A novel, flexible robotic concept emerges: a shape-shifting, slime-like device capable of adapting to navigate through narrow passages, designed for deployment within the human body to safely retrieve unwanted materials.
While current robotics prototypes may not venture beyond lab settings, scientists are actively striving to create adaptable and versatile soft robots that can be applied in healthcare, wearables, and industrial contexts.
However, managing a squishy robot that lacks conventional joints, limbs, or fingers, which cannot be manipulated in the classical sense, requires an innovative approach to control. Instead, it must be able to dramatically reshape its entire form at will. Researchers at MIT are working to address this query.
Researchers have created an innovative management algorithm capable of autonomously devising strategies for transforming, extending, and rearranging a reconfigurable robot to successfully complete tasks that may necessitate frequent changes in the robot’s physical structure. The team also developed a simulator to test and validate management algorithms for deformable smooth robots on a range of challenging tasks that involve complex, shape-shifting scenarios.
Their methodology successfully fulfilled all eight tasks it assessed, outperforming other algorithms in each duty. The method worked exceptionally well on tasks requiring multiple facets. As the robotic arm navigated the narrow passage, it cleverly retracted its upper section while extending two diminutive limbs to facilitate its journey through the slender pipe. Once past the obstacle, it retracted those legs and elongated its body to access the pipe’s opening.
While reconfigurable soft robots remain in their early stages of development, this concept could potentially enable the creation of general-purpose robots capable of adapting their forms to execute diverse tasks.
When people think of flexible robots, they tend to envision machines that can stretch and then return to their original shape. Our robot’s unique ability to reconfigure itself like a shape-shifting entity makes it incredibly versatile and capable of adapting to various situations. According to Boyuan Chen, an EECS graduate student and co-author of the study, it is extremely satisfying that their methodology performed so well due to the fact they were dealing with something very new.
Chen’s co-authors include lead creator Suning Huang, a visiting scholar from Tsinghua University in China who conducted this research at MIT; Huazhe Xu, an assistant professor at Tsinghua University; and senior author Vincent Sitzmann, an assistant professor of Electrical Engineering and Computer Science (EECS) at MIT, who leads the Scene Illustration Group within the Computer Science and Artificial Intelligence Laboratory. The analysis of representations at the Global Conference on Representation Studies is anticipated to be presented.
Researchers typically train robots to complete tasks by employing machine learning techniques such as reinforcement learning, where the robot learns through a trial-and-error process, receiving rewards for actions that bring it closer to its objective.
When robotic transfers involve constant and well-defined elements, such as a gripper with three fingers, this approach can prove efficient. By employing a robotic gripper, a reinforcement learning algorithm could potentially manipulate one finger slightly, exploring through trial and error whether this adjustment yields a reward. As the process continues, the object will then transfer onto the next finger, and so on.
While shape-shifting robots, controlled by magnetic fields, can adaptively compress, flex, or lengthen their overall structures.
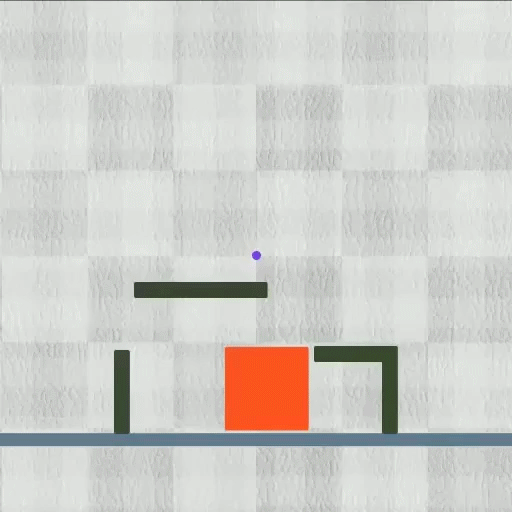
Picture: Courtesy of the researchers
According to Chen, developing a robotic with hundreds of tiny muscles requires an unconventional approach to learning, making traditional teaching methods inefficient.
