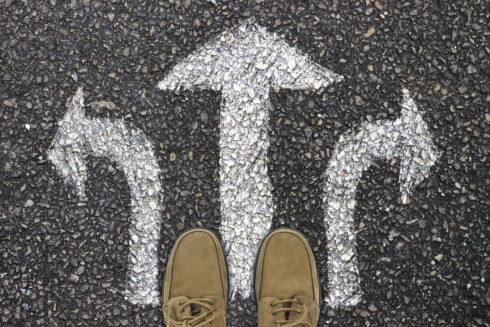
Immediately’s enterprise panorama is arguably extra aggressive and complicated than ever earlier than: Buyer expectations are at an all-time excessive and companies are tasked with assembly (or exceeding) these wants, whereas concurrently creating new merchandise and experiences that can present customers with much more worth. On the identical time, many organizations are strapped for sources, contending with budgetary constraints, and coping with ever-present enterprise challenges like provide chain latency.
Companies and their success are outlined by the sum of the choices they make each day. These selections (unhealthy or good) have a cumulative impact and are sometimes extra associated than they appear to be or are handled. To maintain up on this demanding and continuously evolving setting, companies want the power to make selections rapidly, and plenty of have turned to AI-powered options to take action. This agility is vital for sustaining operational effectivity, allocating sources, managing danger, and supporting ongoing innovation. Concurrently, the elevated adoption of AI has exaggerated the challenges of human decision-making.
Issues come up when organizations make selections (leveraging AI or in any other case) with out a stable understanding of the context and the way they are going to affect different elements of the enterprise. Whereas pace is a crucial issue in relation to decision-making, having context is paramount, albeit simpler stated than accomplished. This begs the query: How can companies make each quick and knowledgeable selections?
All of it begins with knowledge. Companies are aware of the important thing function knowledge performs of their success, but many nonetheless battle to translate it into enterprise worth by efficient decision-making. That is largely resulting from the truth that good decision-making requires context, and sadly, knowledge doesn’t carry with it understanding and full context. Due to this fact, making selections based mostly purely on shared knowledge (sans context) is imprecise and inaccurate.
Beneath, we’ll discover what’s inhibiting organizations from realizing worth on this space, and the way they will get on the trail to creating higher, quicker enterprise selections.
Getting the total image
Former Siemens CEO Heinrich von Pierer famously stated, “If Siemens solely knew what Siemens is aware of, then our numbers can be higher,” underscoring the significance of a corporation’s capability to harness its collective data and know-how. Information is energy, and making good selections hinges on having a complete understanding of each a part of the enterprise, together with how totally different sides work in unison and affect each other. However with a lot knowledge obtainable from so many alternative techniques, functions, individuals and processes, gaining this understanding is a tall order.
This lack of shared data usually results in a number of undesirable conditions: Organizations make selections too slowly, leading to missed alternatives; selections are made in a silo with out contemplating the trickle-down results, resulting in poor enterprise outcomes; or selections are made in an imprecise method that isn’t repeatable.
In some situations, synthetic intelligence (AI) can additional compound these challenges when corporations indiscriminately apply the expertise to totally different use circumstances and count on it to mechanically remedy their enterprise issues. That is prone to occur when AI-powered chatbots and brokers are inbuilt isolation with out the context and visibility essential to make sound selections.
Enabling quick and knowledgeable enterprise selections within the enterprise
Whether or not an organization’s objective is to extend buyer satisfaction, enhance income, or scale back prices, there is no such thing as a single driver that can allow these outcomes. As a substitute, it’s the cumulative impact of fine decision-making that can yield optimistic enterprise outcomes.
All of it begins with leveraging an approachable, scalable platform that enables the corporate to seize its collective data in order that each people and AI techniques alike can cause over it and make higher selections. Information graphs are more and more changing into a foundational software for organizations to uncover the context inside their knowledge.
What does this appear like in motion? Think about a retailer that wishes to know what number of T-shirts it ought to order heading into summer time. A mess of extremely advanced components have to be thought-about to make the perfect resolution: price, timing, previous demand, forecasted demand, provide chain contingencies, how advertising and promoting may affect demand, bodily area limitations for brick-and-mortar shops, and extra. We will cause over all of those sides and the relationships between utilizing the shared context a data graph offers.
This shared context permits people and AI to collaborate to unravel advanced selections. Information graphs can quickly analyze all of those components, primarily turning knowledge from disparate sources into ideas and logic associated to the enterprise as an entire. And for the reason that knowledge doesn’t want to maneuver between totally different techniques to ensure that the data graph to seize this data, companies could make selections considerably quicker.
In immediately’s extremely aggressive panorama, organizations can’t afford to make ill-informed enterprise selections—and pace is the secret. Information graphs are the vital lacking ingredient for unlocking the facility of generative AI to make higher, extra knowledgeable enterprise selections.