ve discussed the progression of Agentic RAG, with a focus on assessing Long-Tailed Contextual Language Models, followed by the emergence of Retrieval-Augmented Generative models and ultimately, the pinnacle achieved in extra-ordinary Agentic RAG. While Long-form Language Models (LLMs) shine in maintaining context across extensive conversations or voluminous documentation, RAG innovates by seamlessly incorporating external information to enhance contextual precision. Notwithstanding each organization’s tendency to fall short through lack of self-initiated action.
As agent-centric RAG evolves, we’ve introduced a revolutionary intelligence layer that empowers decision-making and autonomous actions, seamlessly integrating static data analysis with dynamic process execution. Here is the rewritten text:
The article introduces “Self-Route,” a novel approach that harmoniously blends the advantages of Recurrent Attention Graph (RAG) and Long-Term Memory Language Models (LLMs), striking a balance between efficiency and performance by prioritizing query routing based on complexity.
Ultimately, the choice among these approaches hinges on specific requirements, including cost-effectiveness, contextual nuances, and query complexity, with Self-Route emerging as a versatile solution for various applications?
Regularly Requested Questions
Ans. RAG represents a paradigm where a large language model (LLM) is integrated with an external database to facilitate seamless information retrieval and processing. The ability of an LLM to provide accurate answers is significantly bolstered by its capacity to gather and assimilate relevant external information, effectively incorporating it into its responses.
Ans. Contextual language models capable of handling significantly longer input tokens than traditional models enable them to manage coherence across extensive text and effectively summarize larger documents.
Ans. AI brokers are autonomous technologies that can autonomously make decisions and take actions solely based on analyzed data. Unlike traditional systems that require human intervention, AI Brokers collaborate seamlessly with their environment to complete tasks autonomously.
Ans. Professional language models excel in processing dense content, such as condensing extensive reports or maintaining continuity throughout prolonged discussions, where sufficient resources are available to absorb higher computational costs.
Ans. The Requisite Attention Graph (RAG) outperforms Lengthy Context Large Memory Models in terms of cost-efficiency, making it an ideal choice for situations where computational value is paramount and supplementary contextual information is necessary to accurately respond to queries.