To overcome this limitation, they were forced to reframe their approach entirely. Rather than training each tiny muscle separately, their reinforcement learning algorithm initiates by learning to control groups of adjacent muscles that function in unison?
After exploring the house of feasible actions, the algorithm narrows its focus to specific teams of muscles and delves deeper to refine the optimal coverage or action plan it has identified. Using a coarse-to-fine approach, the management algorithm operates.
Does coarse-to-fine imply that the initial, seemingly aimless movement increases the likelihood of creating a discernible difference? As Sitzmann notes, the transformative impact of exercising lies in its ability to coordinate the simultaneous activation of numerous muscle groups.
The researchers focus on the kinematic chain of a robot, visualizing its movement trajectory within a defined workspace, akin to a digital blueprint.
The AI-driven model leverages images of the robotic’s environment to create a 2D motion blueprint, effectively incorporating both the robot and its surroundings. Using the material-point method, they mimic robotic movements, where the motion domain is divided into discrete units akin to pixelated images, overlaid with a grid structure.
Pixels in an image are linked by their spatial proximity, just as individual pixels forming a tree in a photograph are correlated through their physical adjacency; similarly, the algorithm takes this concept into account by recognizing that nearby motion patterns exhibit stronger relationships. When the robotic arm transforms its shape, factors on its shoulder joint are transferred uniformly, whereas those on its leg joints exhibit a distinct pattern of transfer, differing from the shoulder’s.
The researchers utilize the same machine-learning model to analyze the scenario and forecast the actions the robot should execute, thereby increasing its efficiency.
To verify the efficacy of their novel approach, the researchers designed a testing framework, DittoGym, which simulated various scenarios for thorough evaluation and refinement.
The DittoGym framework outlines eight key duties that enable reconfigurable robots to seamlessly adapt and transform their shape in response to changing scenarios. In a single mission, the robot should elongate and curve its physical form to potentially navigate through obstacles and reach a target zone. One o’er, it ought to undergo transformation to mimic morphemes of the morphology.
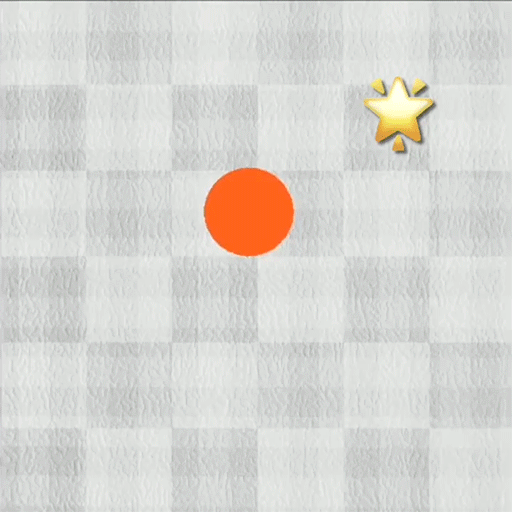
Picture: Courtesy of the researchers
Our DittoGym job selection adheres to established benchmarks in reinforcement learning, while also accommodating the unique requirements of reconfigurable robots. According to Huang, every job is characterized by certain inherent properties, such as the capacity for navigating complex exploration scenarios, adaptability in investigating environments, and the ability to interact effectively with external objects. “We envision that their combined insights will grant customers a comprehensive grasp of the adaptability offered by reconfigurable robots, as well as the efficacy of our reinforcement learning approach.”
The algorithm demonstrated superior performance relative to benchmark approaches, ultimately emerging as the go-to solution for tackling complex, multi-stage tasks that necessitated multiple iterations of form adjustments.
According to Chen, the newly established connection between motion factors exhibiting closer proximity has been instrumental in fostering exceptional results.
While shape-shifting robots may debut on a global scale within years, researchers led by Chen are poised to lay groundwork for their development, inspiring fellow scientists to explore the vast potential of 2D motion spaces in tackling complex control challenges.