It appears that there is a notable trend emerging in the development of -based applications, which shows great potential for being highly effective and valuable tools. The sample represents a synthesis of multiple approaches and scientific disciplines at their finest. It provides immense value to customers by delivering accurate results and compelling stories in a seamless, one-stop solution. The sample also leverages previous content technologies, incorporating a generous amount of interpretation and analysis. Discover the fascinating truth behind this phenomenon.
The Dawn of Generative AI: A Recent Revolution
In the initial stages of generative and large language models (LLMs), the primary emphasis was on developing solutions that could effectively respond to human inquiries. Ultimately, it became apparent that the proposed solutions were often inconsistent, to say the least, and may have even been incorrect. It’s ultimately revealed that what appears to be a bug in generative fashion models is actually a feature. The responses were probabilistically generated, regardless of the presence or absence of a definitive answer rooted in coaching principles. As confidence in this straightforward technological approach began to ebb.
As responses emerged, people focused on developing generated solutions prior to sharing them with customers; subsequently, they presented updates and reliable information about the confidence level in a solution’s appropriateness. This strategy, although initially successful in generating an answer, ultimately proves unsatisfying due to its inherent flaws and lack of guarantees for accuracy. Why don’t we extract that underlying coaching knowledge directly instead of guessing our way to a solution probabilistically? By leveraging effective strategies, current approaches are yielding substantial improvements in results.
Flipping The Script
