Introduction
In a society where artificial intelligence seamlessly integrates with humanity, individuals are self-motivated and resourceful, much like students eager to ace an exam without needing a tutor’s guidance. While this concept may evoke memories of robotic epiphanies akin to those in a Transformers movie, its true significance lies in envisioning the future trajectory of artificial intelligence’s learning processes. able to self-training. Seven innovative strategies are poised to revolutionize large language models (LLMs), empowering them to learn autonomously and evolve into even more intelligent, swift, and adaptable entities than their predecessors.
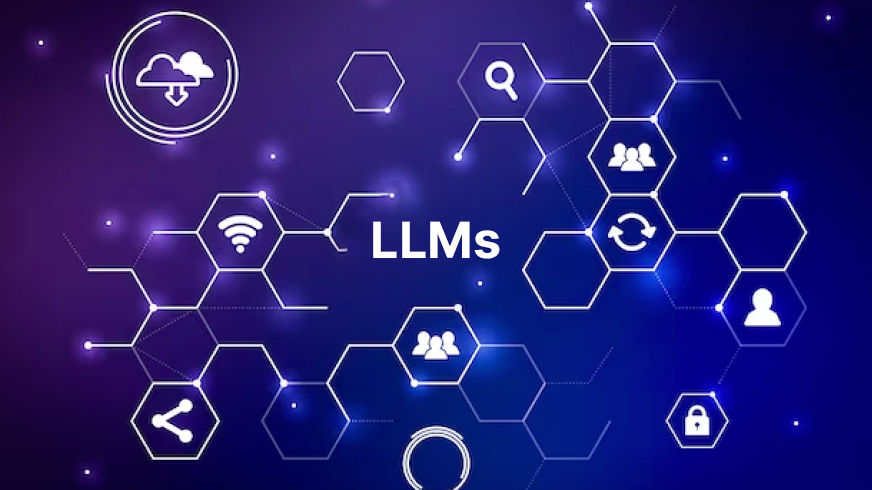
Studying Outcomes
- Explore the concept of autonomous coaching for Large Language Models (LLMs).
- Discover seven distinct approaches leveraged in self-directed training of Large Language Models (LLMs).
- What techniques contribute to the self-improvement of large language models (LLMs)?
- Gather comprehensive understandings on the advantages and obstacles surrounding those approaches.
- Uncover the practical applications and potential uses of highly advanced language models.
- What are the far-reaching consequences of self-supervised large language models on the trajectory of artificial intelligence?
- As society grapples with the emergence of autonomous artificial intelligence (AI) in various domains, including education and coaching, a plethora of moral concerns have begun to surface. Can we truly entrust AI coaches to guide students through life’s most critical decisions?
Can self-supervised learning strategies foster robustness in large language models?
Discovering innovative approaches to coach Large Language Models (LLMs) sans human interaction, let’s delve into 7 groundbreaking methodologies that push the boundaries of AI-driven language learning!
1. Self-Supervised Studying
Serves as the fundamental foundation for autonomous large language model (LLM) coaching. In this innovative approach, fashion models autonomously create their own labels based on input data, thereby eliminating the need for laboriously curated manual datasets. By predicting missing phrases in a sentence, a Large Language Model (LLM) can learn language patterns and contextual relationships without explicit guidance or direction. This methodology enables large language models to train on vast amounts of unstructured data, leading to more generalised and robust models.
A trained AI model can potentially complete the sentence “The cat sat on the” with the accurate prediction “mat.” Through iterative refinement of its predictions, the AI algorithm enhances its comprehension of linguistic subtleties.
2. Unsupervised Studying
Develops novel self-supervised learning techniques that train models on unlabeled data without any human-provided guidance or annotations whatsoever. Large language models autonomously identify patterns, clusterings, and structural formations within the data itself. This technique proves particularly valuable for uncovering hidden patterns in large-scale datasets, allowing Large Language Models (LLMs) to learn complex linguistic representations with greater accuracy and precision.
A large language model might potentially examine an immense dataset of written content and group phrases and phrases according to their semantic equivalence, without any predefined categories or classifications.
3. Reinforcement Studying with Self-Play
Reinforcement learning (RL), in its most fundamental sense, refers to the process by which an agent makes choices within a specific environment, earning rewards or penalties based on its actions. In self-play, Large Language Models (LLMs) can train themselves by competing against various versions or elements of themselves within the context of a video game. As a result of adopting this strategy, notable achievements are feasible across various topic areas, including language evolution, translation, and conversational AI, which can adapt their approaches daily.
A large language model may engage in self-simulation, refining its responses through iterative adjustments to optimize coherency and relevance, ultimately yielding a highly refined capacity for conversational polish.
4. Curriculum Studying
A curriculum studying this manner accurately mirrors the educational program in which a Large Language Model (LLM) becomes proficiently trained on tasks of escalating complexity. By commencing with straightforward tasks and gradually introducing more complex ones, the model builds a solid foundation before addressing advanced challenges. This approach minimizes the need for human oversight by designing an educational pathway that allows the model to learn autonomously and make decisions without human intervention.
A large language model is initially instructed on fundamental grammar and lexical knowledge before advancing to intricate sentence structures and idiomatic phrases.
5. Automated Knowledge Augmentation
Knowledge improvement entails generating innovative coaching methods from existing data, a process that can be streamlined and automated to enable Large Language Models (LLMs) to learn independently without human intervention. Using techniques like rephrased statements, synonym replacement, and reversed sentence structure can produce numerous coaching scenarios, allowing language models to learn actively from limited contexts effectively.
Here is the rewritten text: The canine’s loud bark exemplifies how dogs can effectively communicate their emotions and needs, thus providing an opportunity to explore the complexities of animal behavior in the context of education.
6. Zero-Shot and Few-Shot Studying
Zero-shot and short-shot programs enable language models (LLMs) to leverage their existing capabilities and perform tasks for which they have been specifically trained. With these methods, the need for extensive human-curated training data is significantly reduced. Without prior patterns or training data, the model generates a simulation in a zero-shot setting; conversely, in a brief study, it learns from a limited number of samples.
A highly proficient Large Language Model (LLM), well-versed in English writing conventions, can successfully translate simple Spanish sentences into English without prior exposure to the Spanish language, owing to its innate comprehension of linguistic patterns and structures.
Additionally Learn:
7. Generative Adversarial Networks (GANs)
The generative adversarial network (GAN) consists of two neural networks: a generator and a discriminator. The generator attempts to produce realistic data samples that are indistinguishable from real-world data, while the discriminator tries to differentiate between these generated samples and authentic data? The generator produces synthetic data instances, while the discriminator assesses their authenticity relative to authentic data. As generators evolve over time, they increasingly demonstrate the capacity to generate realistic data, thereby enabling the training of Large Language Models (LLMs). This self-sufficient course of action necessitates minimal human supervision, as the fashion styles are trained on each other.
A Generative Adversarial Network (GAN) could potentially create synthetic textual content so convincing it would be impossible to distinguish from genuine human-written content, thus providing valuable additional training materials for Large Language Models (LLMs).
Conclusion
The direction of acquired LLM coaching constitutes a significant milestone in the advancement of AI-specific disciplines. Without explicit supervision or human feedback, large language models (LLMs) can autonomously refine their performance through self-supervised learning, reinforcement learning via self-play, and generative adversarial networks (GANs), thereby achieving a certain level of self-training. These advancements do not merely improve the functionality of large-scale AI models, but also provide novel guidelines for future development. It is crucial to demonstrate our respect for the ethical implications and ensure that these technological advancements emerge as morally sound as possible.
Are you interested in exploring generative AI and related techniques? Enroll in our program to gain advanced knowledge. This program provides comprehensive coaching and expertise that enables you to acquire the skills necessary to stay ahead of the curve in AI innovations.
Often Requested Questions
A. With scalable advantages, fashion trends can be effectively learned from vast amounts of data without requiring time-intensive and expensive human annotation processes.
A. Self-supervised learning derives labels directly from the data, whereas unsupervised learning forgoes labels altogether, instead focusing on uncovering inherent patterns and structures within the information.
A. In numerous situations, large language models (LLMs) equipped with strategies like self-play or Generative Adversarial Networks (GANs) can achieve significantly better performance through iterative refinement of their knowledge without the influence of human prejudice.
A. Key concerns surround the risk of unforeseen biases, opacity in the research methodology, and the imperative for responsible application to preclude misapplication.
A. By establishing a strong foundation through curriculum-based learning, students are better equipped to tackle complex tasks efficiently and effectively, ultimately leading to enhanced knowledge retention and transferable skills.