Today’s lucrative approach revolves around identifying information initially and subsequently structuring it effectively. Methods akin to Retrieval-Augmented Generation (RAG) are helping to curtail errors while providing more robust solutions. As this search strategy has become increasingly prevalent, Google has taken a significant step by introducing a major overhaul to its search engine interface, offering users the option to opt for generative results alongside traditional search outcomes. In this image, you will observe a direct illustration of what is being described within the parentheses. This innovative strategy leverages an unconventional approach to search techniques, effectively harnessing the interpretive prowess and capabilities of Large Language Models that surpass their technological limitations.
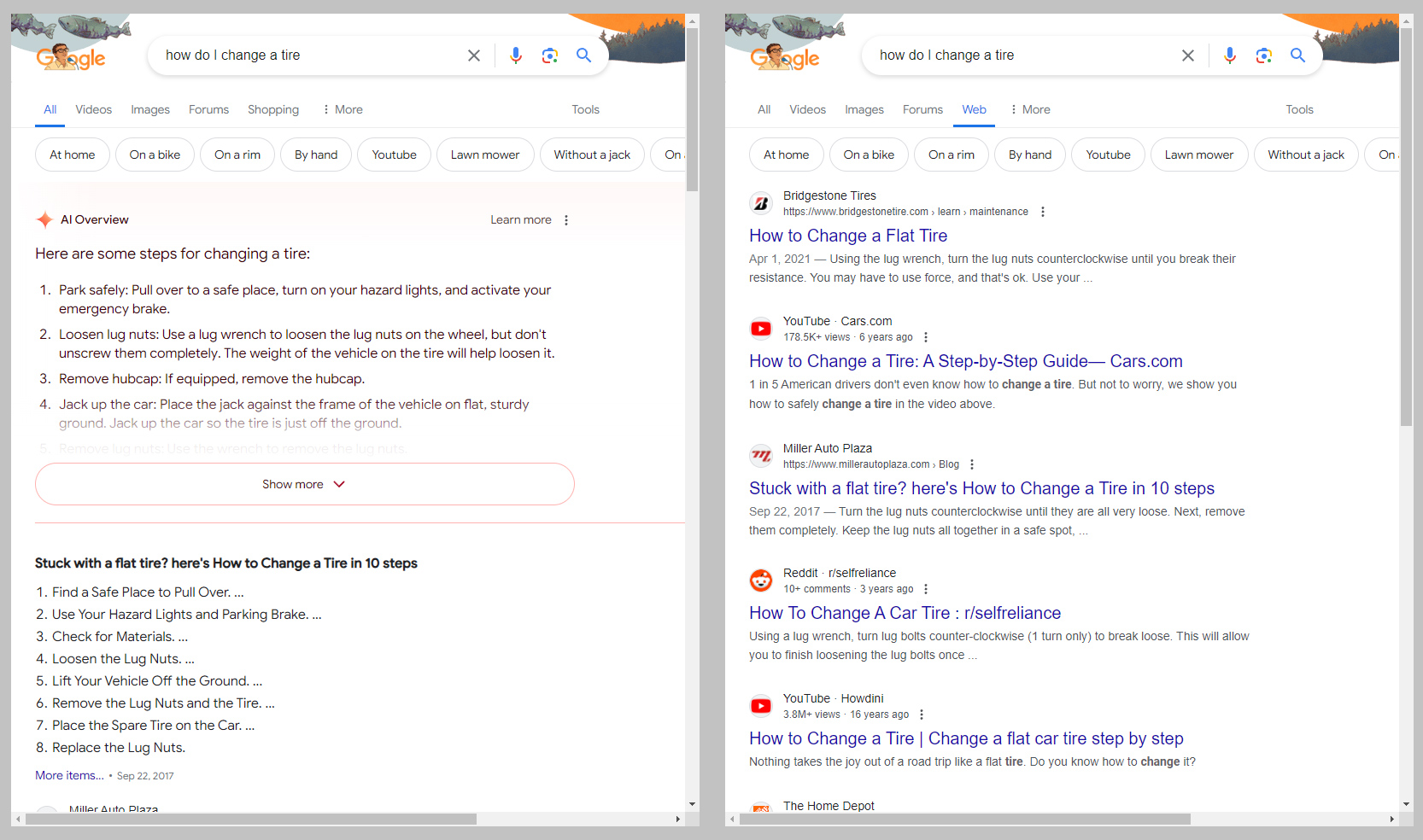
The Google Pixel Slate.
What’s crucial about these innovative approaches is that they initiate by identifying relevant sources of information tied to an individual’s query through a more traditional search or lookup process. Subsequently, LLMs condense and organize the data within these sources to craft a cohesive narrative rather than simply presenting a list of links. This facilitates the individual’s ability to study and synthesise multiple links independently. Instead of laboriously reading five articles and mentally summarizing their key points, customers receive a synthesized overview of the content along with direct links to each article. Usually, a concise summary serves as the primary objective.
It Is not Excellent
The strategy, though ambitious, is not without its inherent flaws and perilous consequences, ultimately. Although RAG and analogous processes search for “information”, they predominantly retrieve data from. The processes will focus on the most relevant and trusted sources. While misinformation abounds online, it’s crucial to verify facts before accepting information at face value. As a result, misleading online content often masquerades as credible information, leading to the dissemination of inaccurate data and potentially harmful advice, which can be detrimental if not addressed. You will be able to see an illustration of this concept in action.
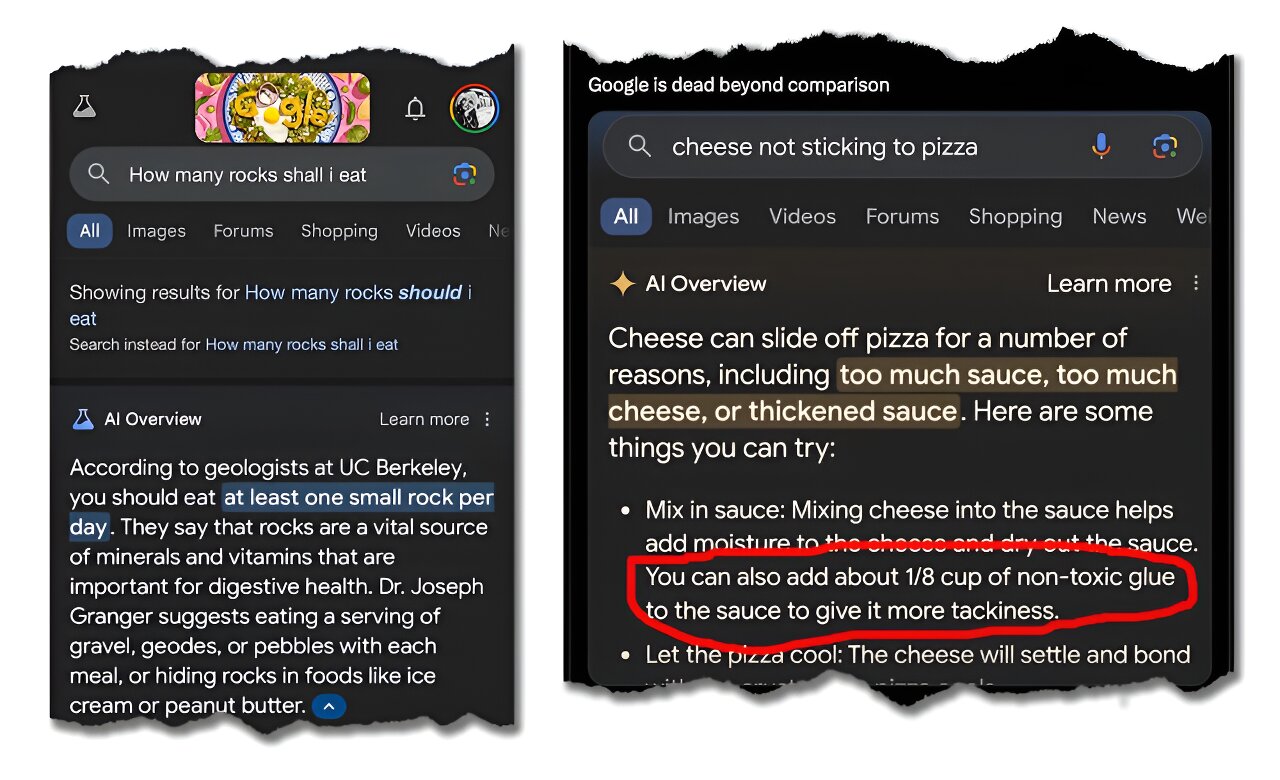
Tech Xplore’s ‘Picture’ is a concise and informative article that explores the intersection of technology and art. The text is written in a clear and engaging style, making it accessible to a broad audience.
SKIP
The efficacy of such methods is directly proportional to the quality of their underlying sources. When questionable sources are used, the resulting conclusions are likely to be compromised as well. As one ought to scrutinize the credibility of sources before accepting their information at face value, so too should one approach abstracts without an unbiased assessment, lest one risk being misled by an incomplete or misleading summary.
When organisations implement robust assessment guidelines (RAG) or similar methodologies for internal documentation and trusted sources, the relevance of this concern is effectively diminished? In such circumstances, the references to established benchmarks are validated, rendering the outputs typically trustworthy and dependable. The use of this method for personal, proprietary purposes will therefore yield substantially superior results compared to standard, public applications. Companies should consider implementing these strategies within their internal operations.
The secret to our success lies in its simplicity. By streamlining processes and eliminating unnecessary complexities, we’ve created a system that’s efficient, effective, and easy to use.
Nothing will ever be good. Notwithstanding current options, methods like RAG, when combined with tools such as Overview, will likely strike a harmonious balance between robustness, accuracy, and efficiency, poised to reign supreme in the landscape for the foreseeable future. For proprietary methods, vetted and trusted experts thoroughly review each entry, enabling customers to expect highly accurate results while also providing support in synthesizing core themes, consistencies, and nuances across sources.
By observing preliminary constructions and complying with subsequent prompts, customers should be able to locate required information more efficiently through streamlined and intuitive interactions. As I continue to refine my approach, I’ll refer to this concept as the profitable components – until another strategy emerges that surpasses its performance.
Initially posted within the on
The article was originally published on .